The dark data challenge

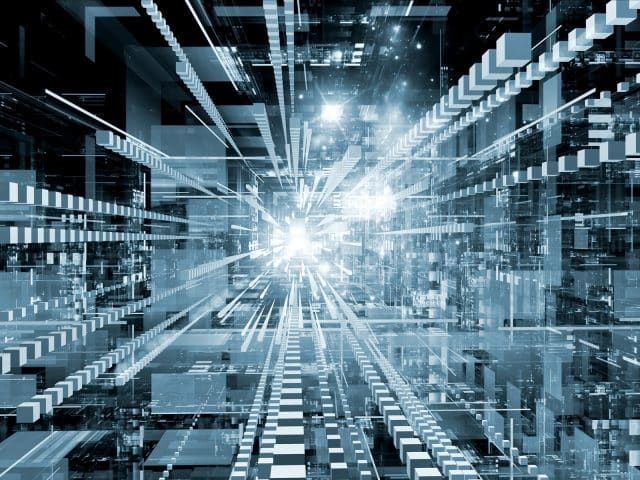
It is estimated that by 2025, the annual global data consumption will amount to 181 zettabytes -- over ten times more than in 2015. Does it mean we will make ten times better-informed business decisions? Most likely not, and the reason is simple: according to different sources, 75 percent or more of the data companies collect lurks in the dark.
'Dark data' is the vast amount of information collected by businesses but never analyzed or used. It can be web and app logs, email correspondence, visitor tracking data, the information generated by IoT devices, etc. Nowadays, every business activity is recorded somehow. Most of this data is unstructured and gathered in different formats. This cornucopia of information has to be processed, stored, secured, and maintained. Instead of increasing ROI, it increases noise, hidden costs, and safety issues since companies are legally responsible for all the collected data, even if they don’t use it.
Some dark data can be tracked, unlocked, grouped, and prepared for analysis with already available AI and ML-powered tools. Even so, employing cognitive automation to identify dark data requires specific skills that are hard to find and substantial analytical resources since the volume of it is often quite extreme.
There’s a low likelihood, however, that anyone will manage to coin a strategy so precise that there’s no redundant, obsolete, or trivial data collected. So is there a way out of the dark data challenge? I won’t delve into defective internal data management practices in this article due to the extensive scope of the topic. Instead, I will quickly go through common mistakes we’ve noticed that companies make when collecting big data from external sources, resulting in poor data quality.
The external data hype
One of the reasons why companies end up gathering redundant data is FOMO and the lack of a clear strategy. Many businesses feel pressured to collect as much data as possible -- they worry that otherwise, they'll be at a disadvantage and won't be able to make informed decisions. Therefore, data gathering practices often miss a clear objective from the start.
The recent proliferation of web scraping tools made massive amounts of public data more accessible to businesses of all sizes. Unfortunately, the sheer volume of dark data implies that companies fail to match the quick rise in data collection capabilities with sufficient ability to clean and analyze it.
In my article about the purpose of data, I argued that data has to provide accurate descriptions of factual business activities and intentionally lead us to actionable improvements. It does nothing by itself until we interpret it, giving it meaning. One of the biggest mistakes is to seek out the data without having a well-reasoned purpose and a list of questions you need to answer. In other words, without a plan for how this data will be utilized. Since data gathering, storage, and processing have associated business costs, collecting redundant information wastes resources.
Web data is noisy
Defining what kind of data the company needs and what purpose it should serve is only the first step toward success. Extracting it brings its own challenges as web data is scattered through different sources and comes in multiple standards and formats. Gathering quality external data requires some programming skills and specific technical experience: web content may be difficult to fetch and analyze, especially at a large scale.
For example, a business might decide to scrape thousands of eCommerce websites for prices, descriptions, and reviews of specific products. Usually, everything’s coming up roses till it appears that the same product is named differently on different sites or there are multiple versions of the same product with only slight functionality differences. Product matching can become quite a hassle for scraping newbies, and the end result might be inconsistent or inaccurate data.
Also, suppose the business doesn’t have enough expertise in data extraction and is trying to gather data from multiple sources indiscriminately. In that case, it can easily fall into the so-called honeypots -- fake and potentially harmful data that security systems feed to unsuspecting crawlers and scrapers.
Another tricky issue the company might run into is that websites are constantly changing and updating their structure. Usually, scraping routines are tailored for specific conditions of the individual sites, and frequent updates tend to disrupt them. Therefore, scrapers require regular maintenance to ensure data integrity.
Often, it’s too costly to develop comprehensive scraping solutions in-house. Oxylabs’ recent research shows that 36 percent of UK financial services companies outsource web scraping activities to solve complex data extraction challenges, and another 27 percent use both third-party and in-house capabilities. Unless you have an experienced in-house team of data scientists and developers, using customized third-party software or outsourcing extraction tasks can be the most cost-efficient way to gather web data.
Open collaboration is key
Since web data is noisy, the company must constantly audit the data it collects to get rid of conflicting, incorrect, or unnecessary information. Auditing helps identify sources that provide the best information for your scraping intentions and allows filtering out sites with too much redundant or garbage data.
If there is still too much data in your databases or it looks inconsistent, it is likely that somewhere along the line, you've collected inaccurate data, or maybe, some of your data is no longer valid. Due to data siloing and poor data integration, companies often lose track or forget what they are collecting, ending up (once again) with redundant or obsolete data.
Finally, even if data collection efforts are successful, the company has to ensure that its team members can easily find that data. If the company doesn’t standardize data collection across all channels and use proper integration tools, employees can run into real problems when trying to locate and analyze it.
Back in 2018, DTC research showed that data professionals were wasting about 30 percent of their weekly working hours because they couldn’t locate, protect, or prepare data. Even more interesting is that another 20 percent of their time passed by building information assets that already existed in their company.
As organizations expand, the possibility that large amounts of data will become compartmentalized in multiple disconnected databases with only basic metadata and limited searchability increases. It means that different departments and teams aren't looking at the same data but rather only have access to a little snippet. Nobody sees the whole picture, making it difficult to make sound and unbiased business decisions.
Data does nothing by itself
It might be that some challenges I’ve mentioned here sound too generic; however, it’s the basics that are most often forgotten or traded off for faster results. Big data is probably the biggest opportunity that lies outside of any business: utilized in the right way, it can identify and solve problems within an organization, provide insight into the customer lifecycle, and inform ways to increase sales. But data is only good if it’s intentional and spurs us to action.
Often, businesses treat having more or having data at all as a necessary good. Fortunately or not, there seems to be data for everything -- customers' interests, website visitors, churn rates, sentiments, demographics, and so much more. With the sheer amount of information available, the most important task before a company embarks on the next data scraping journey is to decide what is valuable for its business and what’s not.
Image credit: agsandrew/ depositphotos
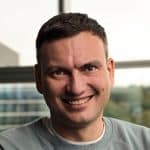
Julius Černiauskas is CEO at Oxylabs.io.