Understanding large language models: What are they and how do they work?

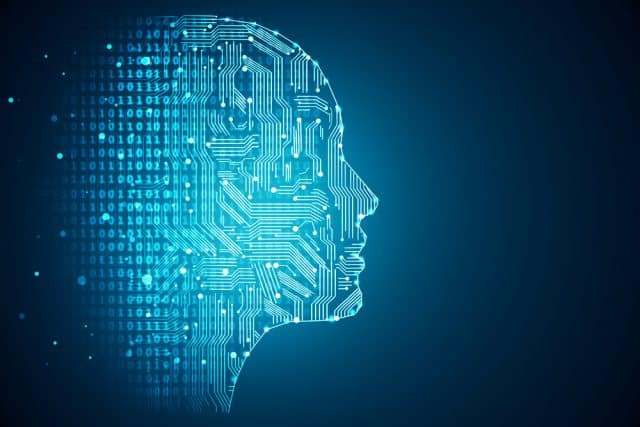
In recent years, large language models (LLMs) have revolutionized the field of natural language processing (NLP) and artificial intelligence (AI). These sophisticated models are used widely in AI solutions, such as OpenAI's ChatGPT, and have been designed to understand and generate human-like text, enabling them to perform various language-based tasks. People are incredibly excited by the potential of this technology which is poised to revolutionize how we live and work. However, to understand the true potential of LLMs, it is crucial that people know how they function.
LLMs, at their core, are neural networks trained on vast amounts of text data. They learn to predict the next word in a sentence by analyzing patterns and relationships within the training data. Through this process, they develop an understanding of grammar, syntax, and even semantic nuances. By leveraging this knowledge, these models can generate coherent and contextually relevant responses when given a prompt or query.
The training process involves exposing the model to a large quantity of data, such as books, articles, and websites. The model learns to identify patterns, extract meaning, and generate text based on the input it receives. As a result, LLMs acquire a remarkable ability to mimic human language, offering the potential to enhance various applications and services.
How to successfully implement Large Language Models
While large language models hold immense promise, implementing them successfully requires careful consideration. Here are some key factors to ensure their effective utilization:
1. Data Quality and Bias Mitigation: High-quality training data is vital for training LLMs. Curating diverse and representative datasets is essential to mitigate biases and prejudices in the source data. Additionally, continuous monitoring and evaluation are necessary to identify and rectify potential biases during model deployment.
2. Fine-Tuning for Specific Tasks: LLMs can be fine-tuned for specific applications to enhance performance. Fine-tuning involves training the model on task-specific data to adapt to a particular domain or problem. This process can help optimise the model's output for specific use cases, such as customer support or content generation.
3. User Feedback and Iterative Improvement: Implementing LLMs successfully requires a feedback loop from users. Collecting feedback, monitoring user interactions, and iteratively improving the model based on this feedback are critical steps to refine its performance over time.
Leveraging Large Language Models to build better virtual agents
One area where LLMs have demonstrated significant potential is in combination with conversational AI. We can achieve even greater interactive and human-like conversational experiences by integrating LLMs into already powerful virtual agents. Here's how LLMs can contribute to building better virtual agents:
- Enhanced Accuracy and Creativity: By integrating LLMs with Natural Language Understanding (NLU), a hybrid system can be created that combines the strengths of both technologies. The NLU provides accurate and reliable responses within a specific business context, while the LLM optimises content generation with its vast general knowledge.
- Empowering Customer Service Agents: Customer service agents can leverage LLMs to enhance their capabilities. For instance, LLMs can scan and condense conversations between customers and virtual agents, providing human agents with a clear and concise summary. They can also help fine-tune the length and tone of responses to match the conversation and generate pre-written messages that include key information from the conversation history.
- Efficient AI Training Process: The text generation capabilities of LLMs can be harnessed to streamline the AI training process. They can generate on-brand virtual agent responses, provide multiple alternatives to a single sentence for use as training data, and rewrite answers to match length and tone. This can save several days of manual work.
- Facilitating Language Expansion: When expanding the virtual agent solution into new markets or languages, LLMs can provide valuable assistance. They can offer translations and provide synonym suggestions when building out a dictionary for language understanding, saving valuable time and enhancing the quality of the virtual agent.
Why a hybrid approach is the way to go
With such a varied list of benefits and abilities, it can be tempting for a business to consider going “all in” on LLMs, but as stressed earlier, responsible and considered adoption is key. The raw processing power of a large language model is exceptional, but it can also be prone to hallucinations and inaccuracies. Adopting a hybrid approach is the way to go if you want to deliver the best experience with customer-facing virtual agents. Connecting LLMs to conversational AI that is pre-trained on company-specific data, with the right guardrails in place, allows for virtual agent scalability and creativity without sacrificing accuracy and data quality.
LLMs have emerged as powerful tools with the potential to revolutionize various aspects of human-computer interaction. By understanding their functioning, implementing them thoughtfully, and leveraging them the right way, we can unlock LLMs to enhance user experiences and create more intelligent and engaging conversational systems. However, it is essential to address biases and connect these models to reliable source data to ensure the information they provide is accurate so that their potential in an enterprise setting doesn’t go unrealized.
Photo credit: Peshkova / Shutterstock
Jerry Haywood is CEO, boost.ai