Bringing observability and AI into your legacy modernization plan

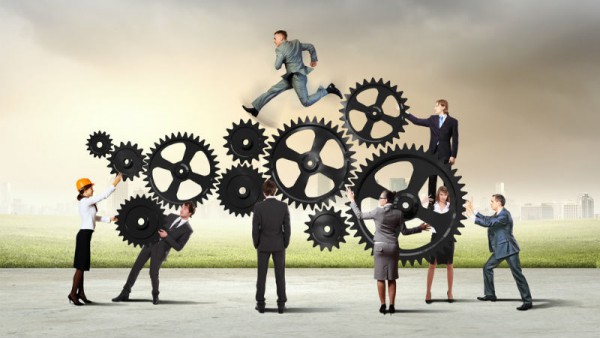
Through evolving legacy modernization, a clear need for automation arose to bring actionable insights to IT and DevOps teams.
Unified monitoring, log management and event management vendors are finding ways to embrace Observability in their tech stacks. And while the overall functionality doesn’t change, these adjustments have led to confusion between IT and DevOps teams. IT Operations and Service Management (ITOSM) professionals are skeptical that Observability is a marketing ploy rather than a tool that actually implements technological change. DevOps professionals, on the other hand, are hesitant of the idea of repurposing legacy tools. So what should vendors do when transitioning standard monitoring technology to use Observability in a meaningful way?
Observability imperative: the road to discovery
The original concept of Observability is based on Control Theory, where a system is considered observable if there’s enough quality data gathered that a person can view how each system reacts to one another, or put another way, you can infer the state of a system from its inputs and outputs. Observability has a different context when referring to DevOps. And in order to understand DevOps teams’ sudden captivation of Observability, we need to explore the origin from an IT perspective.
In 2013, interest in Application Performance Monitoring (APM) began to grow for ITOSM professionals. Because of the overall influence IT has on business success, applications to link IT throughout the business and to customers grew. With each application, event data and end user experience latency metrics were collected, and within minutes, managers had ingestible data to create analyses and detect problems. Meanwhile, markets pressured application developers to improve agility, accelerating the process for improved digital capabilities. From this emerged a new view on how development and production teams interact and, in turn, transformed the entire architecture of applications and infrastructure surrounding it.
The transformation speed of replacing old components with new ones greatly increased, and it gave the opportunity for applications to be formed from smaller, more independent components. Application-level functionality and infrastructure-level functionality also intertwined more with each other. But the most impactful update is how a larger number of discrete, well instrumented services generate far more telemetry than the preceding monoliths..
ITOSM teams were happy with this system, but when the DevOps community took a closer look, the APM technology used made it near impossible to make DevOps applications observable. They needed to keep up with speeds and detail at which software engineers and developers were managing their systems.
AI leads the charge
DevOps teams clearly saw the problem that led to a revolutionary overhaul in technology in order to make Observability possible. Building off of success from monitoring, log management and event management vendors, ingestion rates of data feeds needed to match the speeds of state change rates. Shifting to monitoring systems based on metrics, logs and (in some instances) traces has put teams on the right track to accomplish this goal. Overall, teams must start with more raw, granular data prior to going through any other layers of systems.
There is one caveat that has been widely ignored between DevOps and APM vendors. Even with reports based on metrics, logs and traces, traditional APM technology doesn’t deliver clear insights due to time-space granularity issues. Even the smartest analysts on both DevOps and ITOSM teams struggle with finding meaningful results.
This is why it’s essential to have an AI or ML component tracking your data feed. ML can keep pace with the volume of raw data being processed in a way naive charts and reports can no longer do. In addition, use of Unsupervised ML can help address the challenges of trying to learn baselines in rapidly changing environments.
Two features are essential for Observability tools to enhance your teams: AI and granular data feeds. Having data in its purest form gives AI the ability to quickly find patterns in data feeds. Without both pieces, there is no way of making the systems observable.
The Observability challenge should not be left solely to enterprise DevOps teams to solve. Seemingly overnight, systems with granular, dynamic architecture have become mixed with older systems using coarse data. The DevOps originated systems allows for teams to detect alerts that could stem from traditional systems in microseconds. And while ITOSM teams have found a system that worked for them, it’s imperative that they embrace the space-time scales used by DevOps teams. Any tool not following this initiative simply will not be fit for targeting applications and infrastructure, not just for legacy tools, but new applications as well. It’s time to improve on collaboration between DevOps and ITOSM vendors and come together to simply become Observability vendors.
Image Credit: Sergey Nivens / Shutterstock
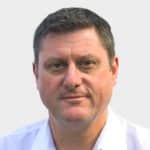
As Moogsoft's chief evangelist, Richard Whitehead brings a keen sense of what is required to build transformational solutions. A former CTO and technology VP, Richard brought new technologies to market and was responsible for strategy, partnerships and product research. Richard served on Splunk’s Technology Advisory Board through their Series A, providing product and market guidance. He served on the advisory boards of RedSeal and Meriton Networks, was a charter member of the TMF NGOSS architecture committee, chaired a DMTF Working Group, and recently co-chaired the ONUG Monitoring & Observability Working Group. Richard holds three patents and is considered dangerous with JavaScript.