Prioritize InfoSec by prioritizing AI data-monitoring

In a survey of IT professionals, 55 percent of respondents reported that their enterprises receive at least 10,000 security alerts every day; of that group, 49 percent receive more than 1,000,000 security alerts each day. And, more to the point, 96 percent of respondents reported that their security teams feel stressed or frustrated over the volume of security alerts that come in.
It's more than mere humans can bear.
The Trouble with Antimalware
Basic tools like antimalware -- as essential as they may be -- are not solving this problem. Antimalware typically works by rote, operating under a negative security model. Negative security models typically identify compromises by matching up what has happened against known attack behavior -- while vindicating everything else. Given ever-evolving attack sophistication, negative security models are not very robust.
To identify compromises that your antimalware software doesn't have a name or entry for, you need to know what a compromised system looks like in general -- i.e., that a compromised system has had something introduced to it that makes it do something that is other than normal.
So, then, what's "normal"?
The Trouble with UEBA
The popular go-to over the past few years for defining "normal" and detecting "abnormal" has been user and entity behavioral analysis (UEBA) -- which compares user login behavior against the norm.
Let's say Alice typically accesses three particular databases, usually from a US-based VPN, and practically never forgets or mistypes her password. One day, Alice accesses six databases from a VPN based in Germany and fails her password three times. UEBA may flag this erratic behavior for further investigation.
Often, this means human investigation, to be clear -- because this is where many use cases of UEBA stop. UEBA solutions know about the three failed logins, but may not be configured to know much of anything about the fourth -- successful -- login.
But that's the most important one to know about. When Alice successfully gained access on her fourth attempt, did she steal anything? Did she delete anything? Did she alter anything? What did Alice do in those databases?
To wit, UEBA is useful, but it is a half-measure that tells only the beginning of the story. UEBA by itself is similar to a bank security camera that records only footage of the people and the ATMs -- while ignoring and editing out footage of the money handlers, vault and safety-deposit boxes.
Let Data Tell the Story
After any breach or break-in, whether it’s your house, car, or corporate database, the first question always asked is, "Was anything taken and if so, what?" If you can’t prevent a breach altogether, at least be able to answer the certain questions that will begin any investigation and incident response program.
Security professionals should want to know:
- Who touched the data,
- Which data they access,
- How was the data accessed,
- When was it accessed, and
- What was done with the data.
- (Bonus points for "why")
So, there’s a need to watch interactions with data.
Alice may access an average of one or two customer accounts an hour. What Alice will not do is access 100,000 customer accounts in a day; that's what a bot, script or simple query does. Compromised systems typically attempt to touch every aspect of every user they can as quickly as they can -- and then transmit that information out.
Even subtler compromises that don't "smash and grab" -- and instead try to act normal -- are telling. Bob, as a salesman, always needs access to customer accounts, and may occasionally need to access data "belonging" to the marketing department -- but he almost certainly never has a need to access HR files. Consequently, if Bob does access an HR file, Bob’s activity may be of interest.
It's pretty easy to distinguish between normal and abnormal behaviors across databases and fileservers if you're looking at them all. But that's the thing: For various reasons, most enterprises aren't looking.
AI for Access Behaviors
Even if they were inclined to look, again, they're drowning in security alerts. Attackers, meanwhile, increasingly have massive botnets and advanced toolkits at their disposal -- sometimes using artificial-intelligence (AI) tools sophisticated enough to evade even AI-driven antimalware. Many attacks fly below the radar by attempting to replicate real-world, real-use behavior. Intruders sometimes lie dormant in a system for months as they quietly poke around. It's impractical, however, to log and analyze every single network action and data interaction in a vacuum. Organizations must monitor and collect data access records, but shift the traditional collect and store mentality, to one of collect, analyze and store.
AI/ML-driven tools that analyze your data access -- and not just your users -- are critical for modern security. They keep security alerts to a minimum, tell a better data narrative, and work well for implementing an effective positive security model -- more acutely defining acceptable and "normal" system and data behavior while cutting attackers far less slack, even if they attempt to normalize their inputs or behavior.
As such, data-targeted AI and ML represent the enterprise's best chance of defending against novel attacks -- but, like other security tools, they aren't as powerful in a vacuum. Combining AI/ML data-monitoring solutions with antimalware, traditional UEBA, and other "lower" security layers allows the security team to more finely hone AI/ML tools without too much inflexibility. All of these layers complement each other -- because there are more threats against enterprise data than we can count.
Image Credit: alphaspirit / Shutterstock
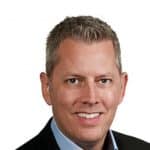