How to address the FTC guidance on AI today

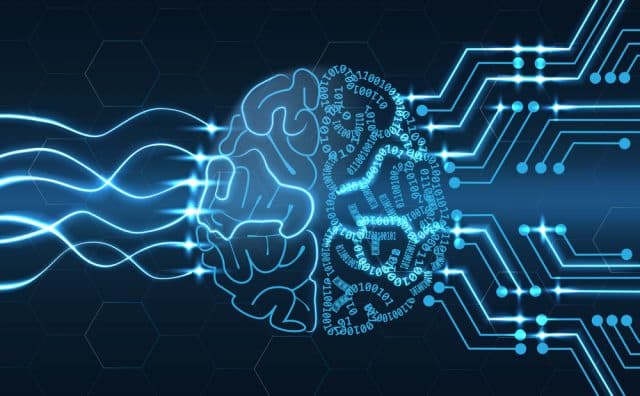
The Federal Trade Commission (FTC) recently published a blog entitled "Aiming for truth, fairness, and equity in your company's use of AI" that should serve as a shot across the bow for the large number of companies regulated by the FTC.
Signaling a stronger regulatory stance on deployed algorithms, the FTC highlights some of the issues with AI bias and unfair treatment and states that existing FTC regulations -- such as the Fair Credit Reporting Act, the Equal Credit Opportunity Act, and the FTC ACT -- all still apply and will be enforced with algorithmic decision-making.
The FTC outlined a set of tenets and recommendations for well-controlled algorithm deployments and this document serves as a pragmatic checklist for enterprises to consider when setting up their AI governance for deploying machine learning models to be in compliance with FTC regulations.
Start with the right foundation
The FTC advocates for a pyramid approach of good data, solid processes, basic analytics, and simple models prior to worrying about higher order modeling. In many presentations I do, I use the below graphic from Monica Rogati, who created The AI Hierarchy of Needs to make essentially the same point.
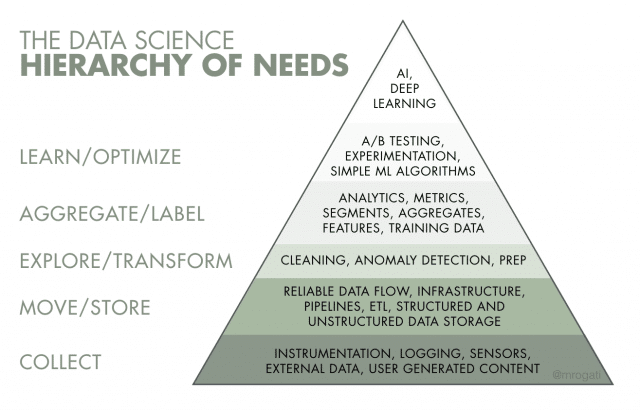
Without building machine learning/artificial intelligence (ML/AI) in the proper order, companies are much more prone to having issues with bias and opacity due to lack of data quality, established model management practices, and mature model deployment infrastructure.
The bulk of ML/AI infrastructure today is focused narrowly on pre-deployment phases and technical audiences. The ideal infrastructure must also support production environments and non-technical users in order to address the other concerns that the FTC outlines, from discriminatory outcomes to independent validation.
Watch out for discriminatory outcomes
Typically, companies fall into a trap of building their models using biased data unknowingly. By using common or internal datasets, with historical bias of humans embedded, they run the risk of building models that are not representative of the population they are attempting to model.
However, companies also need to take a full lifecycle approach to tackling bias since it's not just a data problem. They should also pursue periodic independent validations to determine if models are performing as expected and do not exhibit disparate impact upon different and disadvantaged populations.
Embrace transparency and independence
The auditing profession has been around in some form or another since the middle ages, and after the SEC bill of 1932, the independent audit has become a required annual ritual of all publicly traded companies. These days, no one would dream of investing in a large company without an independent validation.
