When putting AI to work, remember: It's just a talented intern

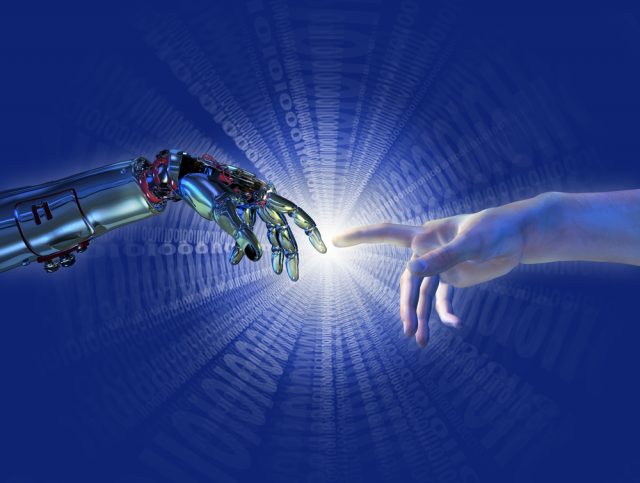
Artificial intelligence (AI) models have been generating a lot of buzz as valuable tools for everything from cutting costs and improving revenues to how they can play an essential role in unified observability.
But for as much value as AI brings to the table, it’s important to remember that AI is the intern on your team. A brilliant intern, for sure -- smart, hard-working and quick as lightning -- but also a little too confident in its opinions, even when it’s completely wrong.
High-profile AI models such as OpenAI’s ChatGPT and Google’s Bard have been known to simply dream up "facts" when they are apparently uncertain about how to proceed. And those instances aren’t all that rare: AI "hallucinations" are a fairly common problem and could contribute to consequences ranging from legal liability and bad medical advice to its use in initiating supply chain cyberattacks.
With recent advancements in Large Language Models (LLMs) like ChatGPT, along with the pace that organizations are integrating AI into their processes, the use of AI for a wide variety of functions is only going to become more common. Observability, a fast-growing field that combines monitoring, visibility and automation to provide a comprehensive assessment of the state of systems, is one example.
But no matter how AI is being applied, it’s important to remember that trust remains a missing element in AI adoption. The models unquestionably add value, but, as with even the most productive intern, you still need to be sure to check its work.
Ensure Trust in AI's Data Sources
The reason the Matrix and Terminator movies were so scary wasn’t that AI had opinions, but that AI made its own decisions. If a computer program with that power makes the wrong decision, the consequences can be dire. On a less dramatic scale, this is the current reality of ChatGPT and its close cousins.
We are not yet at the stage where we're able to take our hands off the wheel and let an AI take control. But we are on the way. So, how can we ensure that we can trust the opinions of an AI?
It starts with being able to trust the sources of data on which an AI model is being trained. Observability teams, for example, need to be taught how to maintain oversight and control of an AI’s training to ensure they get back accurate and trustworthy data analyses and interpretations.
AI hallucinations in this context aren’t caused by incorrect input data. It’s more likely that the AI is incorrectly interpreting that data. AI models are designed to learn as they go -- as opposed to having all of their knowledge preprogrammed -- so the less a system has to read between the lines in assessing the state of a network, the less chance it has of hallucinating and drawing an incorrect conclusion. The more complete its input data is, the more reliable its opinions will be.
Runbooks Offer a Model for Checking AI's Work
In observability, using runbooks for network automation can conquer some of the trust-but-verify issues, combining AI’s speed with in-house experience and knowledge. Runbooks draw on the expertise of people on staff to provide an automated response to issues on the network.
AI can run through a list of scenarios much faster than any human, and can present options for taking action. But we can’t yet trust that AI has all of the right information or has drawn the correct conclusions. That’s because AI’s "thinking" occurs in something of a black box. Explainability -- AI’s ability to describe how it reaches certain conclusions -- is still a work in progress, especially for more advanced models. An AI model’s reasoning can be opaque.
But the logic engine in an automated runbook is a predictable, transparent set of steps. You can see the building blocks and understand how decisions are being made, which allows organizations to trust in their use of automation. We can't trust that AI has all the right information, but we can check its work in the runbooks before pushing forward with whatever solution it recommends.
AI Continues to Learn and Grow
AI is constantly improving, but it is not yet ready to be fully trusted, nor can it be applied generically. Enterprises are building systems from multiple products and need AI for very specific purposes. AI models must be trained for those purposes. Simply giving the job to an all-purpose AI won’t solve your problems. You still need to establish a foundation of full-fidelity telemetry for training and fitting an AI into the model you are running. And then be sure to check its work in a reliable way, such as against a runbook.
The key to the near future is carefully integrating AI to enable organizations to reap its full benefits.
Image credit: AlienCat/depositphotos.com
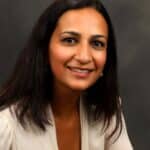
Payal Kindiger, is senior director, product at Riverbed. Payal is responsible for go-to market strategy and execution for Riverbed’s Alluvio portfolio of products. Prior to joining Riverbed Payal has served as a global Marketing leader and business strategist with over 20 years of experience in B2B, startup and Hi-Tech companies, including Resolve Systems and Deloitte. A graduate of UCLA and Kellogg School of Management (Northwestern University), her passion is to serve as a growth catalyst for innovative companies. Areas of focus for Payal include Network Performance Management, Unified Observability, AIOps and more. Payal enjoys sailing, traveling and spending time with her family.