Bespoke machine learning is a must to tackle FaaS underworld

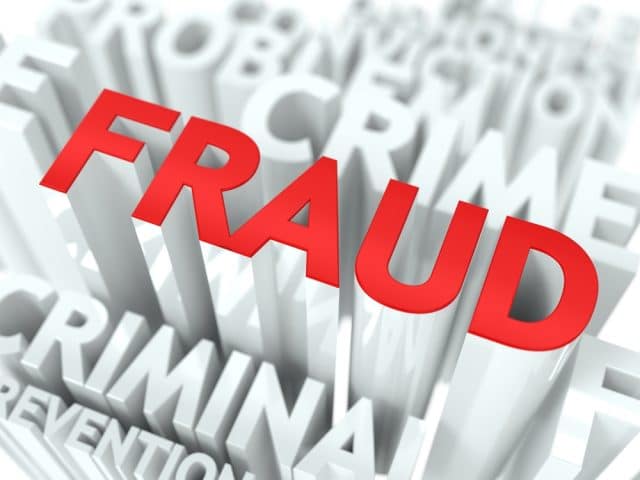
When it comes to fraud, you can never be too careful. Especially when you hear about the brass neck of some criminal gangs that are increasingly adopting the persona of legitimate businesses to peddle stolen credit card details and other financial information.
Fraud-as-a-service (FaaS), as it’s known, has become an industry in itself, with criminals able to provide a one-stop-shop for scammers to rip-off customers and businesses. These organized fraud rings -- often manned by career professionals who know how to bypass rules-based systems -- are becoming increasingly sophisticated.
This is not about chancers trying their luck as opportunist fraudsters hoping to get away with small change. This is digital fraud not as a cottage industry but on a conglomerate scale. As FaaS operators have become more sophisticated, they’ve hijacked the language and techniques used by legitimate business -- such as "free trials" and "service level guarantees" -- to attract new customers.
The emergence of more organized FaaS suppliers -- the international wholesalers of the fraud underworld -- is opening the door to a whole new wave of criminals who don’t need the skills themselves to set-up online racketeering. All they need is to be able to pay for it and earn enough of a margin to make the whole thing worthwhile.
FaaS promotes a façade of corporate legitimacy
Whether you call it FaaS -- or view it as distortion of traditional white label business models -- it’s clear that financial criminals are transforming their operations to cash in on crime.
To combat such cybercrime, businesses have traditionally turned to off-the-shelf packages to stop fraudulent payments in their tracks. Often these are rules-based solutions that tend to be a blunt instrument in the fight against cybercrime.
For instance, research tells us that "high-value orders" -- and orders from "high-risk locations" -- are more likely to be fraudulent. The snag is, if you establish a rule to weed out "all transactions over $500", for example -- or every payment from a "risky region" -- you could potentially block a raft of genuine transactions as well.
One way to overcome this is simply to keep expanding the book of rules to keep pace with the exceptions as they arise. Again, this may work for a while, but invariably, those creating the rules end up tying themselves in knots. And what you’re left with is a tangle of code that can gum up security platforms making them cumbersome and unresponsive. In some cases, it requires manual intervention from IT teams at a time when fraudsters are developing smarter, faster, and more stealthy ways to commit fraud online.
Worse, the career criminals behind FaaS have a pretty good idea what generic or common rules may be introduced. They constantly test systems looking for weaknesses to see to what extent they can defraud merchants before setting off alarm bells. After all, it’s their job to stay one step ahead of legitimate businesses. Crime is their business.
Why machine learning is best suited to fraud detection
So, while a rules-based approach has its merits, new threats need a different, more sophisticated approach. Which is why the adoption of machine learning has proven to be so effective. And it’s given an even greater edge because the more information it processes -- the more it is able to make decisions about the data.
Machine learning in cyber fraud protection allows you to process hundreds of thousands of queries and compare the outcomes to find the best result. If something looks out of the ordinary, it can be flagged for closer examination and a transaction blocked if fraudulent. Instead of teams of analysts manually wading through the data, it can be done automatically in real-time and takes less than a blink of the eye.
Of course, as criminals become more sophisticated, it’s possible that they may initially breach fraud defenses even if they’re reinforced with machine learning. But, unlike rules-based approaches, machine learning will pick this up, analyze and identify the underlying methodology used by fraudsters before creating better, more robust barricades.
That’s the beauty of machine learning when targeting committed fraudsters. The more information it receives, the better able it is to distinguish between legitimate spending patterns compared to the criminal behavior of fraudsters.
Rules and machine learning join forces for fraud detection
As a step forward in tackling cybercrime, there is no doubt that machine learning has become the new front line in combating fraud. But it doesn’t mean you should give up using rules completely. Any anti-fraud strategy should still include some rules where it makes sense, and also incorporate the benefits of machine learning technology.
What’s more, if you really want to beef up protection, businesses must rely on a combination of bespoke machine learning models based on -- and tailored to -- their specific purchasing characteristics.
But what if you don’t have enough data to train your own model?
The answer is simple. Just make sure you work with a payment fraud protection provider that has enough anonymized historical data from similar business types and payment demographics to get you started.
And as soon as the model starts working on your data it will begin to adapt and tailor to your customer base, and therefore become more effective. The more data it receives the better it becomes at spotting fraudulent activity.
With cyber fraudsters becoming ever-more sophisticated, it’s up to legitimate businesses to up their game and do everything they can to protect themselves. That means being security conscious. It means staying up to date with ever-changing threats. And it means a rules-based approach backed by bespoke machine learning in the fight against FaaS.
Image credit: tashatuvango/depositphotos.com
Mairtin O’Riada, is co-founder and CIO, Ravelin.