Developing AI models ethically: Ensuring copyright compliance and factual validation

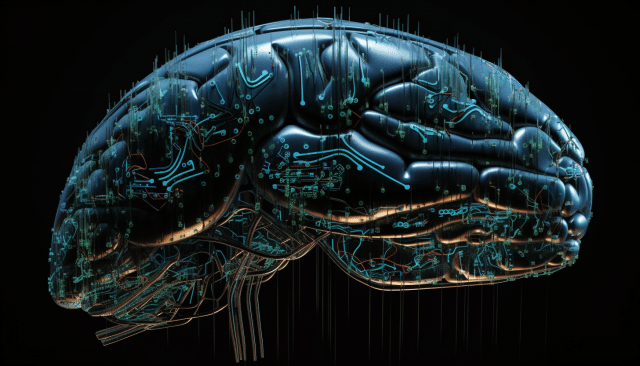
When constructing large language models (LLMs), developers require immense amounts of training data, often measured in hundreds of terabytes or even petabytes. The challenge lies in obtaining this data without violating copyright laws or using inaccurate information and avoiding potential lawsuits.
Some AI developers have been discovered collecting pirated ebooks, proprietary code, or personal data from online sources without consent. This stems from a competitive push to develop the largest possible models, increasing the likelihood of using copyrighted training data, causing environmental damage, and producing inaccurate results. A more effective approach would be to develop smart language models (SLMs) with a horizontal knowledge base, using ethically-sourced training data and fine-tuning to address specific business challenges.
Avoiding Copyright Infringement and Illegal Datasets
To ensure AI models comply with future regulations, developers must verify the sources of all training data. This is often easier for large corporations like Amazon or Microsoft, who possess vast amounts of user data. Start-ups, however, face difficulties in gathering comparable data whilst avoiding copyrighted material.
Firstly, developers should obtain the necessary permissions or licences to access and use selected datasets, and establish rules governing data collection and storage. Secondly, consider using smaller datasets to train models or fine-tune existing open-source alternatives. This simplifies data collection and verification whilst offering the opportunity to enhance reliability in specific domains or industries.
Synthetic training data offers another alternative, as it can achieve higher accuracy levels and circumvent copyright issues entirely.
Addressing Specific Problems
Developers should identify the specific problem they aim to solve, such as locating relevant papers within vast scientific research repositories, and train their model on a focused, labelled dataset from authoritative sources, like publicly-available academic research.
Ensuring Accuracy and Preventing Misinformation
By aggregating a high-quality, thoroughly-appraised set of training data, developers can ensure their models provide accurate, informed responses and reduce the spread of misinformation. Factual validation should be a key aspect of model architecture.
Developers should aim to construct models with more selective unsupervised learning, enhanced attention spans, and improved focus, using internal mechanisms to filter data before incorporating it into the training process.
Pursuing a Smarter Approach to Language Models
Current LLMs built by large corporations consume vast amounts of electricity and resources. For instance, training BigScience’s model, BLOOM, cost $7 million worth of grants on one of the world’s biggest supercomputers: Jean Zay in Paris. This is not only environmentally damaging but also highly inefficient. By refining the training process and focusing on specific use-cases, we can develop sustainable, futureproof models.
In specialized, technical domains, quality is significantly more important than quantity. Developers could also consider using a swarm of smart language model agents to tackle multiple facets of a business problem, rather than relying on a single LLM.
We must focus on 'data-centric AI' if we want to push the industry beyond where it is today. This means engineering the data required to construct specific AI models, improving data quality and labeling to match cutting-edge algorithms.
To create AI models that comply with copyright laws, developers should prioritize quality over quantity. They must research sources, understand data requirements for specific use-cases, and implement factual validation mechanisms to ensure accuracy. By working together, we can develop smarter language models, not merely larger ones.
Image Credit: Wayne Williams
Victor Botev is CTO and Co-Founder of Iris.ai.