Why AI Is finally catching up with financial services

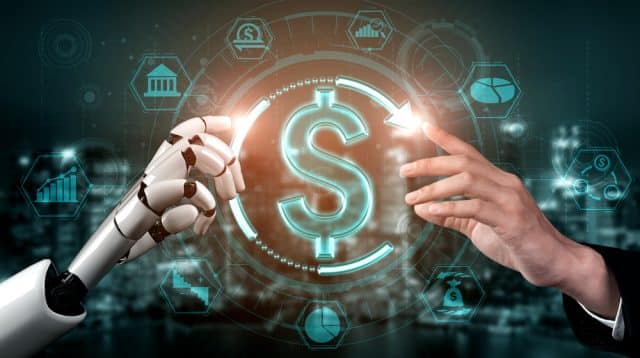
Generative AI has the potential to reshape entire industries and how they operate, and financial services stand out as uniquely poised for AI-driven transformation. McKinsey & Company calls generative AI the next frontier for productivity, estimating that analyzing natural language text -- a core generative AI use case -- accounts for an average of 25 percent of the time people spend in any given enterprise.
The finance industry is a data-driven industry, so it’s no surprise that finance sector firms see big potential in using generative AI to tackle use cases that require assessing enormous amounts of unstructured data, such as company due diligence, know-your-customer (KYC) requirements, sustainability research, and controversy monitoring.
SEE ALSO: Get 'Cybersecurity First Principles: A Reboot of Strategy and Tactics' (worth $19) for FREE
Well-paid finance professionals spend a considerable amount of their time sourcing and examining company reports, regulatory documents, 10Ks and 10Qs, broker research, legal filings, and news coverage. Generative AI promises to liberate them from all of this grunt work so they can focus more time on making more informed and strategic decisions far earlier than they ever could before.
But generative AI has so far fallen far short of the high expectations financial services community has for it.
Any information system must meet three core criteria in order to be enterprise-ready: timely and comprehensive, transparent and trustworthy, and credible and accurate. And until recently, systems built with large language models (LLMs) have failed on all three counts, particularly for financial services use cases.
Because LLMs don’t have access to real-time data, their output is always out of date. This means any recent developments in financial markets, the global economy, or regulations are not accounted for. Even if they are retrained periodically on newer data -- an expensive process -- they still won’t ever be able to meet the near real-time requirements of finance.
LLMs are designed for generalized tasks, but financial service use cases require domain-specific knowledge and access to information from niche datasets, such as sanctions lists or shipping data. This has become a well-known problem with generative AI models used in the banking industry because they’re unable to fully understand intricate financial contexts and nuanced scenarios.
LLMs do not provide sources for the information they generate. This lack of transparency creates a trust gap -- unverified guidance poses risks to investments and reputations -- and a loss of trust among clients and regulators. In compliance use cases, untraceable data sources could violate standards and regulations.
Hallucination
And of course, there’s the infamous "hallucination" problem with LLMs -- coherent and credible-looking responses that are misleading or outright fabricated. In a meticulous field like finance, data accuracy is paramount, and if finance teams are constantly questioning and verifying LLM responses, the benefits are negated while introducing risks of costly errors.
The good news is that with new innovations in AI, these issues have largely been solved.
Pioneered by Meta, retrieval augmented generation (RAG) is a cutting-edge solution designed to handle the kinds of knowledge-intensive tasks that financial services demand. It combines the power of AI-driven information retrieval systems and generative AI to bridge the gap between static knowledge of LLMs and the need for dynamic, context-aware generation in financial services.
Companies like Google, Microsoft, Amazon, IBM and Salesforce are all investing in building enterprise solutions using RAG AI. Let’s explore why.
Unlike LLMs, RAG AI is capable of accessing external knowledge sources, which means its responses can not only be coherent but also based on factual information. This unique combination of retrieval and generation enables RAG-based systems to update its internal knowledge seamlessly, without requiring retraining. This eliminates the need to overhaul the entire system when modifying its knowledge base.
RAG AI’s output is timely because it has real-time access to current information. If an investment banker needs information on a potential regulatory fine, a RAG-based system can quickly retrieve the most up-to-date data on the topic. This ensures their decisions are grounded in real-time data, and decisions can happen earlier because they aren’t wasting time verifying LLM output and supplementing it with the latest information.
RAG-based systems are comprehensive because they can pull from datasets specific to financial services, such as earnings transcripts, sanctions lists, product releases, partnerships, supply chain information, and shipping data. This capability can lead to significant outperformance in investment decision-making while enhancing risk management and helping teams save time in due diligence and KYC efforts.
RAG AI is notable and unique for its transparency and trustworthiness. RAG’s ability to provide sources is incredibly valuable in regard to regulatory compliance and accountability. The ability to back up output with data sources goes beyond just improving accuracy. RAG provides clarity on which sources or datasets the model uses, building trust among users. In financial services, understanding the basis of an AI’s recommendation is essential, whether it’s a market report, financial study, or historical data.
Finally, the ability of RAG-based systems to generate responses solely from the information provided by the retrieval system ensures that the AI remains focused on relevant and factual information, significantly minimizing the likelihood of "hallucinations." For example, a private equity analyst can confidently identify all risks in a deal without needing to meticulously verify each one.
Ideal Financial Services Use Cases for RAG AI
1. Private company pre-screening
The pre-screening process in private equity involves evaluating various aspects of a potential investment, such as industry, products and services, growth opportunities, management team, and risks. Generative AI applications, specifically RAG AI, can improve the efficiency and effectiveness of company pre-screens by summarizing large volumes of data, conducting competitive analysis, identifying risks, and providing personalized insights. RAG AI offers advantages over traditional generative AI by ensuring accuracy, personalization and timeliness through the use of credible information and tailoring the analysis to the specific concerns of the private equity firm.
2. Rapid KYC and background check processes
Know-your-customer and background checks are crucial -- and legally mandated -- in finance to ensure compliance with anti-money laundering laws and to assess creditworthiness. Traditional KYC processes involve collecting and verifying various documents and conducting due diligence to create a risk profile. RAG-based systems have the potential to enhance the accuracy, personalization and timeliness of KYC and background checks by summarizing data, retrieving information from verified sources, and providing real-time risk profiling and alerting.
3. ESG and sustainability research
ESG research is becoming increasingly important in investment decision-making, with a significant number of investors using ESG analysis. Traditional methods of ESG research involve analyzing various sources of data to ensure investments align with ESG criteria -- an extremely time-consuming manual effort. RAG-based systems can leverage real-time data monitoring to offer advantages over manual approaches and generative Ai, such as increased credibility and timeliness of information and the ability to detect greenwashing in company ESG statements.
Image credit: BiancoBlue/depositphotos.com
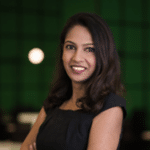
Chandini Jain is the founder and CEO of Auquan, AI innovator transforming the world’s unstructured data into actionable intelligence for financial services customers. Prior to founding Auquan, Jain spent 10 years in global finance, working as a trader at Optiver and Deutsche Bank. She is a recognized expert and speaker in the field of using AI for investment and ESG risk management. Jain holds a master's degree in mechanical engineering/computational science from the University of Illinois at Urbana-Champaign and a B.Tech from IIT Kanpur.