Leveraging advanced data for AI-powered Anti-Money Laundering (AML)

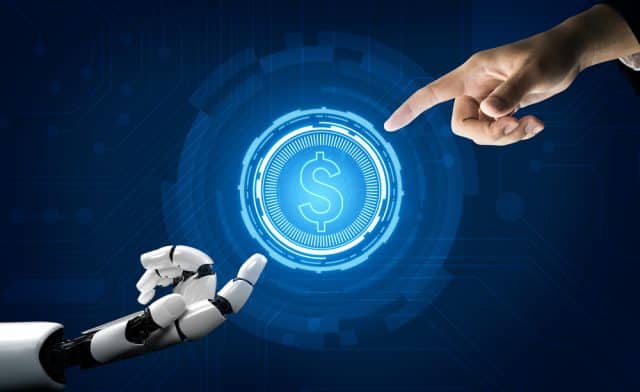
The global financial landscape is witnessing a transformation as institutions increasingly embrace the power of artificial intelligence (AI) to combat money laundering and fraud. AI's technical capabilities present an array of benefits for Anti-Money Laundering (AML) processes, particularly in its proficiencies for data analysis and pattern recognition.
Though, as AI integration gains momentum, it also brings significant challenges that demand thoughtful consideration. Issues such as limited training data and over-reliance without human supervision can have serious implications for AML efforts.
Data Analytics and Pattern Recognition in AI-Led AML
AI is a valuable tool for data analysis and pattern recognition, and compliance teams can leverage it to efficiently process vast volumes of data, enabling them to identify and act upon suspicious activities with increased accuracy. Real-time monitoring capabilities can empower financial institutions to stay ahead of bad actors, take timely action, and proactively prevent illicit transactions.
If institutions can fine-tune AI models continuously and strike the right balance between precision and recall, it should result in more accurate identification of suspicious activities and reduce the burden on compliance teams.
Unraveling Complexity
Sophisticated financial crimes require innovative solutions. AI algorithms are adept at unraveling intricate networks and hidden beneficial owners involved in illicit transactions. These beneficial owners could hold a significant interest in a company or financial account but conceal their identities to obscure the origins of fraudulent funds.
AI algorithms can be trained to unravel these intricate networks. By processing vast amounts of data from multiple sources simultaneously, AI can unveil intricate relationships and connections that might otherwise remain concealed.
AI systems can also process and analyze unstructured data sources, such as text-based reports, emails, and social media, which are often rich sources of information for detecting financial crimes. This capability allows compliance teams to access a wider range of data, enhancing their understanding of complex money laundering schemes.
However, navigating the challenges that come with AI adoption is essential. Limited data and an over-reliance on AI models without human supervision have the potential to hinder effectiveness. Ensuring access to comprehensive and diverse datasets to train AI models effectively is key. Human oversight remains crucial in interpreting the results produced by AI algorithms, especially in complex cases that demand a deeper understanding of related nuances.
Collaborative Approach to AI-AML Integration
Industry partnerships can facilitate the sharing of best practices, data insights, and algorithmic improvements. Collaborative efforts can address the challenge of limited data by pooling resources from multiple institutions, leading to more robust AI models that can adapt to evolving money laundering tactics.
Regulatory bodies will eventually play a pivotal role in AI adoption in AML efforts. Guidelines on the use of AI systems are being continuously developed and published, and firms will be expected to create processes conducive to responsible integration of AI technology.
The future for AI-AML
AI technology holds the promise of transforming the AML landscape. AI algorithms are still evolving, and as they become more adept at identifying subtle patterns and anomalies, we can hope to see increased accuracy in detecting suspicious individuals and transactions.
Financial institutions should remain cognizant of the challenges that come with AI adoption and respect the need for human oversight. As AI continues to advance, we can expect even more sophisticated solutions that will continuously improve the efficiency of AML efforts.
Image credit: BiancoBlue/depositphotos.com
Keith Berry is General Manager Know Your Customer Solutions, Moody’s Analytics.