Reacting with confidence to a new Trojan horse cyber threat

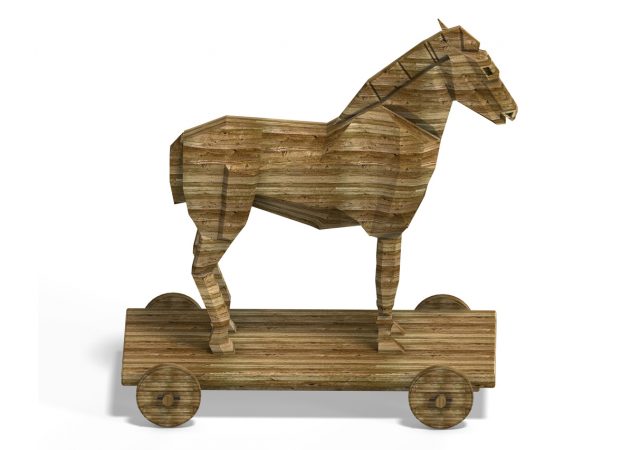
It’s the sophisticated technology that enables the virtual assistant on your kitchen counter, the recommendation engines on your favorite streaming services, and so much more -- and there’s new evidence hackers can use it as a Trojan horse to deliver malware. Because this technology is increasingly embedded not just in your movie nights, but critical industries from healthcare to energy and banking, its newly understood vulnerability is grave news.
But do we tear it out by its roots? Isolate it? No. Artificial intelligence is too important for that -- particularly, ironically enough, in cybersecurity defense. We have no choice but to confront the Trojan horse gambit and block it.
The good news is, I’m confident this is possible. Here’s what I’m talking about.
Artificial intelligence, or AI, has become pervasive -- and increasingly important to our quality of life. A higher-echelon subset of the AI sphere is deep learning, which aims, via mathematics, to mimic human neurological processes -- that is, bring layers of intuition and pattern recognition to virtual experiences. Deep learning runs on deep neural networks, or DNNs, which operate all around us -- think of hierarchical layers of neurons operating on silicon, aspiring to behave like the fleshly neurons between your ears.
The DNN ecosystem has grown phenomenally. Retailers and telecom providers use it to predict customer churn rates. Aviation concerns tap deep learning to solve fleet optimization puzzles. A DNN is behind that fraud alert text from your bank when your credit card is used in some unusual-for-you way. And so on.
Extract deep neural networks from everyday life? We might as well try to pick the nuts out of rocky road ice cream. They’re fully blended.
But into the ice cream parlor walks a skunk. A team of Cornell University researchers has demonstrated a method for transmitting cyberattacks within neural networks undetected. In an August 2021 paper, scientists Zhi Wang, Chaoge Liu, and Xiang Cui declared their method "can deliver malware covertly and evasively through neural network models."
"When neurons are replaced by malware bytes, the structure of the model remains unchanged," they reported. "As the malware is disassembled in the neurons, its characteristics are no longer available, which can evade detection by common anti-virus engines."
Simply put, a DNN contains millions of parameters. A black hat with advanced coding chops could change some less consequential subset to encode malware without degrading the network’s abilities. Not good news, especially as DNN models are widely shared -- and we’re now well familiar with the damage hackers can wreak when they use software service providers as unwitting distribution hubs for trouble. (The SolarWinds and Kaseya attacks are but two recent examples.)
Now, the practice of secreting bad-news attack code within benign files -- image files, text documents, and so on -- has been around a long time. It’s called steganography. The twists worth noting here are twofold: DNNs are pervasive embedded networks, not isolated Word files. And in tests of the Wang-Liu-Cui method, an infected system still worked well enough to escape suspicion.
Worse still, this new attack vector in cyberspace is mostly unacknowledged so far. Hardly anyone is looking out for it. The Cornell paper is only weeks old.
Nevertheless, the answer is not to quarantine deep neural networks, let alone AI itself. They create too much value -- especially in cybersecurity, where ramping up defenders’ AI game is imperative, because our adversaries are setting the pace.
The answer to the DNN Trojan horse challenge is the same answer innovators frame when any emerging, transformative technology, from frozen foods to jet engines, hits a snag. We cope. We innovate some more. We advance the state of the art.
First, note that running this attack strategy on DNNs is something short of child’s play. Hackers must install custom software within the target system to reconstitute the contraband malware on arrival, and the malware in transit can’t degrade the DNN’s performance. No mean feat.
Second, the AI, machine learning, and security communities can -- and must -- take prophylactic steps to make it harder for attackers to succeed. Endpoint solutions can start checking DNN files for signs of tampering. AI researchers can share checksums or other verification data for widely shared DNN models, building a defensive reference database for the common good. Software-as-a-service (SaaS) platforms can adopt and encourage safer best practices -- a trend that should be taken to heart anyway after SolarWinds and Kaseya.
Detecting and limiting Trojan horse attacks via DNN models could be as simple as adopting these modest best practices.
AI is inextricable from our security landscape. "Just as electricity transformed everything 100 years ago," says Andrew Ng, the computer scientist and co-founder of Coursera, "today I actually have a hard time thinking of an industry that I don’t think AI will transform in the next several years." The key to achieving the more secure digital future we deserve is not to panic at new threats. The key is to accept their occasional emergence is inevitable -- and remind ourselves we can muster the brilliance and tenacity to get them under control.
Image Credit: Sadik Gulec / Shutterstock
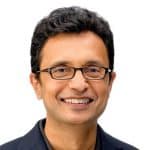
Hitesh Sheth is the president and CEO of Vectra. Previously, he held the position of chief operating officer at Aruba Networks. Hitesh joined Aruba from Juniper Networks, where he was EVP/GM for its switching business and before that, SVP for the Service Layer Technologies group, which included security. Prior to Juniper, Hitesh held a number of senior management positions at Cisco. Before Cisco, he held executive and engineering management positions at Liberate Technologies and Oracle Corporation. Hitesh started his career as a Unix programmer at the Santa Cruz Operation. He holds a BA degree in Computer Science from the University of Texas at Austin.