The real risks of OpenAI's GPT-4

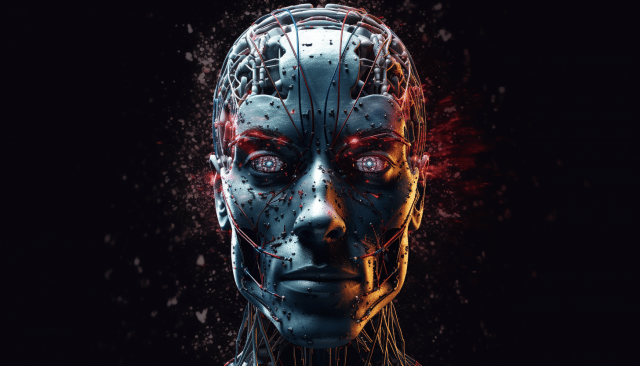
While many were marveling at the release of OpenAI’s GPT-4, Monitaur was busy analyzing the accompanying papers that examined the risks and technical design of its latest engine. In this commentary, I examine this through the lens of proper governance, responsible use, and ethical AI, while also considering the larger landscape of language models within which OpenAI sits.
The analysis results were not what were hoped for.
The potential risks, both known and unknown, of GPT-4
"The additional capabilities of GPT-4 also lead to new risk surfaces."
At a high level, the System Card calls out a few risks that were considered in their review, which they broadly associate with large language models (LLMs). We call out other implied risks below.
The risks listed have been categorized and reordered for better understanding. Relevant quotes from the document have been included for context. It is important to note that these risks are interconnected and should not be viewed in isolation.
- Hallucinations (as defined in the document)
- Automation bias (defined as "overreliance" in the document)
- Susceptibility to jailbreaks (referenced in the document)
- Bias reinforcement (referenced in the document as sycophancy)
- Scalability (alluded to in the document)
Hallucinations
"[GPT-4] maintains a tendency to make up facts, to double-down on incorrect information, and to perform tasks incorrectly."
As a probabilistic LLM, GPT-4 lacks the ability to assess the factual or logical basis of its output. To avoid potential errors, expert human review and critical thinking skills are necessary. Additionally, GPT-4 has shown a level of persistence in its mistakes that previous models did not exhibit. It cannot be guaranteed that tasks requested of it will be completed accurately.
Ultimately, this risk of the model hallucinating is foundational to many, if not all, of the additional risks in the list. For example, the authors draw a direct line to automation bias, saying that "hallucinations can become more dangerous as models become more truthful, as users build trust in the model when it provides truthful information in areas where they have some familiarity."
Automation bias ("Overreliance")
"[GPT-4 hallucinates] in ways that are more convincing and believable than earlier GPT models (e.g., due to authoritative tone or to being presented in the context of highly detailed information that is accurate), increasing the risk of overreliance."
GPT-4 produces a very effective mimicry of human voice thanks to its ability to process massive amounts of human communication. Without close observation and potentially well-designed training, average users cannot distinguish between its output and actual human productions. As a result, we are prone to the influence of automation bias -- essentially believing that the "machine" must be correct because supposedly it cannot make mistakes.
This psychological effect is a legacy of the largely deterministic world of technology prior to machine learning models. However, our collective ability to process and interpret these more probabilistic models has lagged. The authors predict that "users may not be vigilant for errors due to trust in the model; they may fail to provide appropriate oversight based on the use case and context; or they may utilize the model in domains where they lack expertise, making it difficult to identify mistakes. As users become more comfortable with the system, dependency on the model may hinder the development of new skills or even lead to the loss of important skills."
Another characteristic trained into GPT-4 is a "epistemic humility" -- a communication style that "hedges" responses or refuses to answer in order to reduce the risk of hallucinations, which can include hallucinations about its own factual accuracy. Our familiarity with these patterns is likely to overlook and trust the model too much.
Susceptibility to jailbreaks
"GPT-4 can still be vulnerable to adversarial attacks and exploits or 'jailbreaks.'"
