Need your investments in AI to do more? Here's how

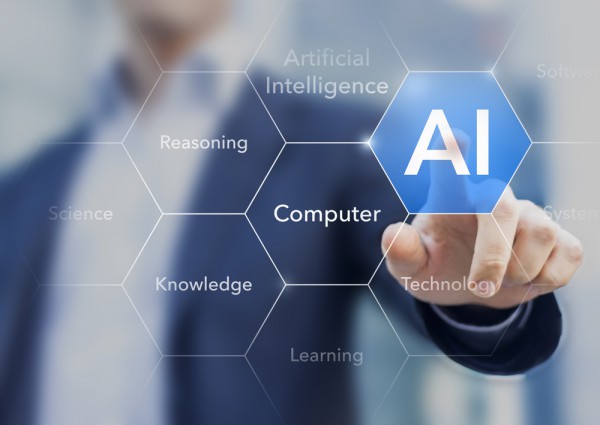
"The impediment to action advances action. What stands in the way becomes the way." -- Famous Stoic and Roman emperor, Marcus Aurelius.
Some organizations have heavily invested in analytics and data scientist teams -- particularly in the manufacturing, retail, and automotive sectors. COVID-19 has disrupted their supply chains, and they find themselves needing to source answers in a day, not in the weeks they would have had to plan through changes last year. They must turn to experts on the front-lines of the business for action, and yet combine this expertise with the power of data scientists’ models to ensure they take optimal measures.
Yet the global downturn that we find ourselves in means that making a business case for further investing in data scientists is cost prohibitive, even if it’s one ideal way to map out possible paths to strategic success. But there are ways forward. And in fact, this is the way… These road bumps, these business challenges, they show enterprises how to do better analytics and conduct better, more resilient, efficient business. It may be a tough lesson to live through, but to bear it well, to turn every obstacle into learning and improvement -- that’s a noble pursuit, as Marcus Aurelius would have said.
Aurelius would have known of one of his Stoic predecessors, Seneca. One of whoms’ sayings has survived to us as: "I judge you unfortunate because you have never lived through misfortune. You have passed through life without an opponent -- no one can ever know what you are capable of, not even you." Businesses need to know what they are capable of.
Rethink priorities for enterprise analytics and be ruthlessly focused on business, not data, KPIs
So how does an enterprise turn obstacles into success, to not only overcome them, but to come out the other side improved? In the world of business intelligence and analytics there are some key tactics that support the business strategy to optimise and compete in a volatile environment. Many of these solve the current problem that companies are spending a lot on data scientists and AI, but not seeing the value.
Organizations need to reorient teams from data key performance indicators (KPIs) to business KPIs to ensure they deliver real business value. As projects go on it’s easy to stray, to not see the word for the trees. Business goals must remain uppermost so that analytics keeps fixed on core goals. KPIs like model accuracy, model complexity, and so on, don’t map onto wider business success. The right KPIs are aligned around improving customer retention, reducing impact on inventory availability from supply chain issues, etc.
Enrich data science by investing in third party data and mashing with the organization's own. It is only by increasing context and making new connections can business teams really be creative and fact-based. Increasing the pool of available business data in whatever field, marketing, customer, supplier, market -- it allows your knowledge and frontline workers to use their expertise and rapidly create, test, and activate new plans.
Companies can't rely on aggregated, averaged data. They need up-to-date information, down to the most granular level, or they will miss critical insights. They can then reorient supply chains, purchasing, inventory, etc., to match shifting demands. Consumer habits along with the whole economy have been affected. Businesses need to consider what new demand patterns may be here to stay in the short, mid, and long terms as the situation evolves. Moreover when the customer asks a question they must get an answer -- or the risk is they walk.
One mantra: Operationalize
AI is only as good as it is consumable by those who use it to make decisions, so rethink how the business operationalizes AI with analytics.
Increase the frequency of the data refresh cycle for the data that feeds these models. Where longer, more stable timelines and supply chains allowed, it may not have been needed to gather fresh data so often. Timelines may have considerably shortened. With higher risk that suppliers or the supply chain may face obstacles it is smart to take decisions to a shorter time horizon and ensure that the overall process is able to iterate and fail/learn fast, the quicker to then succeed.
Automate the process of building and operationalizing machine learning models to allow data specialists to manage the process and iterate even better models. Again, it’s also important to look beyond your own data: To get the most from AI, companies should include external data sources to enrich their own data. More data, with good models, means the business will enhance predictive performance and increase the probability that useful information is in front of decision-makers when they need it.
To do this requires the business turning data science into decision making. Companies are currently racing to operationalize their data models so they can be consumed by regular business users. When analytics is accessible to the frontline staff they enhance their day-to-day decision-making. It speeds up the process of answering customer questions and puts the power of iterating solutions in the hands of those at the 'sharp edge' of activity.
To further increase the speed of business, bring AI-driven analytics to the fore. Data scientists can set the business models and protect the data warehouse whilst the organization empowers users to ask questions via natural language, getting answers at the speed of thought.
To make models accessible to others is only possible with easy to use analytics that can treat a model like any other data source with a natural, consumer-grade front end. Any business that is waiting for custom reports from data experts already knows that the path to success lies in instantly answering ad-hoc data questions on the fly with the same user experience as using search.
We are no longer in the age of users needing to go to coders in IT to use first-wave BI solutions. The power of the cloud offers enterprises the opportunity to give all knowledge workers access to all the data they could wish for -- safely, and with governance. Solutions have advanced such that extensive training is not required any longer. The power of AI within analytics means that a lot of the hygiene and specialist knowledge activities are offloaded and anyone in the organization can simply ask questions to solve problems.
This has freed up data science talent to work on improving access to data, to streamlining governance, and improving the models the business depends on. The opportunity for frontline workers here is massive. They can make their own way, overcome the obstacles that hold them back from high performance, and become masters of their roles.
These are ways that AI spend can do more than just optimise a business data challenge. Every obstacle a business faces in working smarter, faster, and more efficiently shows it where it lacks these skills, where it can make simple improvements to win. The way to success does not come from taking one step, it comes from overcoming every mountain in the path and making a new way.
Photo Credit: NicoElNino/Shutterstock
Spencer Tuttle is Vice President, EMEA -- ThoughtSpot