Making AI agents 'smarter' with connected data

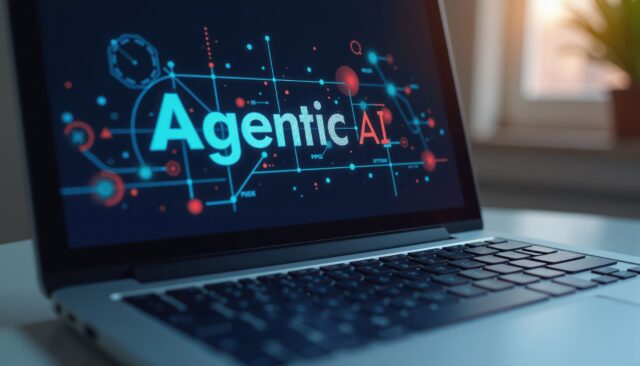
The latest buzzy concept in the world of AI is now transcending its initial hype. Advances in Agentic AI are accelerating the development of autonomous business systems, building upon progress in machine learning. Acting as an independent ‘agent’, the technology can make informed decisions based on the multimodal data and algorithms they’re built on and then ‘learn’ from its experiences.
But even more excitingly, Agentic AI aims to execute tasks independently. It’s this ability to adapt, plan, and execute complex tasks without direct human intervention is what differentiates Agentic AI from its predecessors.
In the supply chain context, for example, AI agents can monitor market trends and historical demand patterns to predict stock levels and plan ways to prevent out-of-stock scenarios, such as automating certain restocking processes. The agents detect when market conditions fluctuate and adjust their behavior accordingly in order to become more effective in pursuing supply chain optimization. It's therefore unsurprising that more than one in four leaders (26 percent) say their organizations are starting to develop strategic roadmaps for using Agentic AI.
However, as great as it sounds to outsource such tasks to Agentic AI, we also need to err on the side of caution. For all its autonomous power, how can the actions and outputs of AI agents be fully trusted? If we rely on Agentic AI to complete sophisticated tasks on its own, how do we ensure its decisions are truly grounded in what’s happening in the real world, or in the enterprise’s view of the world? In the same way our brains use observation and extra inputs to draw conclusions, AI agents need to rely on a lot of external sources and signals to enhance their reasoning capabilities.
This need can be met by solutions and platforms that collect and present data in a way that’s accessible and retrievable. Here’s how:
The high stakes in autonomous decision-making
As discussed, what sets Agentic AI apart from other AI systems is its ability to act autonomously, not just engage in a linear conversation. The complexity of the tasks agents complete typically requires them to refer to multiple, dynamic external sources. As a result, the risk of something going wrong automatically increases. For example, you might trust a chatbot to provide you with an update on the status of a claim or refund, but would you feel as trusting when giving an AI agent your credit card details to book a flight for you?
Away from conversational AI, task-based agents plan and change actions depending on the context they’re given. They delegate subtasks to the various tools available through a process often referred to as “chaining” (the output of one action becomes the input for the next). This means that queries (or tasks) can be broken down into smaller tasks, with each requiring access to data in real-time, processed iteratively to mimic human problem-solving.
The chain effect (in which decisions are made) is informed by the environment that’s being monitored, i.e., the sources of data. As a result, explainable and accurate data retrieval is required at each step of the chain for two reasons. Firstly, users need to know why the AI agent has landed on a particular decision and have visibility of the data source it’s been based on. They need to be able to trust that the action is, in fact, the most effective and efficient. Secondly, they need to be able optimise the process to get the best possible result each time -- analyzing each stage of the output and learning from any dissatisfactory results.
To trust an agent to complete sophisticated tasks based on multiple retrieval steps, the value of the data needed to support the decision-making process multiplies significantly.
The need to make reliable enterprise data available to agents is key. This is why businesses are increasingly recognizing the power of graph database technology, for the broad range of retrieval strategies they offer, in turn multiplying the value of the data.
From confusion to clarity: Harnessing graph power with Agentic AI
As Agentic AI drives decisions from data, the insights underpinning these decisions must be accurate, transparent, and explainable -- benefits that graph databases are uniquely optimized to deliver. Gartner already identifies knowledge graphs as an essential capability for GenAI applications, as GraphRAG (Retrieval Augmented Generation), where the retrieval path includes a knowledge graph, can vastly improve the accuracy of outputs.
The unique structure of knowledge graphs, made up of ‘nodes’ and ‘edges’, is where higher-quality responses can be derived. Nodes represent existing entities in a graph (like a person or place), and edges represent the relationship between those entities -- i.e., how they connect to one another. In this type of structure, the bigger and more complex the data, the more previously hidden insights can be revealed. These characteristics are invaluable to present the data in a way that makes it easier for AI agents to complete tasks in a more reliable and useful way.
What users have been finding with GraphRAG is that not only are the answers more accurate, but they are also richer, more complete, and consequently more useful. For example, an AI agent addressing customer service queries could offer a particular discounted package on broadband based on a complete understanding of the customer, as a result of using GraphRAG to connect disparate information about said customer. How long has the customer been with the company? What services are they currently using? Have they filed complaints before?
To answer these questions, nodes can be created to represent each customer and aspects of their experience with the company (including previous interactions, their service usage, and their location) and edges to show the cheapest or best service for them. A fragmented and dispersed view on the data could lead to the agent offering up a discounted package when it was not due - leading to cost implications for the business. As mentioned by the CEO of Klarna “Feeding an LLM the fractioned, fragmented, and dispersed world of corporate data will result in a very confused LLM”. But the outcome is very different when data is connected in a graph: Positive results have been reported by the likes of LinkedIn’s customer service team, who have reduced median per-issue resolution time by 28.6 percent since implementing GraphRAG.
Get your data ready for the Agentic Times
LLMs powering AI agents are improving rapidly with every iteration, and agentic frameworks are making it increasingly accessible to build sophisticated, multi-step applications. The next critical step is to make your enterprise data as rich, connected, and context-aware as possible so it's fully accessible and usable by these advanced agents. Doing so unlocks the full value of your data, enabling agents that are not only more precise and efficient but also more explainable. This is where the integration of Agentic AI with knowledge graphs becomes a true game-changer. Connected data gives agents the context they need to reason more intelligently, generate smarter outputs, and deliver greater impact.
Image Credit: Twoapril Studio / Dreamstime.com
Jesús Barrasa is AI Field CTO, Neo4j.