If your data isn't real-time, your AI isn't intelligent

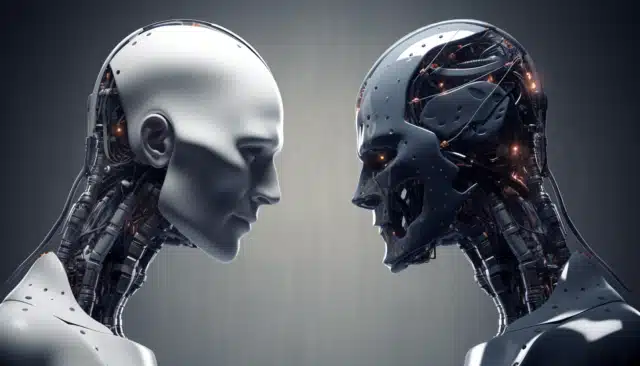
From governments, to entrepreneurs, to enterprises, organizations are looking to understand and exploit Artificial Intelligence to support data-driven decision making. At the heart of this is knowing how AI, and particularly generative AI, operates. Like so much other technology, effective AI is a matter of data analysis.
Put simply, the more data an AI is fed, and the higher quality, the better its analysis will be. This means that the age-old problem of Garbage In, Garbage Out (GIGO) will always be an issue. But there is another, equally crucial issue to consider -- timeliness.
No country for old news
Enterprises understand the issue of GIGO -- feeding an AI bad data will only ever result in bad advice and bad decisions. However, AI -- especially large language models (LLMs) -- is also about the now. It works best when given the freshest, most up-to-the-minute data. Even a delay of seconds can result in out-of-date information, resulting in misguided analysis, especially in industries like finance where milliseconds can make the difference between a winning stock pick and a disaster.
The risk of out-of-date information isn’t new: anyone who has tried to predict a train journey when weather is disrupting services; update health insurance based on years-old medical records; or received online recommendations for products they bought days or weeks ago has already experienced the consequences.
Truth or consequences
For AI, the greatest risk of non-real-time data is creating hallucinations: presenting users with conclusions and advice based on scenarios that are, at best, no longer true -- or worse yet, never were. The result is the same as a desert mirage: the end user believes they are seeing fresh water, or useful insights, but ends up drinking sand.
It’s important to note that this isn’t usually the fault of the AI’s calculations -- it’s offering the best possible analysis based on data that it believes is true. However, circumstances will change so quickly, and businesses make decisions so rapidly, that the slightest deviation from the truth can have major consequences.
This can easily become a life-or-death situation: such as healthcare or disaster response applications triaging treatment based on patients or regions that are no longer in need. Even outside this, businesses can easily miss opportunities because the AI didn’t know they existed; or lose customer engagement because the AI was making recommendations that no longer met their needs.
The consequences go beyond immediate impacts. Over time, organizations will lose trust in their AI: losing the value of any investment and putting more pressure on employees to try and recreate, or review, its findings.
Welcome to the desert of the real-time
To avoid hallucinations, enterprises need to integrate real-time data into the AI process. Naturally, this begins at the point data is first created. How will it be recorded and stored? Can the organization trust the data, or does it need to be vetted -- and if so, how quickly can this be done? Are there any security or privacy risks in creating and using this data -- and if so, how does the organization meet its responsibilities? The answers to this will depend on exactly what data the organization is using, but inevitably will demand that the right processes and technologies are in place to proceed with confidence.
The next question is how fast the organization, and its AI, can process and use the data. The fastest and simplest way – i.e. ensuring data is only ever created, stored and interrogated in one rigid, structured way -- does not support the agility and creativity that should be the hallmark of generative AI. Instead, organizations need to ensure their database can effectively store, manage and query unstructured data; includes a long-term memory layer that can match real-time data with contextual findings; and can search for data in a way that matches LLM’s needs.
Finally, the organization needs to ensure it’s database architecture meets its needs. Given the high demands and scalability of AI, a cloud-based database is a common choice. But in this case, the organization needs to be certain it has control over its data, and its costs -- as the very nature of generative AI means usage can very quickly spiral out of control if not monitored.
Piercing the hallucinations
We have all heard dystopian and utopian predictions about AI. The one thing these have in common is that, whichever side they land on, they involve a large amount of hyperbole.
Ultimately, generative AI is a tool like any other, and can be used for any purpose. But to realise its full value, it has to be built and used in the right way. Real-time data is a crucial piece of the puzzle. Without the right approach to managing and querying data, enterprises risk spending their time chasing phantoms instead of benefiting from insights.
Chris Harris is VP Global Field Engineering, Couchbase.