The new role of behavioral biometrics in fraud prevention

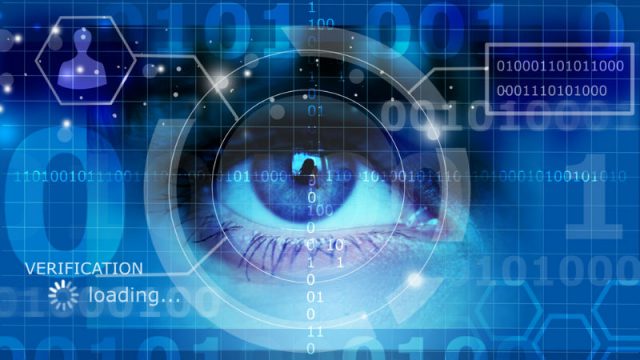
Digital security is undergoing profound transformations catalyzed by the shortcomings of traditional authentication methods. Security strategies often force businesses to choose between robust defenses that frustrate users and seamless experiences that heighten vulnerability. Recently, behavioral biometrics -- using unique users’ digital interaction patterns -- has emerged to offer a compelling resolution to this longstanding challenge by providing continuous, invisible authentication based on unique human behaviors.
Behavioral biometrics can best be understood as digital body language. Like physical mannerisms, such as walking gait or vocal tone, users exhibit distinctive digital behaviors in interacting with their devices -- from typing rhythms and mouse trajectories to touchscreen pressures and even how devices are held. Behavioral biometrics captures and analyses these subtle distinctions to establish a unique digital fingerprint that fraudsters -- and their machinations -- find nearly impossible to replicate. By integrating behavioral biometrics into security and anti-fraud frameworks, organizations can attain a sophisticated balance between strong, proactive fraud postures and the frictionless user experiences that modern consumers expect.
From Static to Dynamic Authentication
Traditional authentication systems have long relied on static credentials -- passwords, security questions and even biometric data like fingerprints -- creating inherent vulnerabilities that sophisticated fraudsters can exploit. The fundamental limitation of these approaches lies in their binary nature; they verify identity at a single point in time rather than continuously throughout the user journey. This one-dimensional verification creates security gaps that can become increasingly problematic as digital transactions grow faster, more complex and prevalent across multiple channels and devices.
For example, during account creation, behavioral biometrics can understand if an account is created by hand or by a bot, if the credit card information is typed or copied and pasted into a form fill, as well as if a mouse moves from pixel A to pixel B in a perfectly straight line; all opportunities for to understand if a human is performing said actions. With bots able to create accounts at scale, being able to discern through strong signals whether an account is bot- or human-generated can make a massive difference in identifying fraud.
The shortcomings of static authentication become particularly evident in the context of account takeover (ATO) attacks, where criminals leverage stolen credentials to hijack legitimate user accounts. These attacks have become alarmingly more sophisticated with the advent of artificial intelligence (AI), with fraudsters employing credential stuffing, phishing and social engineering techniques to bypass traditional security measures, as well as SIM swaps to circumnavigate two-factor authentication (2FA). The consequences extend beyond immediate financial losses to lasting damage to customer trust -- a core asset for business growth, especially in sensitive sectors like financial services.
Traditional defenses struggle against these evolving threats because they can’t effectively distinguish between legitimate users and skilled impostors who possess the correct credentials.
Unlike conventional methods that create friction at specific authentication points, behavioral biometrics works silently in the background, analyzing patterns in how users interact with their devices, including typing speed, pressure applied to touchscreens, hand tremors and navigation habits. The technology effectively transforms authentication from a friction-adding process into an ongoing, invisible action that enhances security without impeding the user experience.
The Technical Foundation of Behavioral Biometric Systems
Behavioral biometric systems combine machine learning (ML) with real-time data collection to create highly accurate user profiles. These systems establish behavioral baselines for individual users during routine interactions and then continuously compare current session behaviors against these established patterns to identify anomalies. Since the technology doesn’t rely on any one behavior but instead examines dozens of parameters simultaneously, it yields multidimensional profiles that are remarkably resistant to manipulation or replication.
Advanced ML modelling allows these systems to distinguish between natural variations in user behavior and suspicious deviations that may indicate fraud. For example, slight changes in typing speed and cursor movements would be recognized as normal variations, while dramatic shifts in navigation patterns or typing rhythms would trigger risk alerts. This nuanced analysis enables security systems to detect potential ATOs even when attackers have valid credentials, identifying the subtle behavioral inconsistencies that reveal impostors.
Device intelligence forms another critical component of behavioral biometric systems, providing contextual data that enhances authentication accuracy. These systems analyze device-specific characteristics alongside behavioral patterns, considering factors like device type, operating system, browser configurations and connection parameters. Integrating geolocation data adds another layer of context, allowing systems to evaluate whether the current location aligns with the user’s typical patterns. This multifaceted approach creates a comprehensive risk assessment that is far more robust than traditional authentication methods.
Implementing Dynamic Friction Through Behavioral Analysis
Dynamic friction represents one of the most significant applications of behavioral biometrics in fraud prevention. Traditional security measures apply uniform verification to all users and transactions, often creating unnecessary friction for legitimate customers while still leaving gaps that sophisticated fraudsters can exploit. A more intelligent approach tailors security measures to each interaction’s risk level, using behavioral analysis to determine whether additional verification is needed. This allows organizations to apply security proportionally -- reserving stringent checks for suspicious activity while enabling trusted users to proceed seamlessly.
Behavioral biometrics plays a central role in enabling this adaptive security strategy. Security systems can instantly recognize deviations from standard patterns by continuously monitoring user behaviors throughout digital interactions and adjusting friction accordingly. For example, a longtime customer logging in from a recognized device and location with expected behavioral patterns would encounter minimal verification requirements. However, if that same user -- or, more likely, an impostor -- suddenly attempts a high-value transaction from an unfamiliar device, exhibits irregular typing behavior, or shows signs of remote access tool (RAT) usage, the system would escalate security measures. These may include multifactor authentication, liveness verification, or even temporary account freezing until further verification is completed.
Social engineering attacks, particularly those involving phone-based scams, further highlight the necessity of dynamic friction. Fraudsters often coerce victims into installing RATs, allowing them to take control of the victim’s device and initiate fraudulent transactions. In conjunction with device intelligence, behavioral biometrics helps detect these attacks by identifying anomalies such as unusual device control patterns, screen-sharing activity or abrupt changes in user interaction behavior. When these red flags are detected, organizations can apply real-time intervention measures, such as blocking transactions, requiring voice verification or prompting the user with contextual security questions.
Implementing dynamic friction through behavioral biometrics delivers tangible business benefits beyond enhanced security. Organizations using these adaptive strategies report significant reductions in false positives -- transactions incorrectly flagged as fraudulent -- which traditionally increase operational costs and damage customer relationships. By accurately distinguishing between normal behavioral variations and genuine fraud risks, businesses can minimize disruptions for real users while swiftly identifying high-risk activities.
Precise, Context-Aware and Unobtrusive
By integrating continuous, real-time device intelligence into everyday digital interactions, behavioral biometrics allows businesses to strengthen fraud defenses and enhance user experiences simultaneously. As AI, ML and complementary technologies evolve, behavioral biometrics will become increasingly precise, context-aware and unobtrusive. Organizations that effectively embrace this shift will position themselves to mitigate sophisticated fraud threats while redefining trust and turning security into a core strategic advantage.
Image source: Shutterstock/Carlos Amarillo
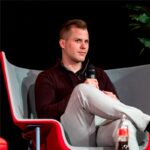
Tamas Kadar is CEO of SEON.