Could democratization of AI help prevent the Great Resignation? [Q&A]

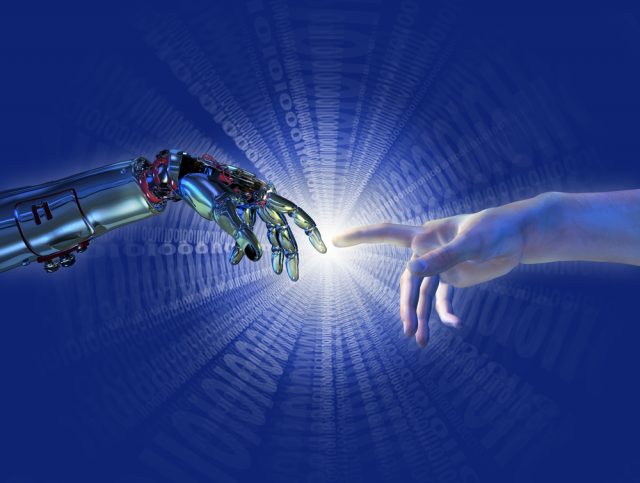
The Great Resignation has hit the IT industry harder than most, with recent figures from Gartner suggesting only 29 percent of global IT workers have a ‘high intent’ to stay in their current role.
AI is sometimes blamed for reducing the number of jobs, but could a democratization of AI in the workplace help retain staff by giving them the skills to be more involved in the flow of work?
Mike Loukides, VP at O'Reilly thinks so and we spoke to him to find out why.
BN: Can you explain what you mean by 'democratization' of AI?
ML: AI started off as a very specialized sector of computer science. To do anything worthwhile, you needed at least a master's degree or probably a Ph.D. Currently, there are many 'regular' software developers who are building AI systems. And it's likely that low code systems, like AutoML and SageMaker, will allow people who aren't professional software developers to build AI models. These are people like sales managers, marketing managers, and executives who want to use AI to make better projections and to conduct planning. People are now able to build the AI tools they need without a team of specialists.
In theory, that's what democratization is. It will be interesting to see how it plays out, especially as we look at two major considerations. First, it helps to have a background in statistics to understand exactly what an AI model is doing and whether it's working correctly. There's a good chance that managers and executives might have a better grounding in statistics than the typical programmer. Second, ever since we started talking about 'big data,' we've been saying that data cleaning is 80 percent of the problem and that's still true. To build an AI system, you need to collect data, prepare it, clean it, and store it. To run an AI system in production, you need to build pipelines to bring in new data. That's still 80 percent or more of the work, and it's something that the current low code tools don't help with.
BN: How is this going to change the development role? Are tech jobs generally going to look different?
ML: There will be a lot of new jobs that are focused on building the low code tools that others will use. We're only at the start of this movement.
A lot of developers will still be needed to do all of the data work mentioned above. Some of this falls under traditional software development and some falls under 'DevOps'. The point of DevOps is that the boundary between the development and operation blurs. However, AI doesn't fit very well into DevOps, and the whole deployment side of AI is going to require a lot of work on tools and systems.
BN: What are the main areas firms should be looking at to reskill their employees?
ML: I hated statistics when I was in college. It was probably the worst grade I got. I really learned nothing from the course, and everything I know about it now I had picked up later on. A lot of programmers feel the same way. If we're talking about AI, statistics is a must. Any programmer can learn Python, and the big AI platforms like TensorFlow and PyTorch are just more libraries to learn. Those are important, but they're not going to be a big deal for anyone who's already in the industry. Let's face it, statistics is difficult.
Software developers are also going to need to learn specific data skills: How you collect data, how you test it for bias (which is statistics), how you build data pipelines, and so on. The demand for data engineers is outstripping the demand for specific AI expertise.
BN: How important are the surrounding technologies like data science and cloud?
ML: It's not really clear what the boundary between data science and AI or machine learning is. I've always seen AI and ML as an outgrowth of data science. The next generation, as it were. The data skills I've mentioned are classic data science. A good argument can be made that lots of problems that people are trying to solve with AI can be solved more simply and effectively with older 'data science' techniques. You don't need a neural network if you can solve your problem with k-nearest neighbors (k-NN). I've actually seen k-NN used as a homework assignment in a first-year college course.
As far as the cloud is concerned, I believe most large-scale computing is going to move to the cloud. In O'Reilly's latest Data and AI salary survey, we saw that the most common certifications that professionals obtained were cloud certifications. I still think that we're only in the early to middle stages of moving to the cloud. There's still a long way to go.
BN: Why will online training be key to delivering this change?
ML: We're currently finishing up a survey which asked cloud professionals about their career path. One of the most interesting findings is that over 90 percent of the survey respondents work remotely at least one day per week, and almost two-thirds work remotely 100 percent of the time. We're seeing this as a permanent shift. People aren't going back to offices, and remote work is an environment for which online training is most ideal.
This is also why learning in the flow of work is vital to employees and tech professionals. Online learning in the flow of work allows an employee to easily get answers to assist a project they're working on, then quickly return to their work. At O'Reilly, we have tools that improve interactions with instructors and allow developers to practice what they're learning in sandbox environments. This is especially important so developers can learn by doing instead of taking long, drawn-out classes or trainings that aren't necessary. By providing ongoing, flexible learning in the workplace, virtual training has the ability to revolutionize the L&D landscape.
Image credit: AlienCat/depositphotos.com