Enhancing data security in an AI-driven era

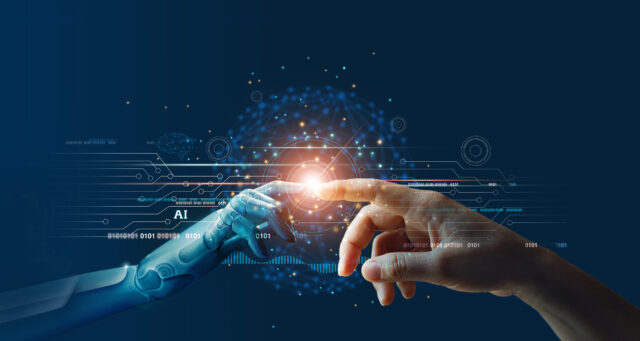
For many years, the IT community has consistently emphasized the inherent value and significance of data. Data is one of the greatest resources within a business, even referred to as an organization’s crown jewels, and as a result, has become a vital part of business’ security strategies.
However, as the global interconnectivity of technology continues to grow, securing data and its integrity has become one of the most complex parts of cybersecurity. The driving factor behind this increasing complexity is the broadening use of generative AI (GenAI) and large language models (LLMs), for which training data has largely become the world’s publicly available data.
Data security and risks from AI are certainly not new. In 2022, Gartner stated that 40 percent of organizations reportedly experienced an AI-related privacy breach. However, the global expanded development of publicly available LLMs has dramatically shifted the data landscape. Training LLMs requires a large quantity of data, and many experts have warned that the world is running out of the quality data required for such training.
Organizations who want to build hyperscale AI and continue to push the boundaries of what is possible, especially in frontier models, will run out of data. While this may be difficult to comprehend, as we are surrounded with more data than combined GDPs of large nations, the fact is that not all data is accessible, usable or purchasable. As a result, there is likely to be an increased reliance on synthetic data. Synthetic data involves creating mock versions of real data, which can be leveraged for training models. However, as it lacks some of the attributes of real data, it is prone to biases and can create accuracy and efficacy issues, which may lead to poorly trained AI models.
AI boom creates complexities for the data supply chain
The data supply chain is akin to a traditional vendor supply chain, whereby organizations identify and manage the data systems they rely on for daily operations. However, in many instances, security measures within the data supply chain do not extend beyond ensuring compliance with basic privacy standards to encompass data reviews or audits. The introduction of AI workloads and the use of multi-vendor AI systems, many of which are trained by and operate on similar data, creates complexities and new requirements for data supply chain security.
Data supply chain risks will become an Achilles heel for organizations, with the threat of interjection of vulnerabilities through the data and machine learning providers that they rely on becoming a very real risk. Poisoning one data set could have huge trickle-down impacts across many different systems.
For example, like modern application development, many organizations building AI models in-house leverage shared resources and existing frameworks and fundamentals. As cybercriminals are always looking for easy or new avenues to cause disruption, open-source libraries and shared code bases are becoming prime targets for data poisoning through methods like prompt or packet injection. Some open-source libraries have already been subject to tampering and while these malicious efforts have had minimal impact, bad actors are likely to increase the scale and sophistication of efforts.
The growing importance of data requires organizations to take a renewed approach to their data supply chain and implement rigorous testing, validation, and verification processes to ensure data security and integrity.
What can businesses do to prepare?
Organizations must be aware of risks and have proper mitigations for their data supply chain in addition to their other vendor security and third-party supply chain security. This issue of data certainty and security is compounded by the varied systems and lack of assurances many businesses have over their data currently. Their data residency, how users can access that data, and technological access to that data either for storage or agentic uses remains a point of concern and often confusion for businesses globally.
Increasing data certainty and security starts with basic data hygiene and management through data classification. Proper data classification ensures sensitive information is adequately protected. Putting systems in place to label and classify business data based on sensitivity and then creating policies to ensure adherence to these labels and classification systems can prioritize security efforts. This will allow organizations to ensure that highly confidential data can be encrypted for extra protection, as required.
Role-based management is equally important. Organizations must uphold strong identity access controls and only provide employees with the data access required to perform their role. Visibility into what internal employees versus what external contractors outside the organization have access permissions to will also help identify areas of the data supply chain that might be exposed and need to be hardened.
While AI technology is often discussed in the context of data risk, it also has a proven track record of protecting business data when applied to cybersecurity strategies and systems. The technology can help better understand business data that is unique to each organization and build patterns of behavior based on that data for individual users and devices. These patterns of behavior create a granular understanding of the business, how its data operates, how its users access that data and where its critical areas of concern are. Leveraging such technologies can help organizations surface and mitigate data risks faster.
AI demands stronger data security controls
While data security has always been an important concern for businesses, in the AI-driven era of globally interconnected technology, it is now paramount for organizations to implement data security strategies and programs. To protect the data supply chains of businesses, as well as continued privacy and data integrity concerns, it is critical that businesses have clear visibility into where their data resides, who can access it, and a robust plan for how to secure it.
Image Credit: Pop Nukoonrat / Dreamstime.com
Hanah Darley is Director, Security & AI Strategy, Field CISO at Darktrace.