Amid ChatGPT's rise to fame, how can enterprises work to eliminate AI bias?

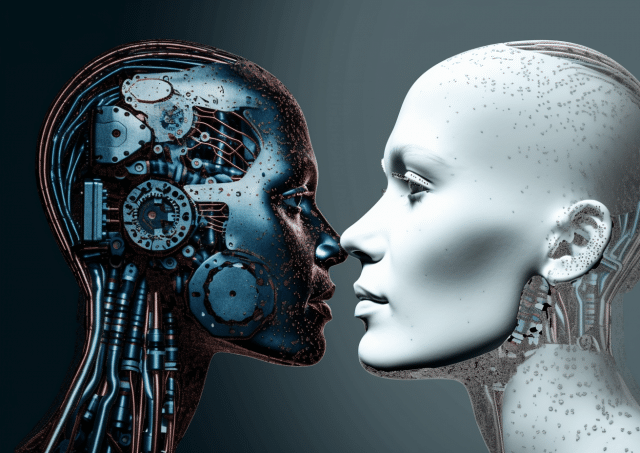
Artificial intelligence continues to hog the headlines, as more people discover the power of tools like OpenAI’s DALL-E 2 and especially ChatGPT. These futuristic-seeming tools work by taking a human’s query or prompt and returning an intelligent textual or visual response.
From an enterprise perspective, AI adoption is growing rapidly. According to Forrester, spending on AI software is set to accelerate from $33 billion in 2021 to $64 billion in 2025 -- growing twice as fast as the overall software market. But while tools like ChatGPT may seem like magic, it’s important to understand these solutions aren’t perfect.
In particular, enterprise leaders should grasp one of the most pressing concerns they raise: AI bias. Also known as algorithm bias, AI bias occurs when human biases make their way into algorithms. These biases can be pre-existing; when human programmers create algorithms, they may inadvertently (or even deliberately) select a limited range of input data, or input data from a biased source. AI bias can also arise due to the limitations of the particular algorithm being used.
AI bias isn’t a minority concern, either. More than 50 percent of organizations are concerned by the potential for AI bias to hurt their business. But what exactly is the issue, and why should enterprises care?
The impact of AI bias
Generally speaking, AI that produces offensive results can be attributed to the way the AI learns, and the dataset it is using. If the data over-represents or under-represents a particular population in a particular way, the AI will repeat that bias, generating even more data that further pollutes the data source and its own decision-making.
Take the case of the Allegheny Family screening model, which helps decide whether a child should be removed from a family due to abuse. Here, the bias reflects a broader societal prejudice: the model’s training dataset only comprises publicly-available data meaning it overlooks families that can afford private care providers. Similarly, in healthcare, AI software for detecting melanoma appears less likely to work on people with darker skin as many of the datasets used to train this software use images from Europe, North America, and Oceania exclusively.
In a British context, a UK government-backed study published in the British Medical Journal in 2022 found that AI models built to identify those people at high risk of liver disease from blood tests are twice as likely to miss disease in women as in men. And finally, a 2019 UC Berkeley study found that AI used to allocate patient care assigned black patients lower risk scores than white patients, despite the fact that the black patients were statistically more likely to have comorbid conditions, and so in fact experience higher levels of risk.
As a result of these inequities, companies could risk serious reputational damage. Indeed, one recent survey of UK and US IT chiefs found 36 percent of businesses have been negatively impacted by AI bias, resulting in lost revenue and customers. The same survey found a loss of customer trust is viewed as the main risk arising from AI bias, with over half (56 percent) of executives citing it.
While some believe ChatGPT has the potential to weaken Google’s dominance of the search-engine space -- or even to usurp Google altogether -- cases like the Berkeley study call this into question. Indeed, Google’s AI chief Jeff Dean has dismissed ChatGPT’s potential threat along these lines, pointing to widespread, pre-existing trust in the integrity of Google’s search results.
Addressing AI bias
Eliminating the biases present in human judgements is a daunting task, and as social scientists have suggested, bias may be an inevitable feature of human brains. Thankfully bias in data sets can be reduced and mitigated.
Data scientists must be trained to better curate the data they use, and to ensure ethical practices are followed in collecting and cleansing this data. They should also strive to preserve and promote high-quality data.
As for the underrepresentation of particular groups, the best solution here is transparency. By ensuring data is 'open' and available to as many data scientists as possible, we can ensure more diverse groups of people can sample the data and point out inherent biases. Using these experiences, we can also build AI models that will 'train the trainer', so to speak, when it comes to identifying biased data.
Taking this a step further, it would also be helpful to remove other data which is correlated with protected information, such as postcodes, which could be used to exclude certain demographics.
A holistic approach
AI bias can have grave consequences for enterprises, and as we’ve seen those consequences can easily spill over into wider society. Whether the consequences are a general mistrust of AI; poor business decisions; or decisions that harm the welfare of whole communities, all of society must come together to solve AI bias.
It’s incumbent on data scientists, enterprise leaders, academics and governmental agencies to work together: sharing data freely and openly to arrive at a point where we can place greater trust in AI. Quite simply, AI bias is too complicated and too important an issue to be tackled any other way.
Image Credit: Wayne Williams
Ravi Mayuram is CTO, Couchbase.