The biggest mistake organizations make when implementing AI chatbots

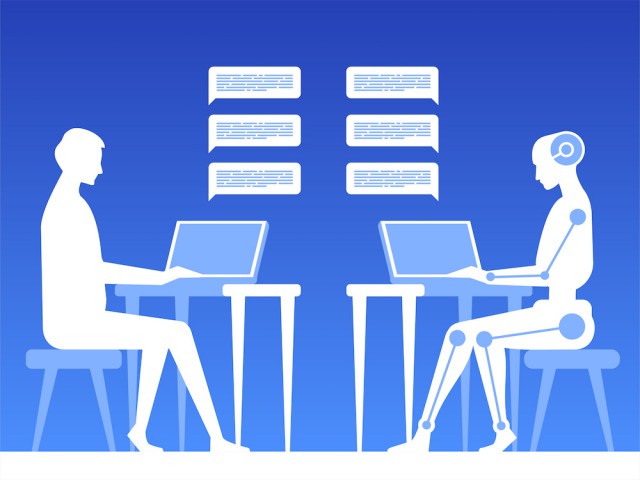
Worldwide spending on chatbots is expected to reach $72 billion by 2028, up from $12 billion in 2023, and many organizations are scrambling to keep pace. As companies race to develop advanced chatbots, some are compromising performance by prioritizing data quantity over quality. Just adding data to a chatbot’s knowledge base without any quality control guardrails will result in outputs that are low-quality, incorrect, or even offensive.
This highlights the critical need for rigorous data hygiene practices to ensure accurate and up-to-date conversational AI software responses.
More data ≠ Better data
A smaller dataset of high-quality, accurate information can be more valuable than a larger dataset filled with errors or irrelevant data. Chatbots that pull from large, low-quality data sets will give poor outputs because larger datasets are full of unimportant noise that makes it harder to detect what is meaningful.
Furthermore, if a dataset contains biases, simply adding more data without trimming the fat can amplify those biases rather than correct them. Similarly, just adding more data without pulling out old data can lead to irrelevant or misleading outputs because old data can quickly become stale. Bad data, even if there is a lot of it, is not enough data to get high-quality outputs.
And there may be a shortage of quality data soon. According to recent research, the supply of publicly available training data for AI-powered LLMs is expected to run out sometime between 2026 and 2032. The data on the internet may seem endless, but it is a finite resource. The rate LLMs are being fed data is far outpacing the rate at which humans are creating it. Soon, some AI giants may be turning to less reliable, AI-generated “synthetic data” to train their chatbots, but many fear that it will lead to degraded performance. The smartest organizations are staying ahead of this curve by mindfully curating their high-quality data through data hygiene best practices.
Tips for squeaky-clean data
High-quality data can only be achieved through adherence to rigorous data management best practices. Here are five key practices for developing effective, reliable chatbots:
- Data quality assurance. Regularly audit your data quality by conducting frequent checks to identify and rectify errors, discrepancies or outdated information. Implement processes to clean and standardize data, removing duplicates, formatting inconsistencies and addressing anything missing. It’s also important to stick to validation rules to ensure data integrity and prevent errors from entering your system.
- Data privacy and security. To protect user data, comply with all relevant data privacy regulations, including the GDPR and CCPA. Employ strong encryption methods to safeguard sensitive information and implement robust access controls to limit data access to authorized users only.
- Data governance. Clearly define data ownership and responsibilities within your organization and establish comprehensive data policies to guide data collection, storage, use and sharing. Create a data retention policy to determine how long data should be stored and when it should be deleted.
- Data labeling. Ensure accurate, consistent labeling of data for training and testing purposes by involving human experts to verify and correct labels when necessary. Regularly review and refine labeling processes to improve data quality.
- Data enrichment. Integrate external data sources to enhance chatbot understanding and responses, and use contextualizing information to enrich data and improve chatbots’ relevance. Keep external data sources up-to-date to maintain accuracy.
By following these best practices, you can ensure that your chatbot has access to high-quality, secure, and relevant data, leading to improved performance and user satisfaction. Chatbot usage will only continue to proliferate, and organizations that cut corners with data now will suffer the consequences in the future.
Photo Credit: Ico Maker/Shutterstock
Todd Fisher is the co-founder and CEO of CallTrackingMetrics. Todd founded the business in 2012 with his wife, Laure, in their basement, and together have grown it into an Inc. 500-rated, top-ranked call management platform serving over 30,000 businesses worldwide. Todd developed the initial software and as the CEO he continues to be the driving technical force of the company. Before CallTrackingMetrics, Todd co-founded SimoSoftware before selling it to RevolutionHealth in 2005.