Why ChatGPT won't solve your real-time translation needs

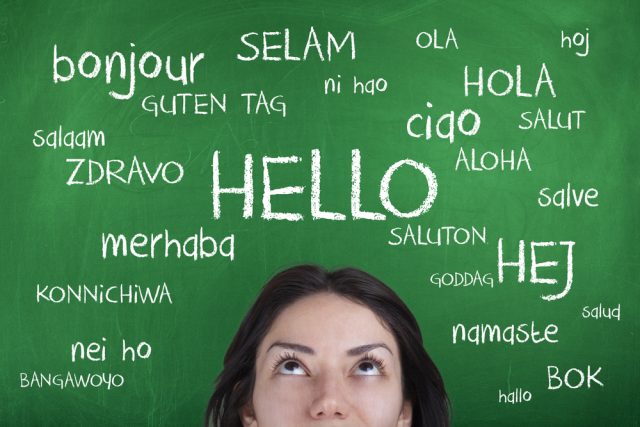
New technologies debut almost every day. This constant barrage of novel tools creates a perpetual cycle of overshadowing -- someone is always introducing a new technology that eclipses the previous innovation, and then something even newer comes out, and the cycle repeats itself. However, OpenAI’s ChatGPT broke that cycle.
Since ChatGPT’s debut in late 2022, the generative AI tool has exploded in popularity. It took just two months for the platform to reach 100 million users, a speed that shattered the previous record for fastest-growing app. The creators of ChatGPT expect the tool to generate $200 million this year and project that number will grow to $1 billion next year. Other businesses, like Google and Grammarly, are taking note. Both of these organizations have developed their own generative AI tool to enhance their business operations.
However, despite its huge user base and potential value, businesses should proceed with caution when relying on ChatGPT. The most recent version rolled out in May, and the fine print reads: "ChatGPT may produce inaccurate information about people, places, or facts." This possibility of inaccurate information extends to language translation as well.
When it comes to natural language processing (NLP)-based language translation, ChatGPT is on par with other platforms that use the same technology, such as Google Translate and DeepL. These large language models (LLMs) are computer programs trained on massive amounts of publicly available data and can interpret language and the intent behind it. Sophisticated LLMs can "understand" human language patterns and how they’re used in communication.
But even with the plethora of data these LLMs pull from, the quality of their translations is lacking for business and customer service uses. Let’s explore some of the challenges teams may face if they rely on ChatGPT or other LLMs for real-time translation needs.
ChatGPT’s translation limitations
In a business setting, real-time translation technology is extremely valuable, especially for organizations that have a global audience. Instead of increasing headcount with multiple polyglots, organizations that implement translation technology allows their team members to virtually communicate with customers in any language, boosting customer satisfaction and saving money.
Like other popular machine translation engines, the accuracy of ChatGPT’s translations is at its best when the text being translated is well-constructed. In real-time communication, however, syntax is rarely perfect. Common characteristics of real-time communication that affect translation quality include:
- Misspellings. In a customer service environment, spelling errors are common in text communication. However, trying to translate typos can grind operations to a halt. ChatGPT will likely mistranslate or not translate the misspelled word at all, leading to confusion for the representative and frustration for the customer.
- Colloquialisms. Industry-specific jargon or colloquial slang often includes words that have more than one meaning, and ChatGPT is more likely to translate words to their literal meanings than parse a figure of speech or informal language.
- Multiple languages. If a French-speaking customer includes an English word in their query, ChatGPT may not be able to recognize it as a different language. This trips up the translation technology and leads to inaccurate translations or no translation at all.
As outlined above, there are many ways to "confuse" ChatGPT and get convoluted, low-quality translations. Without additional technology layers providing context, ChatGPT’s translations aren’t reliable enough to be trusted in a business or customer service setting.
Real-world example of ChatGPT’s translation challenges
To get a better idea of ChatGPT’s translation constraints, let’s consider a message that was sent to a customer service representative in the sports betting industry. The original message was in Spanish and threw ChatGPT for a loop because it included spelling errors, industry-specific vocabulary, one English word, a tennis player’s nickname and an acronym.
