AI-powered data management: Navigating data complexity in clinical trials

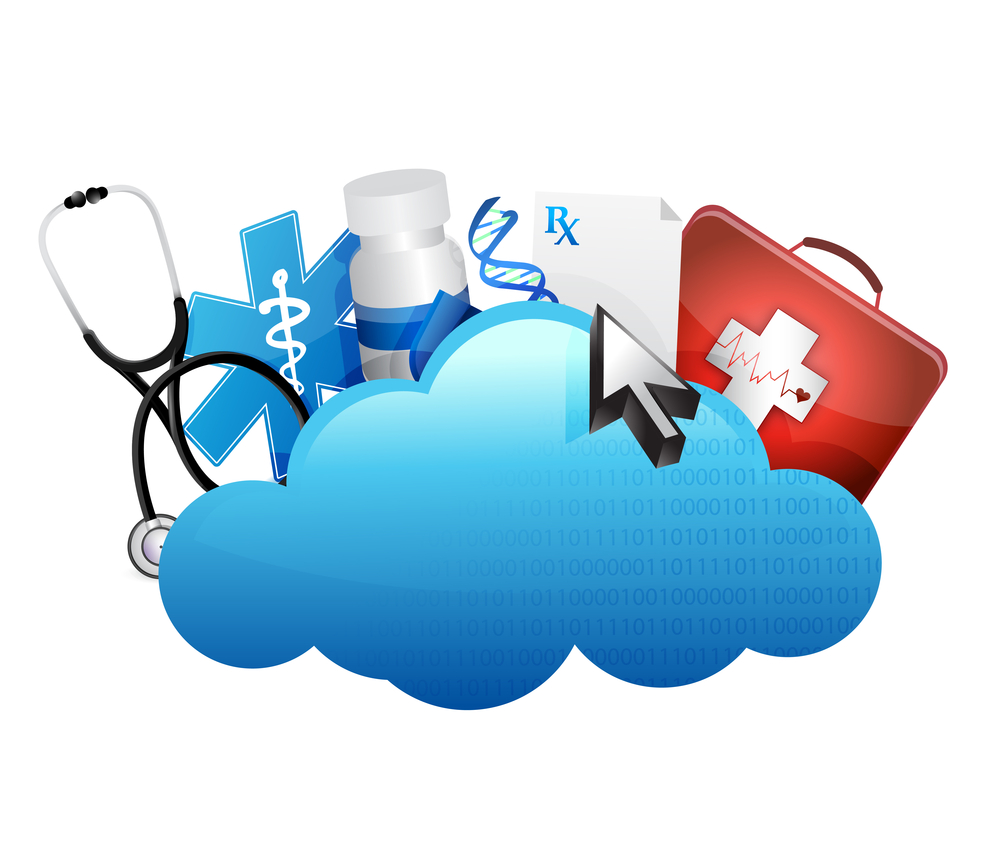
The data flood gates have opened wide for clinical trial research. In fact, the amount of data gathered may be more akin to a tsunami or a monsoon. For decades, researchers struggled with a lack of data available in clinical trials; however, they may have received more than they asked for. Research shows that the biopharmaceutical industry generates up to a trillion gigabytes of data annually and clinical trials, one of the principal contributors to these data points, generate an average of up to 3 million data points per trial. This influx of sources can make it challenging to discern relevant from superfluous information, complicating analysis and delaying critical decision-making.
An increase in decentralization paired with expanded collection methods in clinical trials have increased access to and accumulation of data. Information gathered from remote monitoring devices, electronic health records (EHRs), laboratory tests, surveys and questionnaires and third-party databases, all contribute to the data challenge in clinical trials. In reality, the number of touchpoints across clinical trials, from sponsors to clinical research organizations (CROs) to site staff, combined with the complexity and disparity of data sources leads to challenges in ensuring data quality.
As organizations juggle a multitude of sources and systems, they risk misinformation, mismanagement and extended timelines. Creating more efficient data workflows and streamlining data management can decrease administrative tasks, cut down on timelines, improve staff allocation and enhance patient outcomes.
Providing a Framework for the Convergence of Data Management and AI
To address and streamline the quantity of information across varying sources, organizations are turning to artificial intelligence (AI) to standardize information and collect data insights. The implementation of AI and machine learning (ML) in data management workflows allows organizations to automate and integrate time intensive processes.
By digitizing information, standardizing processes and centralizing data repositories, clinical trial organizers improve the quality, consistency and accuracy of data. Developing a foundational framework for the implementation of AI is paramount as it minimizes security and privacy concerns and creates guidelines to promote the effective use of this technology. The framework should involve:
- Digitizing: Examining the organization’s current business processes and taking steps to identify potential automation opportunities. This will improve productivity and implement an agile workflow. A significant component of this strategy involves augmenting human efforts to achieve strategic goals.
- Standardizing: Implementing a set of standards to create systematic compliance. Establishing standards will also fortify lineage between data collection entities and can be used to speed up processes if protocol amendments are required.
- Centralizing: Storing data in a centralized data repository (CDR) which supplies organizations with a ‘single source of truth’ that can be used to harmonize data. This enhances data-browsing, data-tracking and supports the creation of multiple AI/ML use cases.
It is important to note that improved outcomes with AI/ML are increasingly dependent upon a structured foundation to provide guidelines that enable long term success. Utilizing this technology to improve outcomes is dependent on an established framework to streamline information processing and analysis.
Improving Outcomes Through AI and ML
Streamlining data accumulation and analysis accelerates the drug development process and minimizes risks of data deviation, enhancing staff productivity and improving data collection. The implementation of AI and ML to streamline clinical trial data brings about many benefits, including acceleration of new technology developments. Overall, it increases trial potential to distill large amounts of information as well as easing access to insights through chatbot capabilities. Beyond leveraging these new implementations, clinical trials improve discrepancy identification and author of documentation, identifying and replacing sensitive information in text data. These procedural improvements lead to expedited drug development, reduced risk of data discrepancies, increased staff productivity and elevated data quality.
With AI, organizations can accelerate the acquisition and validation of increasingly complex data from a broad range of sources, and enhance successful trial outcomes via accurate, timely data-driven insights. As with any new technology, organizations must take necessary precautions to ensure regulatory compliance and uphold patient safety and privacy when implementing AI.
By adhering to a foundational framework, clinical trial organizers ensure the safe and effective use of AI in their data management strategy. The established and protected automation of this workflow will ultimately allow for increased development of therapeutics and improved patient outcomes. This new frontier of intelligence provides a breakthrough in data consumption and analysis, which organizations should leverage technology to improve decision-making and accelerate timelines.
Photo Credit: alexmillos/Shutterstock
Tim Riely is VP Clinical Data Analytics at IQVIA.