The problem of unstructured data in foundation models [Q&A]

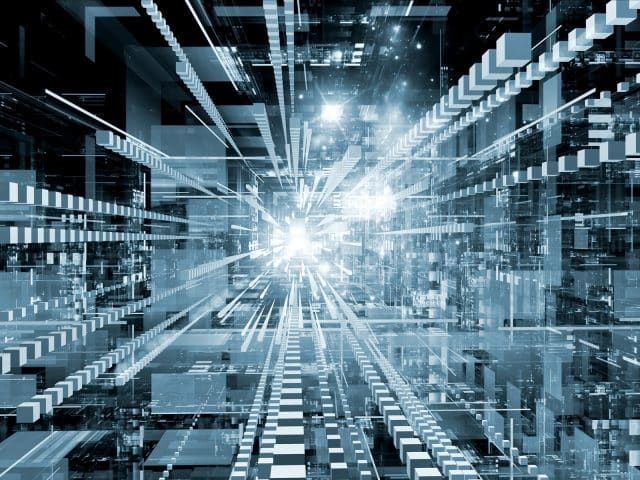
Artificial intelligence is only as good as the data that it has to work with and that means that large volumes of information are needed to train the software in order to get the best results.
Ensuring the quality of data therefore is a key task in any AI implementation. We talked to the CEO of Snorkel AI, Alex Ratner, to find out more about the issues involved and how organizations can overcome them.
BN: What are the major challenges companies face when working with AI models today, particularly language models?
AR: The most significant obstacle enterprises face in using AI, including the latest foundation models or large language models -- like ChatGPT, BERT, CLIP, Stable Diffusion and others -- is the vast volumes of labeled 'training data' required. AI models need ongoing data to learn from and remain up to date. The data must be classified and labeled, and the vast majority of data labeling today is still done by hand. It's costly, time-consuming and error-prone.
Manual data labeling also presents another major challenge: it makes it difficult to manage bias in AI-based systems, leading to potentially harmful consequences and compliance challenges.
BN: What is 'model hallucination' and how can it be prevented?
AR: Hallucination is one of the major challenges any company that leverages large language models is likely to encounter. Models like GPT-3 and ChatGPT are trained to produce the most plausible-sounding text given some prompt or context. They are not designed to optimize for the accuracy of facts, numbers or stats in that output. They are also not well trained to say 'I don't know'. And often, the response falls short of the truth. This is because, in the end, the model is as good as the data it is trained on, and a lot of the data produced in the world is unstructured. It's unlabeled and unclassified. Roughly 2.5 million terabytes of new data appears in the world each day.
In short, this is a very big data labeling problem. Inaccurate labels on training data can distort the model’s learning and conclusions, so that answers may be hallucinations and unpredictably very wrong.
Ultimately, a model isn't discerning like a human; it can't tell the difference between good data and, say, data containing toxic or unreliable content.
BN: Are enterprises ready to put these models to actual use now or is this more of a future proposition?
AR: The answer is both yes and no. If you take foundation models, the large-scale AI models trained on vast quantities of unlabeled data at scale, they are quickly being commoditized. They can be adapted to a wide range of downstream tasks, and large companies are intrigued and experimenting. Particular industries like finance, healthcare, and customer service are already active users. Specially, they are using proven models like BERT, but In other enterprises, though, upfront cost and ongoing cost do hinder adoption -- it takes investment, technology, and skilled people to run a foundation model.
There are also concerns about performance and privacy. The liability of issuing or acting upon a hallucinatory answer could turn out to be very significant. As for privacy, any task that involves access to a company's private information could risk exposure of that data, because the public-facing models draw on everything they've seen.
In specific enterprise use cases such as translation, a foundation model can work very well, as long as their work is verified. Interestingly, countless small businesses such as realtors are actively using AI models for marketing purposes. Many companies use them to quickly generate social posts, which they hopefully human-check before publishing.
BN: What are the key considerations for a company deploying language model software today? And what questions should a company be asking vendor soffering technology that runs on language model AI?
AR: It's really a calculation of the tradeoffs, and guesstimating some unknowns, like what are all the potential use cases and benefits from adopting a large language model (LLM). That gets weighed against the investment required (tech, talent, training and budget) and the risks, which are mainly about data privacy, liability, and wrong or subpar output -- not just hallucinations, but also boring and repetitive marketing copy.
Before adopting LLMs, companies should ask key questions of prospective vendors. For example, what is the risk of a LLM delivering an answer that is absurd or potentially damaging if acted upon? It's analogous to buying a self-driving car. You know it’s going to be great, but you don’t know if it will get a speeding ticket or think a pedestrian is just a shadow. Understanding the safeguards in place is very important.
Can we further train the LLM in our specific domains, so it becomes more reliable, and what will that cost? What’s really involved in labeling and preparing new training data so the model stays current? How do we use the model safely, so our confidential customer and company data won’t be exposed? How is this LLM better than another for our specific uses? I can go on.
Taking the step forward by acquiring a foundational model or vertically-adapted language model is a big commitment. You need to build up knowledge and expertise in AI models, and understand what skills and tools will be necessary to make it justify the price by delivering long-term, valuable performance. Plan some early successes with the project, as well as longer range possibilities. AI and use of language model software are inevitable; the biggest question of all is probably whether you move into it now, with the risks and benefits of early adopters, or you lag back to reduce risks which also means -- probably -- less competitive advantage from AI.
Image credit: agsandrew/ depositphotos