In the world of algorithmic decision-making, companies have hitherto shied away from independent validation and certain elements of transparency often as a means to protect their IP, but also because of a knowledge and technology gap to make audits and validations possible. However, for models making consequential decisions about individuals, this type of "algorithmic mercantilism" undermines public trust in models. For algorithms that affect end users, independent validation is emerging as an important component of a well-assured modeling program.
In full transparency, we founded Monitaur with the vision of doing exactly that: independent assurance for models. We agree with the FTC that the use of objective standards and periodic validation by independent assurance providers ensures trustworthy algorithmic decision-making. The danger in having no objective, external review of models is that regulatory impulses will push much further than model builders would like.
Case in point, the FTC suggests that companies should open up their data and source code to outside inspection. Very few organizations would shoulder the risk to their intellectual property. More likely, such a demand would stifle innovation that could actually benefit the consumers that the regulators want to protect.
Don't exaggerate what your algorithm can do...
The guidance emphasizes that, under the FTC Act, statements to customers need to be truthful and backed by evidence. The hyper-competitive technology space and corporate pressures to signal innovation through AI has driven many companies to exaggerate their capabilities, sometimes calling systems "AI" when instead there are more basic rule-based systems with a strong degree of human process embedded. With the FTC comments, companies should consider that overstatements of AI might actually trigger unwanted regulatory scrutiny.
In addition to broad misrepresentation about AI capabilities, the FTC specifically calls out the danger of exaggerating about "whether [AI] can deliver fair or unbiased results." Automated decision systems are fallible, and companies need to take special care to independently validate decisions and thoroughly test their models on a periodic basis to ensure that bias does not begin to creep in. Unfortunately, the technical discussion about bias in AI has obsessed on it as a problem driven by data alone. This perspective overlooks other issues that cause bias as well. Claims about unbiased models will increasingly require proof of appropriate process and measurement of bias.
Tell the truth about how you use data
In the current mindset of "data is the new oil," many companies are not forthcoming with consumers about using their data for model training. Beyond the unethical business practice and risk for legal action, this will cause regulatory difficulty for a company under the FTC regulatory umbrella in the future.
The tide appears to be changing a bit with GDPR, CPRA, US states, and Congress passing and considering laws that forbid this type of data use without permission or disclosures. Within the technology space, the tide has begun to turn as well with developments like Apple's iOS 14.5, which affords user opt-in to data tracking, and mission-oriented tech foundations establishing principles and best practices. Companies would be wise to create or strengthen data governance programs in anticipation of multiple oversight bodies inquiring into how they use customer data and inform them of that use.
Do more good than harm and hold yourself accountable
We fundamentally believe AI can positively improve our everyday lives and in many ways, create more equity and fairness through transparent data and systems; however, without clear governance and responsible intentions, models run the risk of being unfair and causing more harm than good. Essentially, if a model makes a process unfair or less fair than existing practices for a protected class, the FTC will judge these models as unfair. Again, this stance places the burden of proof on the company building with ML/AI to show that they have compared new AI/ML products with previous offerings and documented the efficacy for the consumer before launch.
Bottomline, the FTC says to "hold yourself accountable -- or be ready for the FTC to do it for you." Accountability should be the primary driver of how companies assure their systems, thus why accountability for your algorithms' performance is a central control in the Business Understanding category in our ML Assurance framework.
Image credit: Laurent T / Shutterstock
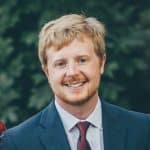
Andrew Clark is CTO and co-founder at Monitaur. He is a trusted domain expert, thought leader, and practitioner from the earliest days of the Machine Learning audit and assurance field. Most recently, Andrew built and deployed ML Audit and Assurance solutions at Capital One in support of their risk and audit needs. His contributions to AI & ML auditing include authoring ISACA's Machine Learning Auditing Guidance, contributing to ISO’s AI standards development, and assisting with the development of the ICO's AI Auditing Framework. Andrew is a featured speaker at ISACA's global conferences and a sought-after specialist by trade and media.