Three ways AI is changing the private equity game

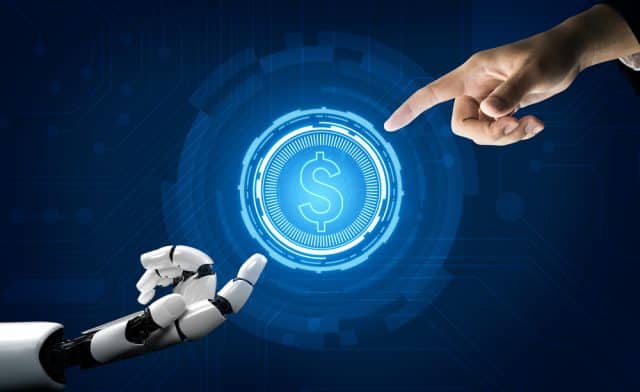
Financial market participants, including banks, insurance companies, pension funds, and hedge funds, are all actively exploring ways to leverage artificial intelligence (AI), and private equity (PE) firms are no exception.
Three areas that are showing a significant return on investment (ROI) for AI among PE firms are deal flow, investment pre-screening, and risk intelligence, because it’s these areas where AI is helping them shift their window to act earlier than their peers. The private companies that PE firms invest in are simply far less transparent than public ones: There’s just not as much information about them, and the information that exists is generally much harder to get.
AI is giving PE firms the ability to bridge this information gap so they can gather the private company intelligence they need to move faster than their competitors -- a critical factor in the private equity game.
Let’s first explore why that’s so hard today.
A Challenging Information Environment for Private Equity
PE firms require timely and accurate private company information, but the sheer volume of available data is enough to overwhelm even the best teams of analysts who need to source and process it all into actionable insights. While private companies aren’t as open and transparent, there’s still far more publicly available information on them than ever before due to the rise of digital media.
There’s also been a great increase in the operating complexity of the typical company as a result of globalization and new technologies. Companies are able to source, manufacture and sell their products in more markets globally than they ever could just a decade or two ago. PE firms must understand the global operating footprint of each company they’re exploring, including locations, subsidiaries, suppliers, owners, key executives and board members.
This creates a dual problem: Analysts simultaneously have too much information and not enough of the right information. They’re buried under a mountain of data, and it takes time and skill to process it all in order to produce insights that matter. And getting a complete picture of a company also demands data-gathering legwork, as some of the most critical data isn’t easily sourced.
This is where AI technologies such as natural language processing (NLP) and generative AI are helping PE firms overcome the information overload problem so they can work smarter, cover more research ground, and identify the unknown unknowns of companies and sectors faster and easier than before.
Private Equity AI Use Case 1: Deal Sourcing
Private equity is a hypercompetitive space, with firms vying to participate in a limited number of companies that are qualified for investment. PE firms that maintain better relationship networks and superior market intelligence operations will consistently get their foot in the door with prospective companies before others do. While AI doesn’t offer much help for improving personal networks, it can be a game changer for transforming a firm’s market intelligence.
The early bird gets the worm, and if firms want to be the first to engage with attractive prospective investments, they need to shift their window to act on new opportunities earlier than others.
Every PE firm must tackle the problem of how to best identify prospective deals, which involves searching public data sets and using search engines. This worked in the past because, well, it was the only method available, and every firm had to do it. But manual approaches to deal sourcing and identification no longer scale enough to be competitive.
PE firms are leveraging AI technologies like NLP to help analysts sift through mountains of information to find relevant insights from what seems like irrelevant data that others overlook. They then use generative AI to summarize it for easy consumption. This is significantly improving their deal flow compared to their peers, who are arriving at the table too late because they identified the opportunity too late.
Private Equity AI Use Case 2: Pre-screening
Once PE firms have identified an investment opportunity, they need to go deeper to understand the company, its operations and personnel, and the competitive landscape. This is essentially the initial phase of due diligence on the company that involves material, non-financial "soft information" analysis where even the best teams typically find themselves overloaded.
PE firms leveraging AI have a leg up here, too -- by helping them shift their window to act earlier.
Sometimes, the action is a "no" decision, and PE analysts that can identify reasons not to pursue a deal quickly -- before burning more time and resources -- are able to move on and focus on the most promising opportunities and avoid wasting time on investments that are aren’t a great fit or are unlikely to be successful.
Private Equity AI Use Case 3: Risk Intelligence
A major risk for PE firms is a public controversy that incurs serious damage to the reputation and brand of one of their companies. Only if they spot trouble brewing under the surface can they take corrective actions in time to head off major controversies. But controversy monitoring has become increasingly challenging, too.
Global company operations have more moving parts to keep track of; they also face more kinds of risks than ever before. Governments, customers and the public are closely scrutinizing company behavior, particularly on environmental, social and governance (ESG) factors -- the kinds of controversies that pose the biggest risks to a company’s brand.
Effective risk intelligence involves processing vast amounts of unstructured information, including news coverage in local languages, lawsuits, regulatory actions, and ever-changing regulations -- and producing actionable insights fast.
The complexity of this task has far outstripped the ability of analysts to manage, but it is another natural fit for NLP and generative AI because it helps us process through vast streams of information every day and understand relevance, sentiment and criticality. PE firms that leverage AI here have much better odds at avoiding catastrophe than those who don’t.
Recommendations
- Define your AI use cases, objectives and metrics. It is clear that AI techniques like NLP and generative AI can help PE firms solve challenges that involve processing large amounts of data into knowledge. Be clear about which stage of the deal cycle your firm finds this challenge most critical and how you will define and measure success.
- Focus on empowering your team with AI tools. Don’t look for AI as an opportunity to replace people, but rather, understand that AI has the potential to liberate your team from undifferentiated and time-intensive manual tasks so they can focus on higher value work.
- Put the right AI leadership in place. The success of any AI initiative depends on having people who intimately understand your use cases and how AI can be used to deliver differentiated value. They’re also critical for capturing and transferring the growing body of institutional knowledge that is essential for long-term AI success.
- Address AI’s flaws and risks head on. Generative AI tools like ChatGPT are insufficient for PE applications -- they’re outdated in their information, lack domain or task-specific reasoning, and often provide inaccurate results (i.e., hallucinations). Advanced models like retrieval augmented generation (RAG AI), which combine the strengths of retrieval-based models with generative ones, can effectively overcome these issues.
AI is a powerful tool that is helping PE firms to gain a competitive advantage. By shifting their window to act earlier to improve deal flow, investment pre-screening, and risk intelligence with AI, PE firms can make more informed investment decisions faster and protect their investments from potential losses.
Image credit: BiancoBlue/depositphotos.com
As first seen in AiThority.com.
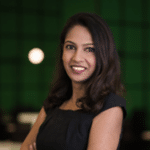
Chandini Jain is the founder and CEO of Auquan, an AI-powered research and data service that helps financial market participants identify and monitor risks in public and private companies across their global operations and supply chains. Prior to founding Auquan, Jain spent 10 years in global finance, working as a trader at Optiver and Deutsche Bank. She is a recognized expert and speaker in the field of using AI for investment and ESG risk management. Jain holds a master's degree in mechanical engineering/computational science from the University of Illinois at Urbana-Champaign and a B.Tech from IIT Kanpur. For more information on Auquan, visit https://www.auquan.com/, and follow the company @auquan_ and on LinkedIn.