How AI-as-a-Service is perfectly poised to meet next-era production's ramp-up & capacity challenges

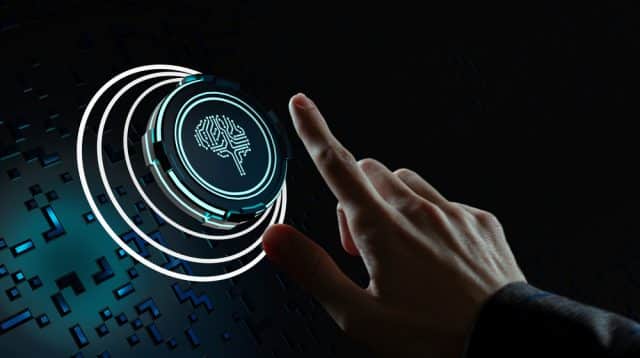
Manufacturing and the science of materials are evolving quickly, so the rate of new products and product variations industrials put into the world is increasing. This evolution also means process parameters, which circumscribe the making of things, are proliferating. Meanwhile, the quality metrics of products and components have become more refined than ever before.
As a by-product, industrial equipment sensors generate an abundance and complexity of data far beyond the reach of statistical process control -- let alone human capacity. Semiconductor engineers, for example, must contend with petabytes of data daily. And they do so from wafers with chip architectures fabricated to accommodate hundreds of millions of transistors per square millimeter.
An example that is emblematic of the production challenges of these times is the seismic, global transition to e-mobility. The International Energy Agency estimates that there could be a staggering 145 million electric vehicles on the road by 2030. This revolution from the internal combustion engine to the electric drive train brings myriad capacity and process implications. These challenges span multiple industrial verticals: from batteries and minerals to automotive and integrated circuits. In sum, greenfield factories must ramp up production as quickly as possible to meet demand spikes and satisfy radically evolving customer requirements.
The turnkey solution to surviving and thriving in the protean landscape of next-era production is, in a phrase, leveraging data for value. Every factory in the world runs according to a control plan. When an unanticipated loss in yield occurs, the control plan is adjusted using expert, time-intensive data analysis. Not only is this process constrained by human experience and time consuming -- it is typically conducted after a production anomaly has already incurred a cost to the plant. It is not fit for competitive advantage in new-era production as an approach.
A data-driven technology like unsupervised Deep Learning, on the other hand, is proven to facilitate proactive, automated optimization. Better yet, the algorithms can be applied to any manufacturing -- irrespective of how new or complex the process is. All that is required is several months of production data to effect significant, continuous, and holistic efficiencies.
How does unsupervised Deep Learning work? Its advanced algorithms do not merely identify or predict production anomalies. This application of AI systematically ingests all upstream and downstream interdependent variables in a production process from multiple data sources. In this way, it can adjust for momentum effects: both between and within steps.
Data scientists work with process and quality teams to exfiltrate sufficient industrial data and use neural networks to build a learned manifold of a production line's process. Next, they model unsupervised Deep Learning algorithms, which parse a mass of historical and live data to discover "Best of Best" (BoB) batches. This AI-driven technique compartmentalizes the manufacturing conditions that will lead to poorer or higher-quality outcomes. Using learned relationships concerning the historical BoB regions, the Deep Learning model delivers prioritized prescriptions to operators.
Depending on what KPIs the industrial has deemed most critical, the AI prescriptions prevent suboptimal runs and allow plants to set new benchmarks consistently. The net result here is cumulative optimization—as the AI's knowledge about the process's inner workings deepens with each iteration of it.
Unsupervised Deep Learning is best deployed with human experts in the loop. AI-as-a-Service is proven to eliminate the risks of adopting AI. It significantly reduces digital transformation costs: guaranteeing industrials expert data delivery, visualization, AI modeling, deployment, and maintenance for ROI. Unburdening manufacturers of the need to source and employ world-class data scientists also frees up time and revenue for innovation and strategic planning, which is increasingly necessary for next-era production.
And it is not just the factories of tomorrow to which AI-as-Service can deliver value. Brownfield factories urgently need to digitize their operations to maximize output and achieve new benchmarks, especially sustainability-related KPIs such as overall equipment effectiveness (OEE).
Ours is a world where a climate emergency, technological acceleration, and global supply chain disruption have rendered company-wide innovation crucial. Data orchestration with machine learning expertise is the only tool potent enough to cut through this industrial moment's efficiency challenges. As the world becomes ever more connected and eco-efficient, this brand of AI-as-a-Service's flexibility means it can also ramp up and shape itself around the requirements of future factories.
Photo Credit: Photon photo/Shutterstock
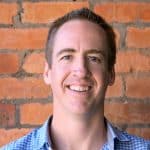
Michael Grant is the inventor of DataProphet PRESCRIBE and currently drives value delivery to customers through consulting and product deployments. He leads DataProphet's innovation, R&D, and technical teams. These teams investigate new and emerging machine learning techniques. Before DataProphet, Michael was Principal Engineer at Transnet leading the Innovation Team and was responsible for many impactful business projects. He has also worked in various roles at CBI-electric: low voltage, including running two of their business units.
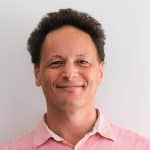
Nicol Ritchie heads up written content creation for DataProphet. He has extensive corporate experience in technical long-form writing across a range of industries -- including financial services, digital advisories, and corporate social responsibility. Nicol holds master’s degrees in both Applied Linguistics and Creative Writing.