AI agents -- how do you get from raw data to meaningful action? [Q&A]

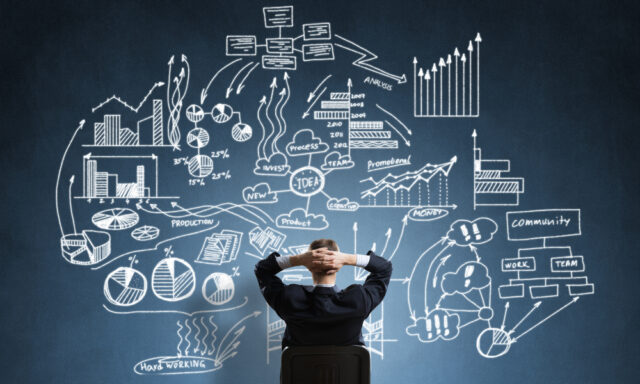
AI agents are here to stay, but there are still some major privacy and data concerns that need to be addressed before the tech goes mainstream.
That's because AI agents are only as good as their data, and right now, that data is fragmented, unsecured, and often unreliable.
We spoke to Yukai Tu, CTO at CARV, to discuss how to give AI agents the verifiability and trustworthiness they need as they take on more sophisticated tasks.
BN: What is an AI agent and are they all the same?
YT: I’m sure plenty of people are wondering the same thing in sorting the hype from the truth. Agents are the next phase of artificial intelligence (AI) -- taking the tech from a passive tool (receiving questions and generating responses) to an active participant (understanding the data and proactively acting on it). Agents are inherently autonomous and intelligent -- making real-time decisions, learning and adapting from previous interactions, and executing independently. This is a big point of difference from generative AI platforms like ChatGPT.
Armed with the correct data and the infrastructure to interpret it, agents can handle various functions. Some are more specialized and others more general, from trading bots making split-second financial decisions to personalized companions assisting with everyday tasks. Regardless of function, all agents share one critical requirement other than computing capability: reliable, high-quality data, and that determines their capability and effectiveness.
BN: What does this mean for managing the data lifecycle?
YT: In these early days of agents, connecting them with the right information and the best infrastructure is mission-critical. People won't trust agents in personal or professional contexts if the information isn't guaranteed on the backend, and agents are only as smart as the quality of information they can access.
Simply put: agents require and users deserve a secure infrastructure that connects data end-to-end. This addresses the current issue that information is fragmented across chains and platforms, and often lacks verification and authentication. The journey from raw data to meaningful action requires several critical steps: first authenticating and verifying the data sources, then processing this information through secure environments, and finally enabling agents to make informed decisions based on this validated data.
This is something we’re tackling at CARV. Through our D.A.T.A Framework, for example, we're creating this infrastructure by handling the complete lifecycle -- from authentication and storage to processing and verification. Users maintain control over their digital footprint while agents securely access the high-quality data they need to function effectively.
BN: How can blockchain technology help ensure AI agents work securely?
YT: We find combining these two emerging technologies is a good way to provide data provenance and consent. Current models like ChatGPT face ongoing questions about copyright -- where did their training data come from and who approved its use? Blockchain provides an elegant solution by creating an immutable, verifiable record of AI data sources and permissions.
By tracking data on the blockchain, we gain unprecedented transparency into how agents access and use information. Every interaction is recorded and verifiable, creating an auditable trail that builds trust. This allows us to implement trustless consensus for verification while maintaining a secure environment for processing sensitive data.
Projects like ours are exploring hybrid blockchain infrastructures that combine different chain benefits. We just launched CARV SVM Chain, for example, a testnet combining Solana's scalability with Ethereum's security, meaning agents can operate with high throughput while maintaining verifiable transactions -- essential for processing large amounts of data quickly and securely.
When paired with technologies like Trusted Execution Environments (TEEs) and zero-knowledge proofs (ZKPs), this creates an environment where agents can operate autonomously while maintaining security and privacy at every step.
BN: What are some real-world applications for this technology?
YT: The sky's the limit for properly secured AI agents that can learn from and evolve with users.
Apart from finance, which we’ve touched upon, some of the applications we're most excited for include gaming and intelligent NPCs that can adapt to create personalized experiences that evolve based on player interactions. Further, the technology also enables privacy-preserved research collaborations in decentralized science (DeSci), potentially accelerating medical breakthroughs. And, even in personal assistance, agents can provide emotionally intelligent support while maintaining privacy.
It's worth mentioning that tracking data provenance also introduces data monetization. Again, by tying information to the blockchain, users can toggle their sharing preferences on and off -- choosing what to share, tracking how it’s used, and receiving compensation when their data contributes to AI development.
This builds a fair ecosystem where both sides benefit: users get compensated for valuable data contributions while AI agents gain access to higher quality, verified information.
BN: When do you think we'll see AI agents becoming mainstream?
YT: This is already proving a big year for agent awareness and adoption. More attention and investment will produce bigger and better results. However, it bears repeating that agents won’t be ready for the mainstream until our sector addresses the very real data privacy, security, and availability questions.
Corporations, for example, won't share proprietary information if the backend is unclear. That’s why we must prepare today’s infrastructure for tomorrow's mass onboarding. The infrastructure must track data from source to use with complete transparency around access and origins.
That's why our sector needs to actively solve the data layer problem -- creating a secure foundation where AI agents can access, learn, and act on verified, structured, and quality data. With the right infrastructure in place, it will only be a matter of time before AI agents transition from niche applications to everyday mainstream tools.
Image credit: Khakimullin/depositphotos.com