Is AI adoption the next great risk to data resilience?

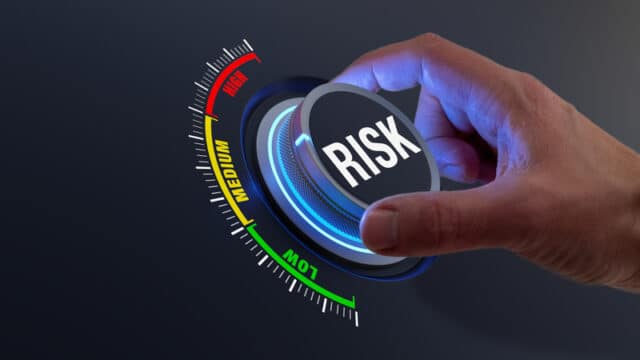
With cyberattacks surging across every sector from critical national infrastructure to commercial businesses, it’s never been more vital for organizations to get control of their digital footprint and restrict access to their most sensitive data. Instead, organizations are being pulled in the opposite direction by AI, which is demanding access to as much data as possible to deliver much-hyped business solutions.
Organizations worldwide are pouring resources into AI innovation, with spending set to hit an astronomical $632 billion by 2028, according to Gartner. Some are even redesigning their organizational structure, introducing new AI-focused roles and even rerouting workflows as they deploy generative AI into day-to-day operations. At the same time, AI organizations are generating unthinkable amounts of investment with OpenAI raising another $40 billion already this year. It’s clear that AI is here to stay, but have organizations lost sight of their data resilience in a bid to keep up with the AI race?
Data resilience still high on the agenda
That’s not to say that data resilience has fallen by the wayside entirely. Organizations have come on leaps and bounds, working to improve their security postures as the threat landscape becomes ever more complex. But these threats are only becoming more sophisticated, and more easily accessible for threat actors. In 2024, Malware-as-a-Service (MaaS) was responsible for 57 percent of all cyber threats to organizations, with less-experienced attackers now able to lean on pre-made malware to carry out attacks.
In response, new cybersecurity regulations have been introduced, especially across the EU, with both NIS2 and DORA coming into effect in the last 12 months. Organizations have had to adapt at pace to meet these new requirements, introducing frequent scenario-based stress testing of incident recovery plans to reach compliance. Just as the attack surfaces and vulnerabilities being targeted each day are changing, regulations are working to ensure organizations are keeping up.
Regulatory bodies have also raised the data resilience stakes when it comes to non-compliance fines, with organizations now liable for fines up to €10 million or 2 percent of their global revenue, whichever is higher. This, combined with the revenue loss and reputational damage that organizations face following a breach, has kept data resilience largely at the front of mind for business leaders, until now, that is.
AI is transforming organizations - but is it for the better?
Organizations have raced to keep up with AI innovation, with many fearing being left behind as their competitors show off their exciting new AI tools. According to McKinsey, 78 percent of organizations are now using AI in at least one business function. However, not all of them are adopting AI securely, with vulnerabilities and exposed AI models all having been identified as common and significant security concerns.
The frenzy that AI has whipped up has led to rash decisions and split-second judgements on how best to adopt it at pace. It’s a somewhat familiar sight, harking back to the mass ‘lift and shift’ to the cloud, where most organizations went all-in without considering the impact on their data management strategies. Except that with AI, the stakes are arguably higher. It doesn’t just concern where your data is stored, but who and what has access to it. Giving unfettered access to your data to AI applications could have far worse consequences if the right safeguards aren’t in place. They can be compromised, either by design or by bad actors.
However, it’s a necessary evil -- AI needs the right data access to turn that data into valuable, usable insights. However, there are data management practices that, when employed correctly, can protect organizational data. Organizations just need to focus, take a few steps back, and implement intentionally before they go too far.
Data management for the AI age
Before organizations go past the point of no return and implement AI models blindly across their data, or even let employees use AI applications on corporate devices, it’s essential that they rethink their data management best practices.
There might be a knee-jerk reaction to turn straight to strategies like ZeroTrust, but for most AI uses, if not all, that’s too many steps in the wrong direction. While it will certainly lock down your data, it will also limit the access of AI to the point where it can’t deliver any valuable output whatsoever.
So if you’re an organization looking to implement AI without compromising your data resilience, how do you go about it?
For a start, before any action is taken, you’ll need to define your data governance policies. Covering everything from your data provenance, how you’ll ensure your data is kept accurate, right up to a set of ethical guidelines as to how you intend to use it. Once this is in place, you can set up a dedicated AI data governance team to embed those guidelines and ensure accountability across your organization.
With all of that in place, you can then move on to the most important part, securing your data for use in AI. At the very least, this should include encrypting sensitive data, although organizations should also consider implementing bespoke access controls and automatic monitoring systems to ensure the security of their data. Role Based Access Controls and Multi-factor authentication would be best placed here alongside audit logs to track access to data and monitor for any inconsistencies or areas for concern.
Although, yes, security measures on their own are better than the alternative; to truly thrive, organizations need comprehensive backup and recovery procedures in place. No cybersecurity system can be 100 percent watertight, and organizations need to ensure that in the event of an incident, they can respond rapidly and resume operations as soon as possible.
But the job isn’t done once these measures are implemented, staying data resilient as you adopt AI is an always-on activity. Take your data quality, for example. You might set up AI with great data, but unless you continue to monitor and update it, the model will become outdated in no time at all. You must enforce your data retention and deletion policies to ensure the dataset is kept up to date and compliant with regulations around data lifecycle management, such as the General Data Protection Regulation (GDPR). And when it comes to new and incoming regulations, all your internal AI policies should be regularly assessed against them, as well as new AI risks, tools, and regulations to stay relevant.
Adopting AI without adopting additional risk
AI has immense transformative potential for businesses, capable of unlocking new functionalities and efficiencies as it develops. Organizations are right to embrace it with open arms, but they mustn’t get swept up in the excitement completely. Like with any other new tool, its impact on the rest of the organization’s security needs to be carefully considered and planned for.
Take the time now to focus and set your organization up properly for AI and keep your data resilience up to scratch or risk undoing all the work you’ve already done.
Image Credit: Nicoelnino / Dreamstime.com
Rick Vanover is Vice President of Product Strategy at Veeam.