Addressing AI challenges for the enterprise [Q&A]

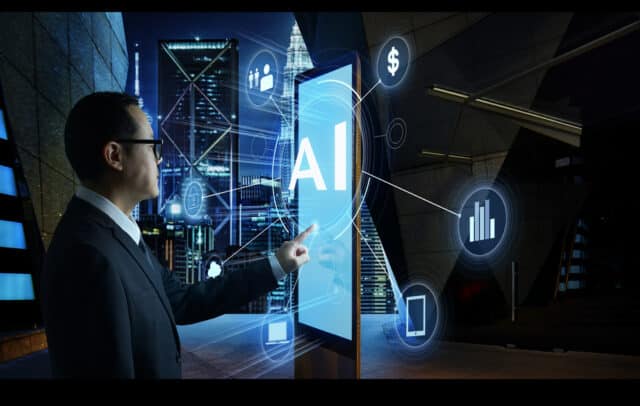
With more and more businesses keen to benefit from the possibilities that AI offers it seems like everyone is jumping on the bandwagon. But this raises a number of implementation and management challenges, especially now as enterprise AI workloads begin to scale.
We spoke to Tzvika Zaiffer, solutions director at Spot by NetApp, to discuss how these challenges can be addressed and the best practices that are emerging to ensure that implementations go smoothly.
BN: How are enterprises balancing the urgency to implement AI with the need for longer-term scalable deployment strategies?
TZ: Its a big challenge that’s getting bigger by the day. Many, if not most, enterprise leaders are up against intense competitive pressure (and in some cases a looming threat of obsolescence) if they don't get their AI initiatives right. Right now, it's a one-way street: according to IDC's March 2024 Future Enterprise Resiliency & Spending Survey, enterprises are determined to sustain their large-scale investments in generative AI and LLMs regardless of the economic landscape.
But as we move past the shiny-new-object era of advanced AI, organizations will increasingly realize that following prudent strategies and best practices -- particularly in operating AI models and applications within cloud environments -- are essential for achieving sustainable scalability. Enterprises with unchecked appetites for costly resources will ultimately end up needing to slow down and resolve these issues, and that’s not a situation many businesses can afford to be in.
BN: How are organizations addressing the infrastructure challenges of AI workloads, especially in terms of GPU utilization and data access across hybrid environments?
TZ: To meet AI goals, enterprise infrastructure must meet the reliability and performance needs of AI workloads. If an enterprise lacks sure-footing in shaping its infrastructure to support AI initiatives, either with on-prem, public cloud, hybrid cloud, or a combination of options, it's unlikely to serve those initiatives well or keep costs in check. Managing the highly expensive GPU processing power quickly becoming essential to large AI models is a key concern, because misuse or poor configurations can produce massive cost overruns and performance shortfalls. DevOps techniques that leverage automated cloud infrastructure optimization offer an ideal approach to controlling costs while delivering effective performance at scale, and should be deployed in tandem with MLOps or other AI/ML automation strategies.
BN: What is the role of FinOps in managing the high costs associated with generative AI projects, particularly in cloud environments?
TZ: Generative AI projects are expensive. Cloud bills -- from the several environments required to build and test AI models and then operate them in production -- add up very, very quickly. Those cloud costs will scale directly with applications and become prohibitive to success unless enterprises prioritize cost efficiency. Sooner or later, they will have to do just that. Assigning FinOps to work alongside AI/ML teams is a crucial practice for achieving that needed efficiency. Specifically, FinOps should introduce real-time cloud cost visibility and analytics, and leverage tactics like automated provisioning and instance-size and pricing optimization based on monitoring data and insights.
BN: What best practices are emerging for fostering collaboration between data scientists, engineers, and operations teams in larger-scale AI initiatives?
TZ: Enterprise leaders should champion collaboration among teams by implementing processes that facilitate internal coordination, and importantly, by holding generative AI teams to the same responsibilities all other teams must adhere to. This sounds simple, but it's become the practice at many enterprises to allow AI teams to ignore budgets and longstanding business processes with the idea that they'll succeed faster with no rules or limits. What actually happens is that everyone from product managers to DevOps to FinOps and other stakeholders have to scramble to fulfill their roles, without the reliable processes they normally count on. That's a recipe for chaos and killing collaboration, and seldom achieves its aims. Maintaining and improving upon clear structures for collaboration around AI is the more effective way to go.
BN: What specific strategies are proving most effective in recruiting and retaining AI talent, given the current shortage of skilled developers?
TZ: Experienced AI developers, data scientists and data engineers are absolutely hot commodities today, and can certainly make a difference in an enterprise's success with generative AI. For that reason, they more or less have their pick of where to take their talents.
A strong strategy for recruiting and retaining AI talent is to appreciate it. Too often, AI talent on AI/ML or big data teams are tasked with maintaining cloud operations related to generative AI, removing them from the work they do best, that they prefer, and that moves the needle on the quality of AI applications. Enterprises that focus on making their cloud operations more efficient, and therefore can allow AI talent to focus on their preferred work, will build a recruitment and retention edge over those that don’t.
BN: How are enterprises leveraging Kubernetes to optimize their AI workloads, and what challenges are they encountering in this process?
TZ: Similar to AI/ML tooling and infrastructure, familiar cloud tools like Kubernetes carry the same impact on AI success. Correct Kubernetes configuration and management can make the difference between an AI application with strong ROI potential, and one where expenses spiral out of control. Optimized cloud and Kubernetes costs and efficiency, which enterprises can achieve with the right automation tools and practices, is clearly an essential component of generative AI success. By getting AI and cloud cost controls right and automating operations whenever possible, enterprises can put themselves in the right position to scale, and to win the race to deploy cost-efficient AI models and applications.
Image credit: jamesteohart/depositphotos.com