Although not present in the document’s list of risks, GPT-4 is extremely susceptible to users tricking the model into circumventing the safeguards that OpenAI has built for it. In many cases, GPT-4 will "refuse" to answer questions that violate OpenAI content policies. However, a very large number of jailbreaking patterns were documented by users on social media and other online venues.
- Alter ego attacks -- Ask the model to respond as another model without restrictions (e.g., do anything now, aka DAN), as an evil version of itself in parallel, in the voice of specific public figures or celebrities, etc.
- System Message Attacks -- According to the report, "one of the most effective methods of 'breaking' the model currently," system messages provide the model with behavioral guidance along with a user prompt that can generate undesired content.
While OpenAI has taken some steps to mitigate jailbreaks, they will have to play whack-a-mole with these methods of attack as they arise because of the black box nature of the model. Human creativity in the hands of bad actors opens up an enormous number of untestable, unpredictable vectors of assault upon the boundaries, and given the scale of usage, the amount of moderation and mitigation could very well overwhelm OpenAI’s ability to address the volume. There is the additional risk of playing one LLM against another to further scale jailbreaking patterns.
Bias reinforcement, or sycophancy
"[GPT-4] can represent various societal biases and worldviews that may not be representative of the users intent… [which] includes tendencies to do things like repeat back a dialog user’s preferred answer ('sycophancy')."
As with all models powered by machine learning, GPT-4 is directly influenced by the biases that exist in the data on which it was trained. Since its data set consists of internet content on the largest scale to create its advanced language production capabilities, naturally it contains all of its biases.
But the System Card notes separately that the model additionally learns to create a sort of information bubble around users by recognizing what each individual prefers in answers. Hallucinations, of course, enhance the dangers of sycophancy because the model has no ability to sort fact from fiction, and thus the fictional "world" presented to users can grow entrenched.
Scaling risks
"Overreliance is a failure mode that likely increases with model capability and reach. As mistakes become harder for the average human user to detect and general trust in the model grows, users are less likely to challenge or verify the model’s responses."
The point of taking advantage of modeling approaches in general is that they allow us to radically scale our abilities to process information and act upon it, whether that information is reliable or not and whether the action is beneficial to all stakeholders who could be impacted.
This fact was perhaps so obvious to the authors that it was not worth calling out as a key driver of risk. But the ability to scale -- particularly at the incredibly low price points at which OpenAI is offering API access -- multiplies every risk covered in this analysis. Hallucination, automation bias, and sycophancy are very likely to worsen as usage increases. They will not become more manageable or easier to mitigate with scale but far more difficult to do so if not adequately equipped to assess underlying models and their inherent risks.
Considerations and next steps with GPT-4
Companies that want to consider employing generative AI need to have a strong understanding of the risks and how to mitigate them. Although generative AI has the potential to augment worker productivity, its benefits must be weighed against false information and the time it takes to have an expert review generated documents. Having a strong grasp of where generative AI can be helpful, such as in generating outlines, versus where it isn’t -- actually drafting documentations on nuanced, technical, or where facts matter -- will be key.
This blog post has only touched the tip of the iceberg on potential issues with GPT-4. Out of scope for this document was data privacy and IP protection, among other risks. Stay tuned for subsequent posts unpacking consequential first-order risks, macro and systematic risks, as well as practical approaches that can be used to properly govern responsible use of generative AI.
NOTE: If individuals associated with these projects provide further details or we learn more about the process in media reports, we will update this post accordingly.
Image Credit: Wayne Williams
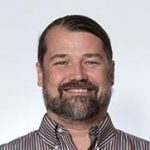
Tom Heys is the product strategy leader for Monitaur. With more than 15 years in startup SaaS leadership, he is dedicated to making the world better through technological applications of AI that are responsible and ethical. Tom holds a bachelor’s degree from Stanford University. For more information on Monitaur, please visit www.monitaur.ai, and follow the company on LinkedIn at www.linkedin.com/company/monitaur.