How to optimize AI at the edge and retain data sovereignty

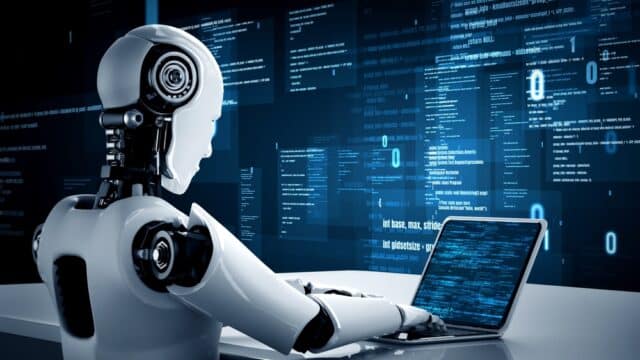
Artificial intelligence (AI) is fundamentally transforming the way businesses operate and the value they can deliver to customers. Industry body techUK cites estimates that the UK’s GDP could be up to 10 percent higher by 2030 thanks to AI adoption. But first there are major cost, efficiency and data governance challenges to solve. This is where edge computing comes into its own -- offering a fast, resilient and cost-effective way to run transformative AI applications. Even better, it can help organizations to meet requirements around sustainability and data sovereignty.
The key will be finding a database platform that can seamlessly support both traditional cloud and edge computing environments in this context.
The race for AI advantage
The UK is punching above its weight globally in the race for AI advantage, with the market forecast to grow to over $1 trillion by 2035. Demand is surging as organizations look to harvest intelligent automation to free-up talent and lower operating costs. More still are turning to AI models to help them analyze historic data and run predictive scenarios -- in order to improve business decision making. And generative AI (GenAI) could help to close skills gaps and boost productivity in areas like customer service and coding. Research reveals that a third (33 percent) of global organizations need to increase productivity each year just to remain competitive.
That’s part of the reason why AI accounts for almost a third of enterprise IT modernization investments in 2023 and 2024, with over half (54 percent) focused on taking advantage of breakout technologies. For IT leaders, it’s becoming an existential challenge. There are real concerns that if they don’t seize this opportunity, their organization will lose staff, fail to get funding and lose its competitive advantage, according to the same research.
Why the edge makes sense
However, AI is not just about pushing the boundaries of data science. Organizations must first get the right infrastructure in place if they want to take advantage in a sustainable way. They must be able to access, share and use data with minimal latency to reap the benefits of GenAI. And they need total control over where data is stored, who has access and how it is used to ensure such models are used safely and in line with data sovereignty requirements. They also need to meet its surging demands for computing power, whilst satisfying ESG requirements and keeping costs under control. Unfortunately, over half (54 percent) of global enterprises still don’t have all the elements in place to deliver an all-encompassing data strategy built for GenAI.
This is where the edge offers several advantages over a strategy reliant solely on traditional cloud computing data centers. Edge computing platforms now have sufficient compute power to support many AI applications. By running calculations at the edge instead of in a centralized cloud data center, organizations can improve speed and reduce the costs and security risks associated with sending data back and forth. It also means that insights are always available at the edge, even if there’s limited or no connectivity to the cloud.
Edge computing supports mobile AI, and use cases in more remote environments such as mines, windfarms and other locations. And because data can be kept at the edge, where it’s captured and used, organizations can reduce the risk of non-compliance with GDPR, or other regulations which may have strict demands around data sovereignty. It’s part of the reason why 65 percent of enterprises believe edge computing will be critical for enabling new AI applications.
Putting the right pieces in place
Factor in energy requirements and cloud egress charges, and the cost of operating AI systems can soon snowball. But by combining centralized cloud with edge computing, organizations can reduce latency, cost and carbon footprint, without compromising on data privacy or sovereignty. Yet first, they need the right data layer, to support edge devices and secure storage of local data.
This is where modern mobile database platforms come into their own. Their architecture will typically feature a database in the cloud, one at the edge in an edge data center, and embedded databases running within apps on devices like smartphones and tablets. All of the above will be connected via data synchronization capabilities, to ensure consistency. Even better, IT managers can set up access control policies at the edge to ensure data is not synced with centralized cloud servers—or else to only allow certain data to leave the edge. That way they can mitigate security and compliance risks.
It all comes down to data
The success of any AI initiative depends on data. That means a great deal of time and effort has to be expended to ensure the best quality data is fed into models. But the hard work doesn’t end there. Where organizations store and process that data also has huge implications. Seek out a modern mobile database platform, and it will enable a hybrid cloud/edge model to unlock true value from AI.
Image credit: BiancoBlue/depositphotos.com
Chris Harris is Vice President, Field Engineering at Couchbase.