7 steps for managing data in the AI era

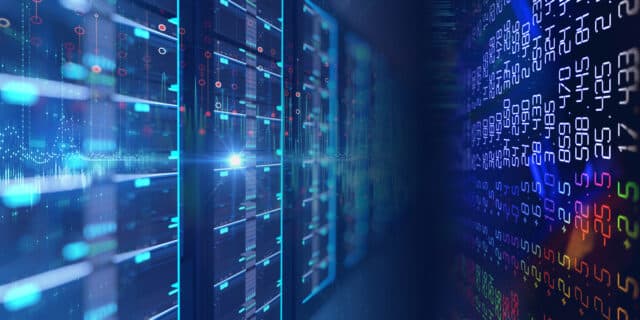
AI will generate 10 percent of all new data in 2025, according to Gartner. This statistic has significant ramifications for business leaders in the digital age.
First, it hints at another substantial development: Overall data generation will skyrocket alongside advanced AI and machine learning (ML) tools. Statista predicts that humans will create, process and consume 180 zettabytes of data in 2025, up nearly 300 percent since 2020. This prediction foreshadows worsening data sprawl, a problem wherein organizations have more data than they can process or understand.
Additionally, mass AI data generation may negatively impact data quality -- so even if business leaders understand their data, it might not necessarily be correct or usable. We’ve already seen these issues arise anecdotally with generative AI “hallucinations,” which fabricate data points or stats. Now, imagine the risks an organization could face if those hallucinations were reproduced at exponential scale and across all systems.
In this complex data landscape, it’s essential that leaders conduct an effective data health check. Doing so is the first step to organizing and managing business data sustainably.
What is a data health check?
Simply put, a data health check gauges an organization’s data accuracy, consistency and reliability. It does so by answering questions like:
- Does the master data contain duplicate entries?
- Is the data format consistent across all records (e.g., date formats, numerical formats)?
- Are the relationships between data objects correctly represented (e.g., parent-child relationships)?
- Are there any broken links or references between data objects?
- Is the data structured in a way that supports business processes?
A business’s specific criteria for data health will vary. For example, certain industries require timelier data than others. Thus, these businesses’ data health checks will prioritize hyper-timeliness as defined by a pre-set “expiration period” (e.g., “data older than three months = expired”).
Data health checks are critical to AI strategy -- and financial wellness. Gartner estimates that poor data quality costs organizations an average of $12.9 million annually. With so much money on the line, leaders should prioritize starting an audit sooner rather than later.
How to audit your data starting today
We’ll break a data audit into seven distinct steps.
1. Check database configuration
An organization’s database comprises all systems managing and storing business data (for example, a master data management (MDM) solution). This repository should include all types of data, from transactional and reference data to metadata.
Start your data health check by verifying that your database is configured correctly. Ensure that buffer pools or caches are sufficiently high to handle workloads efficiently. Additionally, confirm that table and index layouts match the default schema.
2. Verify schema
Validate that all modifications align with business requirements about data integrity and consistency. This process includes checking for additions, deletions or alterations of columns, data types, constraints and indexes. Ensure all changes have been documented and reviewed to comply with your data governance policies.
3. Update table and index statistics
Update database table and index statistics. Doing so will enable the database optimizer to choose the most efficient access plans for SQL queries, improving query performance.
4. Delete old versions
Implement a policy to regularly delete old versions of data to prevent performance degradation. Accumulating obsolete data can lead to increased storage costs, slower query performance and higher maintenance overhead.
5. Ensure high cache hit ratios
Monitor and optimize cache hit ratios to ensure that database queries are served from the cache rather than the disk (when possible). This step reduces latency and improves overall system performance.
6. Leverage AI
AI and ML are powerful tools for automating the data health check process. AI can assist with data cleansing, validation and anomaly detection. It can identify patterns and trends that human eyes may overlook.
7. Measure data health
Once you've completed steps 1-6, your organization's database should be much tidier and healthier. Nonetheless, it's important to verify the success of your data health campaign.
Develop metrics to measure the health of your data, focusing on aspects such as completeness, consistency, accuracy, timeliness and validity. Create dashboards and reports that provide visibility into these metrics, allowing all stakeholders to track data quality over time.
Does your organization’s data pass the stress test?
During a data health check, you’ll identify several weaknesses in your organization’s data strategy. This is normal, especially considering that 52 percent of all business data is “dark,” or uncategorized and unusable. My advice? Use the information you’ve learned to fine-tune your data strategy now instead of waiting for data sprawl to worsen in the years to come.
And, if reviewing the steps of a data audit hasn't helped, it’s likely time to hire a data leader or consult an MDM provider about the steps your organization can take to improve data quality and effectiveness. Doing so today will set your organization up for success down the road.
The bottom line is that AI is advancing and data is expanding. Organizations must fight to catch up. I’m hopeful that advancing data intelligence will enable leaders to keep pace with the digital era -- but only if they enact correct data management policies.
Image credit: monsit/depositphotos.com
Steven Lin is the Product Marketing Manager at Semarchy, responsible for executing the go-to-market strategy for the awarding-winning data company. Prior to joining Semarchy, Steven was a Technology Strategy Consultant at Ernst & Young advising large-scale data initiatives for Global & Fortune 500 firms. He holds a B.S in Marketing & Tech Management and a Masters in Information Systems from Indiana University - Kelley School of Business.