How biometrics is moving from verifying identity to verifying humanity

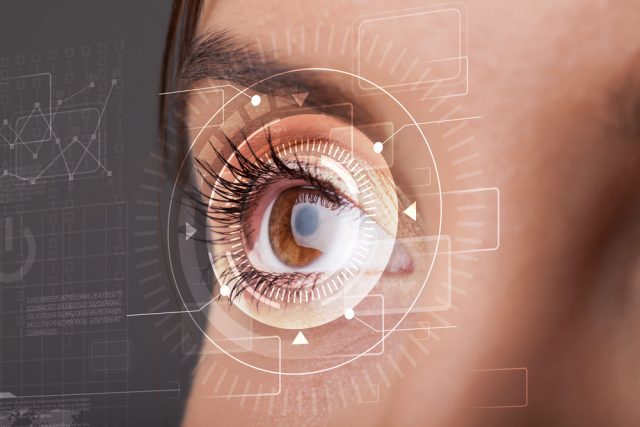
Even though 'face spoof' sounds like a piece of skateboarder terminology used to describe a particularly vicious wipeout, it’s actually something more commonly dealt with by InfoSec professionals who work for huge banks, telecommunications companies, and healthcare providers.
Simply put: it’s when a scammer uses a 3D mask -- or a printed or on-screen image -- to fool biometric security measures a la "Mission Impossible" in order to gain access to the system those security measures are in place to protect.
During the pandemic, there was an understandable surge in the demand for touchless biometric solutions, so the fear of those systems being compromised became a real topic of concern across industries. As remote workers in charge of managing sensitive data sought ways to unlock access to encrypted files using facial recognition, rather than manage a long string of individual alphanumeric passwords, biometrics had to find a way to add an extra layer of security to facial recognition.
Current methods to prevent face spoofing require the user to demonstrate to the biometrics software that they are alive and not an image by performing a series of physical actions -- but this method is prone to failure. There are multiple inherent drawbacks that need to be unilaterally addressed by developers in a way that simplifies the user verification process. With that in mind, the very concept of determining 'humanity' rather than 'liveness,' it would seem, is the way forward.
The unhackable selfie
There are very many ways to verify humanity, and one of them is to monitor activity. Current 'active liveness' checks implemented into biometric login software for some personal banking apps will give users a randomized set of instructions like, "turn your head to the left," and "chin up," or "look to the right," in order to prove that the user trying to log in is not a static image. However, this first wave of liveness checks has proved to be merely a stopgap on the journey to a better solution due to some immediately noticeable design flaws in how they request user information from the set of instructions they give.
Current 'active liveness' checks as described above have their limits. If you are told to your head to the left -- how can you see what your next instruction will be? And when are you supposed to turn back and look at the screen for new instructions? If the user turns too soon, the algorithm will abandon them from the service and force them to log in again. In fact, users abandoned enrollment 20-30 percent of the time because of active liveness checks. Our measurements have shown that just by switching from active to passive liveness checks, the ratio of people finishing the enrollment increased from 63 percent to over 99 percent. At the same time, they spent just one second doing the actual liveness check, compared to 13 seconds with the active approach.
The new 'passive liveness' checks use an algorithm to verify liveness just from a selfie. The way it works is by sharpening the computer’s vision to check the image for pixelation, photo edges, deformations of the face that would prove it’s not a real face but a rounded mask, and other discrepancies.
For even tighter control, all of the sensors built into the smartphone come into use by factoring in how the user is holding the phone, and where they are based geographically. If the user is in a strange location and the phone indicates it’s not being held in the way that would normally correspond to someone holding their phone out at arm’s length to look into their screenside camera, the algorithm will flag the login attempt and prompt additional security questions. The act of the user taking a selfie can also be algorithmically trained so that once the camera views the user in an optimal way, it snaps the best photo automatically.
Making faces
Passive liveness checks are based on a photo or a short stream of images fed into a vision algorithm trained to differentiate between genuine live people and clever scams. Because this technology is relatively new, it’s often confused with facial recognition technology -- which has been around for years -- so one of the biggest questions people have about it is "How does it work?"
The difference between building something based on machine learning and building something like facial recognition software is that there is an actual hands-on process when it comes to training a machine on how to think. With facial recognition software, for instance, there are international standardized datasets of millions of properly labeled images that can be fed directly into a neural network so it can learn. The companies then further refine and label them to improve accuracy.
With passive liveness checks, on the other hand, no such dataset exists and creating one has to be built from scratch. Everyone developing this software right now will have their own way of teaching their algorithm how to detect for liveness, so it’s a particularly interesting industry development.
When Innovatrics started building the algorithm, we created an almost 'automatized' setup to feed data into our neural network. Our research lab set up smartphones on stands, computer screens, printed images, and facemasks and ran them past the camera for the algorithm to crunch. After generating thousands of photos a day to train the algorithm in its initial stages, we deployed a collection app that we encourage everyone in the office to use -- which basically became a company-wide competition to see who can fool the algorithm the best.
The point of the app is to get everyone in the company to take selfies in low-light situations, contrast light situations (like taking a selfie against a window, so there’s lots of backlighting) or with colorful or patterned backgrounds in order to train the algorithm to function under those conditions. We also encourage people to take photos of faces of their computer screens or printed images or photos to train the algorithm that way.
As the neural network has developed, it’s become increasingly more difficult to fool the machine, so our staff’s attempts to do so have had to become quite creative.
An 'in-your-face' solution to help ease the bureaucracy
As biometrics gains widespread adoption, companies’ goal will be to lower the risk for the user while making everything more convenient. This means tailoring solutions that will require fewer steps, less algorithmic decision-making, and broader adaptability to lives lived through smartphones.
The public sector stands to benefit tremendously from adopting biometric technology by applying it to certain processes that are mired in antiquated bureaucratic technicalities. Some countries still require checking the identity by a physical person in a branch or post office. This is a requirement regardless of the fact that the technology is proven to be much more accurate at assessing identity than a human is.
With Covid, nobody wants to go to a physical location to prove their identity. It’s a nuisance -- it’s not really optimal. Once integrated, this new tech will help smooth over in-person processes that were once time-consuming and irritating.
As biometrics become ubiquitous, smarter security features to detect liveness and other new factors will ensure scammers are less successful in their efforts to unlock accounts using various spoofing methods. This means that in a few short years, your very humanity may be the password encoded into many of your seamless, secure, digital interactions.
Photo credit: ra2studio / Shutterstock
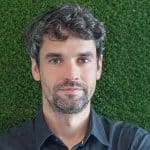
Jan Lunter is CEO & CTO of Innovatrics. Graduated at the Télécom ParisTech University in France. Co-founder and CEO of Innovatrics, which has been developing and providing fingerprint recognition solutions since 2004. Jan is an author of the algorithm for fingerprint analysis and recognition, which regularly ranks among the top in prestigious comparison tests (NIST PFT II, NIST Minex). In recent years he is also dealing with image processing and the use of neural networks for face recognition.