How to build AI that fosters unbiased customer interactions

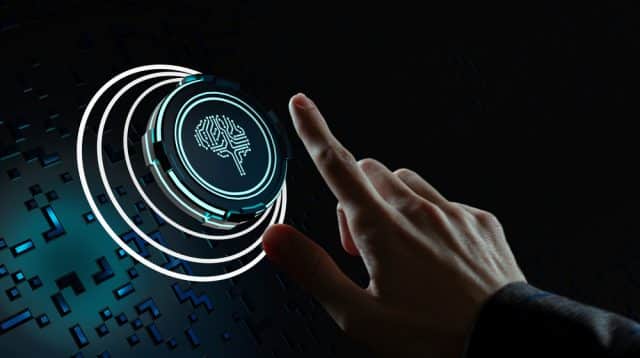
As AI is used more often for several purposes, issues of bias -- often injected unintentionally -- have become more apparent. On a broad level, bias tends to stereotype people. Customers should have useful and positive experiences with AI, but sometimes, these unexpected biases can sour that interaction, leaving customers feeling frustrated or marginalized. As customer service drives customer loyalty, and loyalty drives revenue, this can negatively impact the business as a whole.
Bias in AI can manifest itself in many ways. A sizable percentage of executives polled by Speechmatics found that voice-recognition software struggled to understand some voices.
Meta’s BlenderBot 3, a conversational AI prototype, lasted only a weekend before the AI became, in a word, racist. Similar problems have plagued AI models at least since 2016, when Microsoft’s Tay chatbot started mimicking inappropriate language from Internet users.
How, then, should models be trained to give consistent and helpful responses to customers without creeping bias? At Skit.ai, we have found that building an unbiased AI requires three key components.
1. Train AI for specific interactions rather than for broad use
Narrowing the scope of interaction is key to reducing bias. At Skit, we’ve focused on creating a voicebot with a specific use case: a customer service model that responds only to specific, business-related questions. A bot’s purpose might be collecting feedback, providing updates on claim status, or collections over call. But they are not created to be all-encompassing.
These guardrails ensure that the AI doesn’t begin answering questions it is not qualified to answer. It keeps the entire conversation focused and engaging for customers. Skit.ai’s platform also allows clients -- companies -- to take complete control of the creation and running of the voicebot and its models. This transparency from both sides makes spotting and resolving any bias much easier.
At the end of the day, customers just want to solve their problems quickly and pleasantly. AI trained for specific uses can help companies provide that experience.
2. Consciously clean the data you feed to the AI.
AI models are only as good as the data they are trained on. They tend to go off the rails when fed poor or limited data. While AI needs expansive training data to cover all expected inputs, large data sets increase the likelihood of accidentally training bias into the AI.
Therefore, every AI company must be fastidious about filtering data.
Ayelet Israeli, an associate professor at Harvard Business School, explained in a recent interview that we need to go beyond addressing "incomplete or unrepresentative information" to avoid introducing bias. A good example is avoiding the use of secondary characteristics:
"Suppose that women are more likely to buy red iPhone cases compared to other groups. Now, I decide to exclude gender when I train my algorithm to predict something, in order to prevent any biased outcome. But if I still let the algorithm use the color of the iPhone case, then I'm essentially using a proxy for 'this person is a woman.'"
While many data sets have been polluted by what could be called human error, managing what the AI "eats" can avoid giving it -- or the people it interacts with -- a stomachache.
3. Set up a system of checks and balances.
What constitutes bias in society can change over time. AI models need to account for a dynamic world, and companies need to keep improving their filters and definitions to ensure that they serve their customers fairly and effectively. Because AI is a black box to the end user -- customers -- planning ahead and building transparency into models can spot and correct bias before customers experience it.
Shomron Jacob, engineering manager for AI at Iterate.ai, recently detailed several open-source tools companies can use to help mitigate bias in their AI models. Some of these tools, like Deloitte’s Trustworthy AI framework, help guide companies through potential ethical issues as they develop AI models. Others, like Google’s What-If Tool, help companies visualize potential bias issues.
When you test your AI model, make sure you solicit feedback regarding potential bias. OpenAI caught a number of issues with DALL-E, the art-generating AI, thanks to the diligence of early testers.
These steps -- training for specific use cases, meticulously cleaning data, and setting up a system of checks and balances -- embrace the strengths of both humans and machines. Bias is an inherently subjective entity. It can change depending on your vantage point, so the work continues. By keeping humans proactively involved in shaping the AI, you can prevent bias from forming and improve the model for all customers.
Photo Credit: Photon photo/Shutterstock
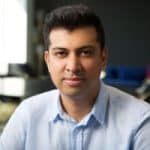
Sourabh Gupta is the CEO and Co-founder of Skit.ai, an augmented voice intelligence platform designed to empower contact centers to manage customer inquiries more efficiently. Founded in 2016, Sourabh’s vision in creating the company is to elevate customer experiences and lay the groundwork for the future of voice interactions. Under his leadership, Skit.ai has raised $27M in Series A and Series B fundraising and has expanded the team to 300+ employees worldwide. The company is operational across India and entered the U.S. market in June 2022. Sourabh has been shortlisted for prestigious leadership awards in India, including Forbes 30 Under 30 Asia 2021 and Entrepreneur India’s Tech 25 Class of 2021.