Why Data Quality is critical for business

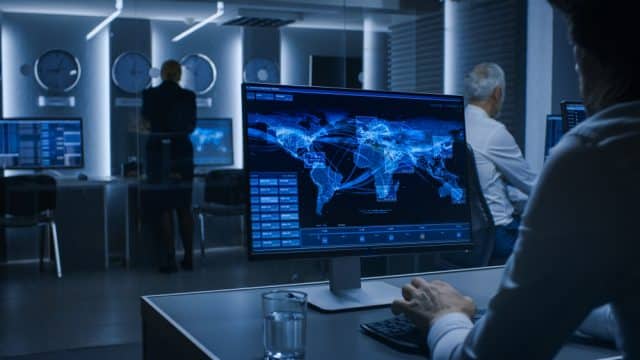
Since the explosion of technology in the last few decades, data has been increasingly positioned as a silver bullet that can fix all the trials and tribulations of the modern world. For those giant tech companies who amassed mass amounts of (mostly) third-party data, data was the new oil -- sold in barrels to any company wanting to find and scale an audience. But KPIs on data effectiveness became increasingly viable, businesses began to question the amount of data they’d bought.
Parallel to this, governments and consumer rights groups became aware of the increasing volume of unwanted noise being thrown at potential clients and customers. Businesses, both B2B and B2C, became liable for data missteps -- case in point with Meta being fined 17m euro for what amounted to bad data housekeeping.
Despite this, data -- in particular, 'big data' -- has risen to be a focal point for businesses, a must-have to build and maintain growth. However, this eclipses the fundamental truth: when it comes to data, effectiveness isn’t about quantity, it’s about quality.
Which is more valuable: A dataset of 1000 anonymous prospects who have never bought from you, or a properly maintained and detailed dataset of your 100 best customers? Needless to say, the latter comes out on top. But this has taken time for companies to realize. As more and more businesses recognize this point -- it’s also become clear that doing something about it is proving hard for many.
Data quality, governance, and access issues stand in the way of many organizations making the most of the data that they -- in theory -- have at their disposal. And as the quantity of data and the speed with which it is generated both increase, many businesses are finding that they simply need better systems and processes for managing and accessing data.
Making data count: Data Quality
For those at the forefront of making data count, Data Quality (note the capitals) is a recognized term whose definition has evolved beyond the obvious 'data of quality'. According to the Data Management Body of Knowledge, the accepted definition is that Data Quality "is the planning, implementation, and control of activities that apply quality management techniques to data to ensure it is fit for consumption and meets the needs of data consumers."
The core of Data Quality can be broken into constituent parts of whether it’s complete, valid, timely, unique, accurate and/or consistent. Whilst these may seem relatively basic, many businesses fail to properly run hygiene on their data, seeing it as a Sisyphean task that won’t yield a return on costly time investment. Yet -- ironically -- to view 'data' as the overwhelming, never-ending final task on a business’ to-do list completely misses the end goal of proper data management, which is to find and squeeze out data’s most important dimension: value.
Data Quality: A trinity approach to value
Try breaking down data quality value into three distinct areas: business value, risk and financial value, attributable to any single piece of information. By adopting this trinity approach, analysts and other data practitioners (internal and external) can assign ownership and priorities to different core areas of owned data, across any data quality project.
In reality, this entails assigning tangible values to data, so to return to our trio of values, this might look like:
- Business value: Chances are that employee salary data isn’t much use to the product dev teams, but something HR would find great value from, likewise customer email addresses for the marketing department
- Risk: What personally identifiable Information (PII) are you holding? If not adequately protected, a business is likely exposed to GDPR fines, or similar, depending on geography
- Financial value: E-commerce companies tend to find most financial value from data -- email addresses and credit card numbers are the bare minimum required to transact. Profiling the data and keeping it updated and of the highest quality possible enables e-commerce businesses to gauge average customer value and build sales
The benefits of enacting best-practice Data Quality are myriad, but specifically, there are a few key and clear benefits:
Firstly, there’s an undeniable boost in ROI from marketing activity, given the increased reliability of targeting from better data. It also enables both marketing and product teams to synchronize on personalizing product offerings -- something your customers increasingly expect. Then there’s improved and quicker decision-making from the increased visibility each internal division will have over their fiefdoms.
Perhaps less interesting to most employees, but still vitally important, is the increased compliance with existing and new regulations as they are brought in. And finally, but by no means least, is the time saved from fixing what we call 'dirty data' -- my own experience suggests that between $1-10 can be saved per record with best practice Data Quality.
The risks of immature data practices
Immature data practices can lead to some significant business-critical risks if left untamed. As touched on, but worth reiterating, there’s wasted marketing spend from inaccurate customer data, and potential fines from non-compliant data -- as we know with GDPR this can be 20m Euros or 4 percent of annual turnover, the EU will happily take whichever is higher! Beyond this though, there’s the core damage it does to the functioning and profitability of the business day-to-day.
In our tech-enabled world, businesses are constantly iterating and evolving or they face being dominated and wiped out by aggressive competition. Poor data quality can hinder modernization projects, as legacy systems are updated without correct mapping or poor data. It also prevents AI and machine learning from working their magic, as the output is only as good as the data input. It also wipes out the ability to be truly strategic. Data has the power to help businesses anticipate the future - whether through evolving customer buying trends, or spotting where the next warehouse/office should be located -- so it’s not just the immediate customer experience that fails when data quality isn’t put front and center of an organization's focus.
Data Management: 'Great Techpectations'
So where to start? Arguably the first and most important step to fixing a problem is recognizing you have one. With this in mind, businesses of all shapes and sizes should profile their data to better understand the current state of (data) play. There are a few data management tools, many with free versions for smaller businesses, where you can begin on your path to better data quality. And for those who’ve already set out on their Data Quality quest, there’s always value in looking again at where things stand, and always even small improvements that can reap huge rewards.
Photo Credit: Gorodenkoff/Shutterstock
Nick Stammers is VP of UK & EMEA, Ataccama.