Why AI still needs human intervention [Q&A]

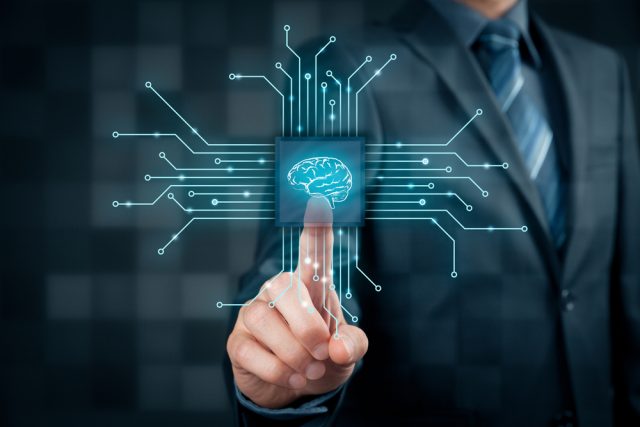
There have been a number of instances lately where the line between artificial intelligence and artificial stupidity has been a pretty thin one. Microsoft's Bing insisting that we're still in 2022, or Google's Bard serving up factual errors, for example.
These problems show that human intervention is still key to getting the best from AI/ML models. We spoke to chief product officer at Tamr, Anthony Deighton, to discuss how organizations can leverage AI/ML while also keeping the key human component of the process.
BN: Why does AI make these silly errors?
AD: There's a perception with AI/machine learning that it's a magical black box. You send data in, and clean data comes out, with little to no transparency as to how it works. This approach makes it easy to resolve large amounts of data quickly and at scale, but it lacks the human feedback needed to improve the models.
BN: What is the role of human feedback in making AI more accurate?
AD: On the other end of the spectrum, we find processes that are 100 percent human-driven. Companies hire tens, even hundreds, of people, often in low-cost areas, and ask them to resolve the data and the entities in the data. Human-driven processes work, but they are labor-intensive and don't scale.
While both of these approaches are options when it comes to mastering data, I believe that there is a middle ground. A place where the machine takes the lead and humans provide guidance and feedback to make the machine -- and the results -- better. This is supervised machine learning, and it’s the data mastering approach that delivers the best outcomes.
BN: How much of an influence are the rules governing the AI in the first place?
AD: The rules governing AI/ML can have a significant influence on the development, deployment and use of these technologies. The rules can affect various aspects, including ethical, legal, social and economic considerations.
From an ethical perspective, rules governing AI/ML can help ensure that these technologies are developed and used in ways that are aligned with human values, such as fairness, accountability, transparency and privacy. Rules can also help prevent the development of AI/ML systems that could cause harm, such as those that are biased, discriminatory or pose risks to human safety.
From a legal perspective, rules can establish the rights and responsibilities of different stakeholders involved in the development and use of AI/ML systems. For example, rules can govern the liability of AI/ML system developers, the ownership of intellectual property and the privacy rights of individuals.
From a social and economic perspective, rules can help ensure that the benefits and risks of AI/ML are distributed fairly and equitably across society. Rules can also help promote innovation and competition in the AI/ML industry by establishing standards, certifications and other measures that encourage responsible development and deployment of these technologies.
In summary, the rules governing AI/ML can have a significant influence on the development, deployment and use of these technologies, as well as their impact on society. It is therefore essential that these rules are carefully designed and implemented to ensure that they promote ethical, legal, social and economic considerations.
BN: Are there some areas where we can trust AI more than others?
AD: Not without supervised machine learning that combines the best of the machine with the best a human has to offer. Machines are very good at resolving data and data entities at scale and with speed. And they don't get tired. This is a benefit, especially as data volumes continue to grow at a rapid pace.
Humans, on the other hand, are very good at providing feedback and ensuring that the machine's results are accurate. And the more feedback they provide, the better the machine becomes. Another benefit of human involvement is trust. When humans participate in the process and have a hand in training the machine, they are more likely to trust the data. And when they trust the data, they are much more likely to use it in analytics and to drive decisions.
Let's take a look at an analogy to illustrate my point: self-driving cars. Today, companies like Tesla are touting the benefits of their self-driving cars. And they believe that the black box model delivers the better outcome. This is probably the wrong ambition.
See, self-driving cars work really well… Until they don't. When they encounter a situation that they've never seen before, they don't know what to do. And they don’t know how to anticipate the outcome. This happened with a Tesla. The car was driving itself, and up ahead was a stopped firetruck. The Tesla didn't stop and ended up crashing into the fire truck. Why didn’t it stop? Because the situation was unknown to the machine, and the algorithm didn't anticipate a crash as the outcome.
One could also argue that fully human-driven cars are not much better. Accidents happen all the time when humans take the wheel.
But human-supervised driving is the best of both worlds. It combines the power of self-driving cars with human oversight to guide the machine when a new or unanticipated situation arises. In the case of our fire truck accident, if the human was guiding the machine, it could have applied the brake and stopped the car before it crashed. Then, moving forward, the machine would recognize that situation and know to apply the brake before it hit the fire truck.
BN: Are we going to see governments attempt to regulate how AI is used?
AD: As AI continues to become a greater part of a data-driven culture and increase its reach and impact, it is inevitable that greater demands will be made to understand these processes and how they work. We're already seeing governments and agencies set up guidelines for the use of AI. This level of transparency should extend not only to audit the AI processes, but also to the quality of data being fed into such systems to ensure it doesn't introduce biases.
BN: What’s the one thing that needs to improve to make AI better?
AD: One thing to add is the idea of 'truth'. The debate so far has been framed as between 'smart' and 'stupid', but I think the actual debate is 'true' vs. 'false' (or 'right' vs. 'wrong'). AI has no concept of 'truth'. It just says that something fits the model. But people are concerned with truth, so having a human guide AI to a better, more 'true' answer is a laudable goal.
Image credit: Jirsak/depositphotos.com