How matchmaking platforms use behavioral analytics to predict compatibility

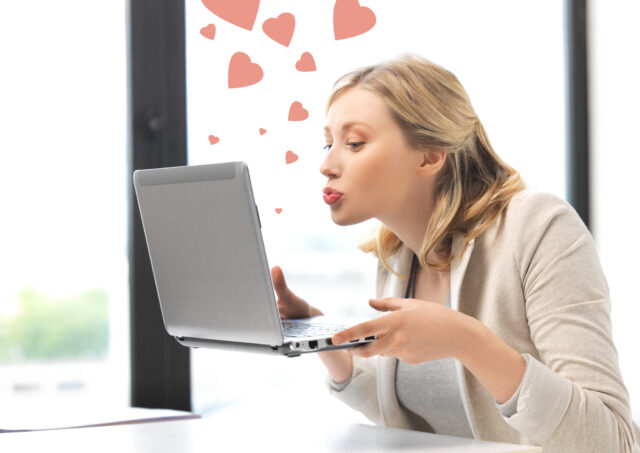
Today, modern matchmaking platforms rely on behavioral analytics to uncover deeper insights about user preferences, communication styles, and actions. These platforms are not just about matching people based on shared hobbies or interests.
For individuals seeking specific connections, such as finding Russian ladies for marriage, matchmaking services should analyze explicit preferences alongside implicit behaviors to predict compatibility more accurately. Unlike social media, which prioritizes engagement metrics like clicks and likes, matchmaking platforms focus on different patterns. Let’s break down how these platforms work and what makes their technology stand out.
At its core, behavioral analytics looks at the small but telling actions users take while using a platform. Matchmaking services use this information to create detailed user profiles that go beyond the basic information entered during signup.
Behavioral analytics is built on data -- specifically, data that tracks how people interact with the app and others on it. This includes:
- Interaction history: How often a user swipes, skips, or revisits profiles.
- Messaging patterns: Response times, message length, and tone.
- Engagement depth: Time spent viewing specific profiles or reading bios.
- Search adjustments: How users modify their filters or preferences over time.
- Decision trends: Choices made during key moments, like prioritizing a conversation over browsing.
These data points are often subtle. For example, someone who consistently views profiles with outdoor photos might subconsciously value shared experiences in nature. Platforms should be able to analyze these tendencies to refine recommendations.
Advanced Machine Learning Models
Machine learning enables matchmaking platforms to process massive datasets and identify patterns invisible to the human eye. These algorithms evolve with use, adapting to user feedback to improve over time.
Collaborative Filtering
Collaborative filtering matches users based on shared preferences and behaviors. If two people have liked similar profiles or share overlapping search patterns, the system predicts that they might find each other compatible. It’s a widely used model but becomes more precise when paired with behavioral data, such as time spent engaging with a profile rather than simply liking it.
Content-Based Filtering
This method looks at individual preferences and recommends matches with similar traits. For example, someone who lists “music festivals” as an interest might be matched with others who show interest in live events. Unlike collaborative filtering, this model focuses solely on the user’s data, making it ideal for capturing unique preferences.
Reinforcement Learning
Reinforcement learning continuously improves matchmaking suggestions by analyzing how users respond to previous matches. If someone tends to message people with a certain style of profile description, the algorithm picks up on this and prioritizes similar profiles in the future. This iterative approach makes matches more accurate over time.
Natural Language Processing (NLP)
NLP analyzes text to determine conversational compatibility. For instance, it can assess whether a user prefers humor, directness, or thoughtfulness in messages. This helps refine matches by prioritizing profiles that align with someone’s communication style.
Matchmaking Platforms vs. Social Media Algorithms
Matchmaking platforms and social media both rely on algorithms, but their objectives differ significantly. Social media platforms aim to keep users engaged for as long as possible, often through content recommendations that prioritize attention-grabbing posts. Matchmaking services, on the other hand, focus on quality over quantity.
Feature | Matchmaking Platforms | Social Media Platforms |
Purpose | Build meaningful connections | Maximize engagement |
Data Focus | Preferences, communication styles | Content preferences, trends |
Outcome | Long-term user satisfaction | Short-term interaction |
Algorithm Design | Predictive compatibility | Reactive content promotion |
Building Compatibility Through Data
Behavioral analytics plays a significant role in transforming raw data into actionable recommendations. Matchmaking platforms use a multi-step process to deliver personalized matches.
Step 1: Data Collection
Everything starts with data. Platforms collect explicit inputs, like age and interests, alongside behavioral signals, such as swiping habits or conversation patterns. These subtle actions often reveal more about preferences than users realize.
Step 2: Pattern Identification
Once the data is collected, machine learning models identify recurring patterns. For example, a user who consistently interacts with profiles featuring shared interests in art and music might be flagged for matches with similar profiles.
Step 3: Prioritization of Preferences
Not all preferences carry the same weight. Advanced algorithms assign different levels of importance to traits based on behavioral trends. For example, shared core values might outrank less critical factors like proximity if the platform detects a strong focus on deeper compatibility.
Step 4: Continuous Feedback
User behavior changes over time, and matchmaking systems must adapt. Platforms incorporate feedback from rejected matches, successful conversations, and updated preferences to fine-tune future recommendations.
Behavioral Analytics in Action
Take the case of a hypothetical user, Emma. When Emma first signs up, she provides basic information and selects a few interests, like outdoor activities and photography. As Emma starts swiping and messaging, the platform notices her preferences are more specific than what she initially shared. For instance:
- She spends more time on profiles with detailed bios.
- Her messages indicate a preference for humor and creativity.
- She consistently matches with people who list hiking as a hobby.
Over time, the platform prioritizes matches that reflect these deeper preferences. It starts recommending profiles with engaging bios, shared interests in outdoor activities, and evidence of a creative sense of humor.
Despite its potential, behavioral analytics in matchmaking is not without hurdles. The use of personal data raises privacy concerns, while biases in algorithm training can affect outcomes.
Matchmaking platforms collect highly personal data, from preferences to private conversations. Implementing robust encryption and transparent policies is essential to protect users and maintain trust.
Avoiding Algorithmic Bias
Algorithms are trained on historical data, which may include biases. For example, if past data skews toward certain demographics or preferences, it can inadvertently limit the diversity of matches. Platforms must regularly audit their systems to ensure fairness.
Balancing Automation with Human Input
While automation enhances efficiency, too much reliance on algorithms can strip the process of its human element. Allowing users to manually adjust preferences or refine filters ensures they remain in control.
Realistic limitations
The advancements in behavioral analytics and machine learning have brought matchmaking platforms closer to offering highly accurate compatibility predictions, but there are realistic limitations. Compatibility involves intangible factors, like chemistry, shared experiences, and emotional timing, that no algorithm can yet measure.
Image Credit: Syda Productions / Dreamstime.com