Leveraging AIOps to keep pace with cloud-native complexity

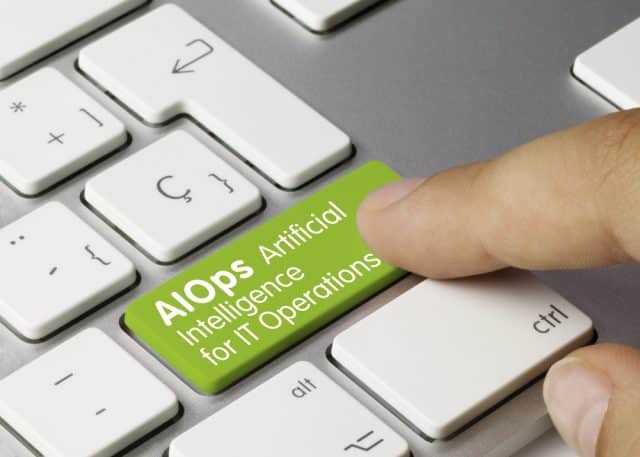
Companies have massively increased their cloud infrastructure investment in the relentless pursuit of innovation. Cloud-native apps, hybrid clouds, microservices, and serverless all enable companies to serve their customers with greater agility -- and at greater scale -- than ever before.
But the rapid adoption of these technologies has also created distributed cloud environments that are immensely difficult to understand and monitor with conventional observability tools.
And when something goes wrong, a lack of visibility and context into a company's production environment can become an existential threat. Outages and service degradations are harder than ever to troubleshoot in a world where infrastructure, applications, and network concerns are increasingly interconnected.
Companies need a new approach that accounts for the complexity of the modern production environment.
The Rising Complexity and Its Risks
Gone are the days of monolithic IT applications. Today, IT infrastructure is distributed across dynamic systems; most companies use two or more public and private cloud environments, and the use of popular managed services from cloud providers like AWS RDS and Google App Engine adds opaque layers to an organization’s infrastructure. Despite the benefits of cloud-native, the corresponding complexity can obscure system dependencies and make troubleshooting difficult, which poses management challenges.
Just a decade ago, for example, a typical e-commerce retailer operated on a straightforward monolithic architecture. This architecture encompassed the entire shopping experience, from user authentication and product selection to payment processing and order fulfillment. Fast forward to today, and that same e-commerce retailer has transformed into a labyrinth of complexity. In the modern landscape, this retailer relies on dozens or hundreds of SaaS apps to manage everything from customer relationship management to supply chain logistics. And the utilization of Kubernetes for container orchestration has become the norm, abstracting a myriad of interconnected microservices dedicated to various functions.
In the chaos of these modern distributed environments, DevOps teams find themselves constantly operating in reactive mode. An issue in one of these dozens or hundreds of SaaS apps and services may cascade into showstopper-level concerns, and DevOps must often scramble to just uncover the root cause. Distributed systems keep engineering teams preoccupied with defensive matters and take time away from new feature work.
Beyond impeding team efficiency, degrading performance, and stifling innovation, observability issues arising from popular dynamic architectures can introduce a higher risk of security breaches -- by making it harder to manage and control access, test thoroughly for vulnerabilities, and properly secure and audit. Couple that with soaring cloud costs, and IT teams face a true resource-strapped struggle to maintain visibility and control.
Fighting Back with AIOps and Observability
According to Gartner, AIOps "combines big data and machine learning to automate IT operations processes, including event correlation, anomaly detection and causality determination."
This sounds promising -- exactly, in fact, like what so many DevOps teams are painfully missing today.
But production environments are complex and noisy. Trying to identify meaningful correlations and infer causality at scale -- without deep context into the relationships between services, applications, infrastructure, third-party dependencies, and user impact -- is like trying to find a needle in a haystack.
That’s why it’s not enough to simply throw machine learning (ML) algorithms at a flood of observability data: the scale and complexity of modern distributed systems make this kind of naive approach ineffective. Rather, you need a way of overlaying context -- providing a "map" of the relationship between production environment components -- to begin making higher-order inferences.
One proven approach involves automated discovery of all components, mapping of dependencies to capture runtime dynamics, and creation of a graph (a "topology") of key workflows. These foundations provide the basis for real-time signal correlation to uncover hidden relationships and root causes. ML can be used to build a baseline telemetry and reduce noise, and rapidly detect anomalies even for unprecedented errors.
At its most powerful, an AIOps platform (with automated discovery, topology, and ML as described above) excels at uncovering "unknown unknowns," meaning stress points exposed during configuration or deployment updates. For example, if an internal API is unknowingly exposed to a customer, AIOps can map the new correlation between the interface and the end user.
Rich observability data is essential, but it requires high-fidelity metrics, logs, and traces. Observability platforms that use newer sampling techniques, such as extended Berkeley Packet Filter (eBPF), truly put the "AI" in "AIOps" -- these platforms don’t just detect anomalies, but use AI to analyze their context.
The Path Forward
The percentage of companies using distributed cloud environments is expected to surpass traditional IT environments by 2025, according to Gartner. Companies must embrace AI and observability to prevent complexity from hindering innovation. The genie can’t be put back in the bottle, and accelerating trends put the onus on companies to build up their observability stack to stay ahead of the curve.
The AIOps market is projected to reach nearly $650 billion by 2030, driven by cloud adoption and rising data volumes. The potential of AI and observability tools to simplify complex architecture continues to grow. By taking a higher tech, AI-driven approach to network performance monitoring, organizations mitigate the risk of serious disruption to their business.
As modern IT environments continue to reach new levels of complexity, companies must be prepared for the complications that accompany this innovation. Fortunately, AI and data-driven insights can enable organizations to stay one step ahead. Developing a thorough understanding of AIOps and observability to effectively leverage microservices and cloud-native tools is the key to managing this complexity -- before it manages you.
Image credit: Momius/depositphotos.com
Amir Krayden is CEO, Senser.