AI, Machine Learning and Deep Learning in the enterprise: Implications to data storage

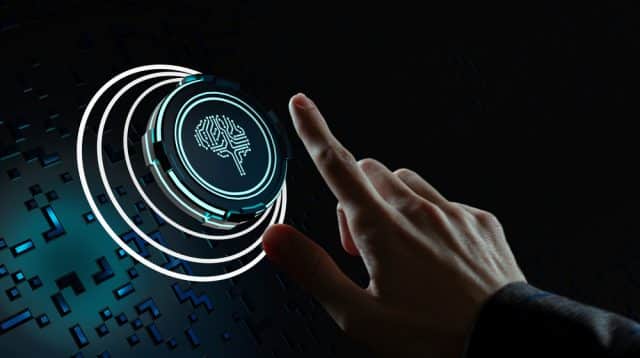
Artificial Intelligence (AI) has been a hot topic for a long time, but its impact on our society and in the enterprise are just beginning to be realized. AI and other forms of machine learning and deep learning will revolutionize business, automating repetitive tasks and accelerating outcomes -- all based on huge sets of data.
Developing deep learning applications generally follows a three-step process of:
- Data preparation, where huge amounts of "raw materials" are turned into usable data
- Model training, where software programs are trained to learn a new capability from the data
- Inference, where (as implied) the program applies this new learning to new data
All of this adds up to massive data growth. Industry analysts project that unstructured data -- files and objects -- will double or even triple in capacity in the next few years. One of the big drivers for this growth is for AI, machine learning, and deep learning use cases.
This "next era" of data creates some distinct challenges for IT infrastructure leaders. First, the datasets are at a scale and volume that is exponentially larger than anything before. Some of our customers developing driver-assistance technology -- essentially a form of machine learning, specifically machine vision -- have generated over an exabyte of data in just a few years. So the scale is massive.
In addition, deep learning applications put huge demands on storage infrastructure performance. Processing these massive unstructured datasets requires extremely low latencies, and critically the performance must be consistent at massive scale. Disk-based storage systems, which are based on serial hard drives, simply cannot meet these requirements. This has led to the growth in all-flash file and object storage, and that growth will accelerate in the next five years as the price of flash decreases, and as new architectures use memory technologies like Non-Volatile Memory Express (NVMe), and Remote Direct Memory Access (RDMA) that enable ultra-low-latency distributed storage architectures. So storage system performance has to improve by orders of magnitude.
Lastly -- the data doesn’t live in one place. It is generated outside of the data center, it is moved somewhere to be processed. This may be in the public cloud, it may be in a data center, or more likely parts of the data pipeline happen in both places. So the movement and management of this data across its lifecycle are a chief consideration. And increasingly, these datasets will be preserved for decades -- not five years or seven years. Specifically, the large datasets used for data preparation, as well as the models themselves, may be stored for decades or longer in case models have to be retrained.
All of these factors have already put pressure on legacy storage architectures. Most of the world’s unstructured data is stored on systems that were designed over 20 years ago. These systems were designed at a time when most of the files created were created by people, not devices, and the notion of trillions of files and objects and exabytes of data to be stored for decades was not on the horizon.
For IT infrastructure decision makers, if your business has digital transformation initiatives, or new business initiatives based around artificial intelligence, machine learning, or deep learning, your data storage infrastructure might be holding your business back. It may be affecting the productivity of the data scientists, content creators, and analysts that rely on this data every day to produce a result. And it is definitely causing you to make unfair trade offs to try and make it work. Take the next steps now to assess what a next-generation architecture should look like, to power the next generation of AI and deep learning applications.
Photo Credit: Photon photo/Shutterstock
Eric Bassier is Senior Director of Products at Quantum.