How old, incomplete, and inaccurate data can hurt businesses

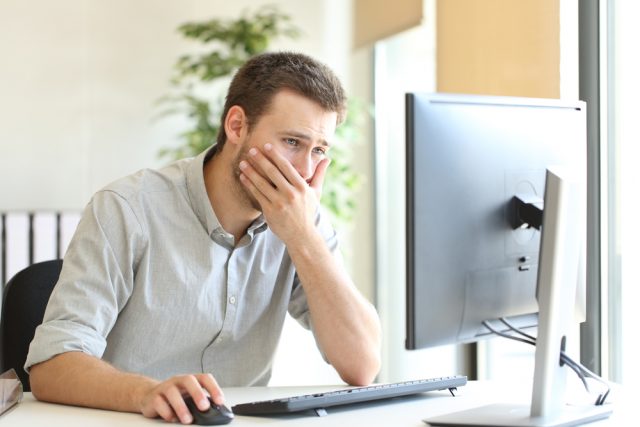
Across the board, from operations to finances to customer satisfaction, the wrong data -- old, incomplete, and inaccurate information -- sets business back. According to MIT, "The cost of bad data is an astonishing 15 percent to 25 percent of revenue for most companies."
The differences between these errant data are important. Also important is understanding that, strictly speaking, there is no "bad data." "Bad" makes data subject to the interpretation of a value judgment. A better, more descriptive term would be "technical debt." This is when engineers begin to indiscriminately start to modify code and introduce new features. Over time, features are layered one on top of another and, if unchecked, teams sacrifice long-term efficiency via a durable, hard-won solution for the sake of easier, short-term approaches that yield a quick solution.
With technical debt, data tends to grow in an application platform or a system. In some ways, it is a measure of success; your platform is only successful if people use it frequently, changing it and customizing it to suit their needs; it gets more powerful as more functionalities are introduced.
But it also gets more cumbersome. The situation is aptly illustrated in something said to me years ago. My boss told me, "for every ten lines of code you put in, you’ve got to delete five. If not, you're just going to be adding to the problem."
The dangers of the wrong data
A successful business favors a bias for informed action. You want to improve overall service, take care of customers, introduce more services, and improve your bottom line. These are achieved by combating technical debt situations, intelligently using the data you need to support the decisions you make. Incomplete and old data work against your productivity.
Enterprise organizations risk everything by making incorrect IT decisions on hiring, on fixing problems, on selling products, on forecasting sales, all the way to spending the wrong money in the wrong place, for the wrong reasons. This is often the cost of inaccurate data, and the risk of technical data, doubling down on a short-term fix when a longer-term solution is required.
Incorrect priorities cause customer dissatisfaction. In the worst case, you lose customers because they feel uncared for, even unwanted. You lose customers, you lose business. The first step IT leaders and managed service providers can take to resolve incomplete and old data is simple: Be proactive. Don't let data become incomplete or old in the first place.
IT must example this challenge holistically: from the collection point of view, the storage point of view, and the output point of view. How you collect information needs to be comprehensive. And -- that bigger issue -- it needs to be accurate. You need processes in place that prevent your data from being incomplete. So, for example, if you collect weather data, you need monitoring in place that lets you collect it on a daily or, if needed, hourly basis. And if data goes missing for a period, a dedicated admin needs to be available to address and correct the situation.
Inaccuracy is a tricky problem. Inaccuracy could result in a misunderstanding of values: Is something a priority-one ticket, or a priority-five ticket? Or does it happen when people update a record in a system? Instead of putting a value in Fahrenheit, for example, they input degrees in Celsius because they are accustomed to European metrics for temperature, distance, and weight. It’s vital to establish strict criteria on setting data that all agree on -- "This will mean Celsius, not Fahrenheit" -- which actively reinforces the prevention of erroneous information from being introduced.
Inaccuracy also comes from missing data, deleted data, duplicated data, and other data that the group doesn’t need. And to make it even more complicated, inaccuracy also arises from the data’s container. So, if you added new fields and didn't populate them or deleted some fields thinking they weren't needed, but they contain historical data, the whole frame of the data is inaccurate.
Best practices
How do we get from point A to point B? How do we get from a system of workflow to a system of analysis and purpose data-wise? Among the best practices against outdated data is snapshotting, having frequently automated or routine review and data comparison to find and correct anomalies. Snapshotting data in this fashion and adding it to a data warehouse, is a worthwhile beginning. But going the extra mile – having a process that’s capable of analyzing a trend, updating records, and realizing their inaccuracies -- is just as important.
Synching technology is also key to identifying and resolving data inconsistencies. Those inconsistencies happen based on the data tracking and extraction methods being used and there are two ways of doing so -- pull and push.
The pull approach is the industry standard. You can pull data from the outside and pull it from a specific place. However, to pull data, you must give someone permission to access the data with personal information like a username, password, and other login details. This approach can expose your critical data to outside elements such as other users and programs, leaving the data vulnerable to deletion, inaccurate changes, and other problems.
The push method, however, offers more secure, accurate updating and monitoring. Credentials don’t need to be given out and outside users and applications don’t have access to your data systems. Instead, you are only pushing out the data you want into the warehouse, allowing for a more efficient and protected approach that ensures data is updated and analyzed consistently.
Inexpensive storage or data warehouses, data lakes, or analytical storage let enterprises adhere to the best practice of snapshotting data to preserve its accuracy. Every competitive enterprise should have a meaningful data strategy that includes these measures to address data inaccuracies effectively.
Photo credit: Antonio Guillem / Shutterstock
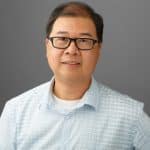
David Loo is the Chief Product Officer for BitTitan, and is responsible for driving the product organization. A 30-year veteran in systems and applications integration, David founded Perspectium in 2013 and was a founding member of ServiceNow's development team and instrumental in creating the foundation for integrating and extending the platform.