Four steps to securing sensitive data in the cloud

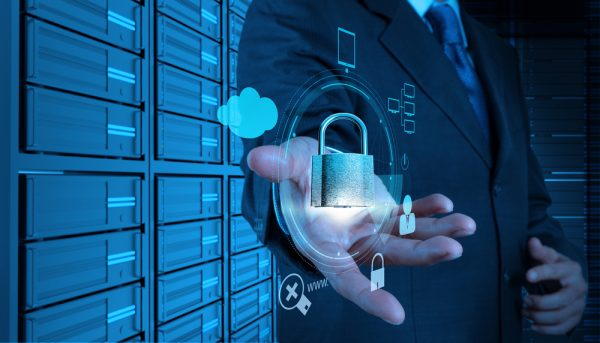
For organizations across the globe, the journey to effectively manage, and extract value from, sensitive data in the cloud isn’t a new one. IT and security professionals have long struggled with challenges to the easy adoption of cloud technologies, and the question of how to ensure that data, including personal identifiable information (PII) or sensitive data, stays safe and compliant with regulatory requirements, without sacrificing data utility, remains a top challenge.
In 2020, the ongoing global pandemic increases this obstacle as remote work drives businesses to move more activity to the cloud quickly. A cloud usage survey released in May found organizations had already surpassed their 2020 cloud spend budget by 23 percent -- highlighting the question of how well data is being protected during this shift to home offices.
In addition to exponential growth of remote environments, the amount of data stored and processed in the cloud is multiplying rapidly. As contact tracing and similar initiatives gain significant attention in the media, concerns about data privacy are increasingly in the headlines around the world. As a result, watchdogs and consumers alike are casting a critical eye on how organizations are collecting, processing, and storing this information, especially in the cloud.
Because the move to the cloud continues to accelerate, it’s important for businesses to understand how they can (and should) ensure that data privacy is built into their processes. Wherever an organization may be on its cloud journey, below are four steps every enterprise should follow to secure and protect sensitive data in cloud environments.
1. Revisit Policies to Understand Who is Responsible for Privacy and Security
A crucial part of securing cloud environments is revisiting cloud policies to ensure ownership is clearly defined between providers and their customers. The three main cloud providers, Amazon Web Services (AWS), Microsoft Azure, and Google Cloud Platform (GCP) all include a "shared responsibility model," which outlines a split of responsibilities between the providers and customers using their services. This creates obligations set out under data protection laws such as GDPR to determine the difference between "data controllers," those who control the procedures and purposes of data usage, and "data processors," those who process the data that the controller gives them. With these definitions in place, businesses can get a better idea of which responsibilities are theirs -- so they can ensure they are taking all the necessary precautions.
2. Establish the Path for a Safe Data Pipeline
When working in the cloud, businesses need to implement and automate a safe data pipeline that provides safe data to those who need it, quickly and easily. Simply put, a data pipeline is a workflow that helps to move data between different sources to achieve business goals. As such, it often contains sensitive identifiable data, such as names and locations that could lead to security issues if that data is compromised on its journey to and from the cloud. For example, a data pipeline could enable sensitive data to be provisioned from a data lake or data warehouse to a data scientist who needs it to perform analytics. A critical part of this is ensuring the data is safe for use from a privacy standpoint.
3. Deploy Dynamic, Comprehensive Privacy Controls
When granting someone access to datasets, the level of associated risk depends on who they are, their role, and how they intend to process or use the data. This risk needs to be balanced with the need to derive accurate insights from the data, and not "overprotect" it to a point at which it loses analytical value. To improve data utility without creating undue risk, a combination of data privacy controls, including different pseudonymization, generalization, and minimization techniques, can be applied to the data before access is granted. These techniques, when applied contextually to the data and its intended use, can remove identifying characteristics, so that no individuals can be identified if an outside security threat occurs or data is leaked accidentally, while maintaining usefulness for analytics, artificial intelligence (AI), and machine learning (ML). This step also provides an extra barrier to protect an organization from human error, the most common factor in data breaches according to the Verizon 2020 Data Breach Report
4. Create Protected Data Domains
Once privacy protections techniques have been applied, it’s important to distribute data with transparency and traceability in mind. One such method of distributing data to use case or user-specific datasets called Protected Data Domains (PDD), a set of managed data releases that evaluates privacy risks and helps mitigate them. These datasets can be digitally watermarked, which creates traceability to the data in the event of a breach and shows which privacy policies were applied to the data, by whom, for what purpose, and when. With this transparency and traceability in place, organizations can tap their data safely for business insights and intelligence.
While cloud activity does come with its own set of challenges, that doesn’t mean that data shouldn't be collected and analyzed in cloud environments. Cloud services can drive growth and fuel innovation, which is why organizations must successfully navigate privacy concerns to derive the most value from their sensitive data. The four basic steps outlined above will provide organizations with a starting point to implement a basic framework for privacy and security measures.
Image credit: everythingposs/depositphotos.com
Tom Kennedy is Director of Cloud & Technology Partnerships, Privitar