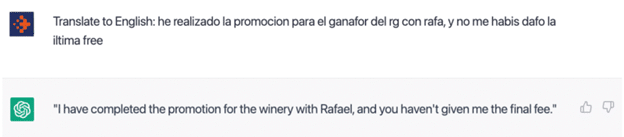
The translation to English was practically incomprehensible. ChatGPT mistranslated the typos and the English word and failed to translate the jargon and acronym at all. Though it did correctly translate the tennis player’s nickname to his full name, the rest of the message was confusing nonsense that accomplished nothing aside from exasperating everyone involved.
This example shows that NLP-powered translation still has a long way to go in a business setting. To achieve a coherent translation, it’s critical to provide the platform with additional context. Without it, the tool won’t know what to do with the industry terms and misspelled words that are commonplace in customers’ communications, and this translation trouble will cause problems for customer service representatives just trying to do their jobs. Counting on a translation method that doesn’t take context into account will only lead to misunderstandings and frustration for both the customer and the representative.
Impact of generative AI in customer service
Generative AI is only as good as the data it’s trained on, and ChatGPT is trained on data from the public internet. This brings up several issues, including the following:
- Biases and inaccuracies: The internet is full of misinformation and prejudicial content, and ChatGPT is unable to discern fact from fiction and objective observations from subjective ones. This means that ChatGPT inherits the biases and inaccuracies present in its data set and subsequently outputs that incorrect information as though it were true. That inaccurate output may result in some confusion, but it could also lead to damaging falsehoods with serious implications.
- Security: Enterprises have concerns about the security of personal or otherwise sensitive data that passes through LLMs like ChatGPT. Even with secure versions, ChatGPT retains content after the completion of the conversation, which raises privacy concerns about data security.
- Gated content: LLMs face limitations when it comes to gated content, which is information that isn’t accessible to the public without some kind of input from them, like filling out a form or entering a password. This means that any information that is behind an internet "gate" is not part of ChatGPT’s knowledge base, so it won’t have the answers to many specific customer questions that reference inaccessible content.
These problems inherent in the current iterations of LLMs will likely be resolved in the next several years as the platforms are developed further, but what about now? How can organizations leverage machine translation to achieve accurate outputs? The answer is context.
As demonstrated, many of ChatGPT’s issues stem from a lack of context. Rather than leaving a generative AI tool to its own devices to interpret and translate text, businesses looking for a real-time translation solution will be better served by a technology that utilizes a glossary of industry- and brand-specific terminology. Layering such technology on top of ChatGPT or other generative AI tools’ outputs will help bring context to that content when translating, as it will enable the proper translation of commonly problematic queries such as slang, jargon, and misspellings. Neglecting to implement this context piece will lead to disjointed conversations and unhappy customers.
Despite their reputation as sophisticated, cutting-edge tools, ChatGPT and other similar LLM-based technologies are not yet able to consistently output accurate translations for businesses. High-quality translations can only be achieved if businesses layer a contextualizing technology on top of ChatGPT. Investing in such a technology is more cost-effective than increasing headcount and will lead to better multilingual support and, ultimately, higher customer satisfaction.
Photo Credit: Aysezgicmeli / Shutterstock
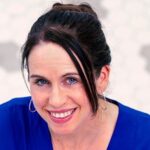
Heather Shoemaker, founder of Language I/O. Heather is the mastermind behind Language I/O’s core technology, which eliminates the need for expensive and time-consuming neural machine translation engine training by dynamically selecting the NMT engine that will best translate a given piece of content and imposing company-specific terminology onto any of the many NMT engines integrated into the Language I/O cloud solution. Prior to co-founding Language I/O, Heather was well-known for globalizing code for Fortune 500s. She was also the senior director of Product Management and Globalization for eCollege, which was acquired by Pearson Education during her tenure. While at Pearson/eCollege, Heather and her team built a next-generation, online college education platform, which was launched globally.