Breaking bias -- ensuring fairness in artificial intelligence [Q&A]

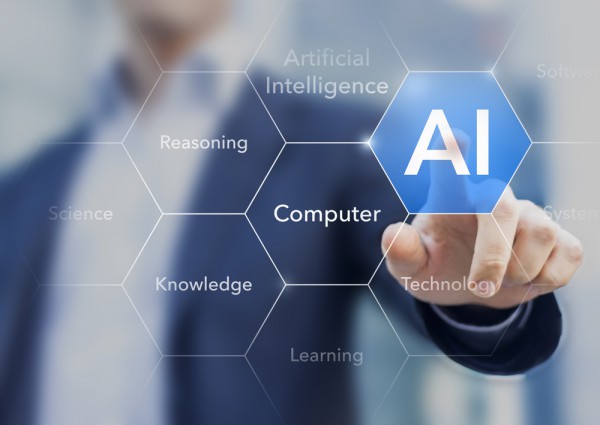
Artificial intelligence is creeping into more and more areas of technology, increasingly becoming the basis for commercial and other decisions, but bias can find its way in to AI systems and lead to results that are neither fair nor objective.
To prevent bias in AI businesses need to understand the different types of bias that can occur and know what’s needed to address each of them. We spoke to Alix Melchy, VP of AI at Jumio, to find out about the problems AI bias can cause and what enterprises can do about them.
BN: How does bias find its way into AI systems?
AM: Bias typically finds its way into artificial intelligence systems in one of three ways. The first instance is when machine learning models are overly simple and fail to capture the trends present in the data set. This is referred to as model bias.
The second is sampling bias, which occurs when the machine learning model uses datasets that do not reflect a country or region's demographics.
Lastly is fairness bias, which enters into AI systems through training data that contains skewed human decisions or represents historical or social prejudices.
BN: What sort of problems can this bias lead to?
AM: Bias in AI can lead to skewed results that are neither fair nor objective, most notably seen in unfair hiring practices or law enforcement screenings. As AI is adopted for an increasing number of business functions and data analysis, this bias is concerning to many experts. A few years ago, bias in AI made national news when it was discovered that a benchmark dataset used for testing facial recognition software had data that was actually 70 percent male and 80 percent white. This triggered a movement to address the bias issue in AI, which has been a productive shift, but there's still a lot of ground to cover especially as data and algorithms change all the time. On a larger scale, bias can be damaging to the credibility of AI as a whole, which is why we must explore new and existing methods of mitigating the problem of bias for this technology.
BN: What strategies can be used to ensure greater fairness and reduce bias?
AM: To ensure greater fairness and reduce AI bias it is first necessary to understand what factors are leading to bias -- if there are issues related to modeling, sampling or fairness -- and then it’s possible to navigate a solution.
When dealing with modeling bias, we want to ask the AI system many questions and test different scenarios within the data. Running these tests confirms if the model performance changes when a few data points change or when a different sample of data is used to train or test the model. Sampling bias, on the other hand, can be prevented by stratifying the datasets by the protected attributes of interest to ensure a balanced representation of the population. Lastly, to mitigate fairness bias, a starting point is prioritizing diversity in design and development teams because teams that lack any diverse perspective will create experiences based on their privileged background and abilities.
BN: When developing an AI project do you need someone on the team dedicated to overseeing its ethics?
AM: Assigning a diverse team of people -- not an individual, but a representative group -- to oversee the ethics of an AI project is a wise thing to do, but it has to go beyond that. When developing a system, organizations must consider the layers and dimensions that are built into the technology that ensure fairness as an integral part of the project. Fairness, anti-bias or ethics can never be a retraced afterthought; in other words, companies that don't build an AI system with bias considerations from the start are never going to catch up to an industry-standard level of accuracy. While dedicated ethics professionals can enrich the perspective of a team building AI systems, expertly trained data scientists, with the proper level of awareness, are essential to building ethical systems from the start.
Photo Credit: NicoElNino/Shutterstock