Five ways AI can boost your data backups

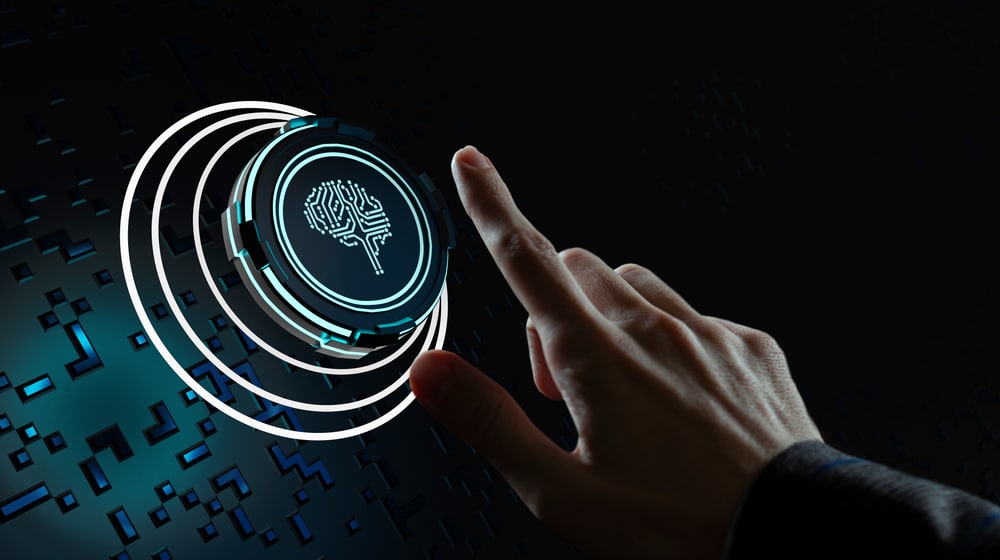
In the event of a data emergency -- say, a cyber attack or a natural disaster shutting down a data center -- no organization wants to worry about whether they have secure, up-to-date backups, and whether they can be easily recovered.
But without the help of artificial intelligence (AI) or machine learning (ML), fewer organizations are able to protect their backups against attacks, meet the necessary backup workload, or meet the defined service level agreements (SLAs) for the availability of information, applications, and infrastructures (or do so quickly and recover efficiently).
AI and ML are already indispensable technologies. They enable us to evaluate data from backup history, derive models for more efficient backup and recovery, and therefore help organizations make better predictions of data security events - from hardware failure and natural disasters to a successful cyber attack on the backups. In the event of an emergency, AI and ML also support the fastest and most efficient restoration of functioning infrastructure and data to get back to business as usual.
AI isn’t just a fun new tool -- it’s become a critical necessity to stay ahead of sophisticated cybercriminals and thereby a vital asset in supporting corporate memory. Here are five ways in which IT teams can benefit from this innovative technology in regards to their backups.
1 -- Scheduling automated routine tasks
Traditional backup plans rely on static rules and schedules, which often leads to complex configurations and inefficiencies, such as suboptimal job runtimes, excessive job wait times, or an exceeded backup window.
By using time series-based ML to predict job run times, AI and ML-powered data management platforms constantly improve the job calendar through optimal sequencing. Cyber-resilient data protection platforms calculate best possible recovery point objectives (RPOs) for cyber-resilient data protection and prioritize recovery workloads based on availability targets. At the same time, AI minimizes the time windows necessary for data backup. If desired, all of this can be done fully autonomously, without IT managers having to intervene manually.
2 -- Streamlined management and monitoring
AI continuously collects performance data from various backup operations to granularly analyze the status of thousands of daily jobs. It identifies anomalies that deviate from the normal security process and can classify them according to type, frequency, and severity. Some errors may be temporary or routine and can be resolved without immediate human intervention. However, other events require the attention of the IT team. Without filtering these critical errors, they often remain hidden longer than desired. For events that require human intervention, the IT team receives relevant filtered alarms so that they can act quickly.
3 -- Data classification and risk analysis
AI and ML also help define and classify information and determine which data should be restored with priority in the event of a disaster. Classification models are trained using the company's own data and identify the document types that are particularly relevant in a business area due to access frequency, for example. In order to accelerate this learning, IT teams can also provide the AI with particularly representative data as an example so that it can derive the relevant models from it. Deep learning and text analysis reliably classify even the most complex, unstructured data.
Personally sensitive information can also be searched for using key terms and classified into different security levels. This leads into risk analysis, which determines the level of threat of information based on context and metadata.
4 -- Attack detection
Data backup simply does not work without cybersecurity. Professional ransomware actors not only attack the productive data, but also the backup files. AI must therefore be able to interpret data anomalies as indicators of an attack. For example, analyzing the entropy of a file is an indication of a compromised file. In an emergency, it is important to detect such anomalies immediately in the moment of encryption. This is not possible for a human observer given the number of events in a set of data, but AI can do this effortlessly.
5 -- Data and infrastructure recovery
Lastly, with AI and ML, IT teams can define optimal recovery time objectives (RTOs) and RPOs with minimal information loss and rapid re-availability, and they receive alerts when predefined SLAs on data availability may no longer be met. AI also helps to define the necessary recovery steps in advance of a disaster. A clean, malware-free recovery in a cloud cleanroom benefits from AI and ML-powered definition of the last clean backup in a dataset.
The future of AI is here
What was once a far-off dream is now today’s reality -- organizations can do more with AI and ML than ever before, and even the capabilities we see now are advancing every day. These technologies are a gamechanger when it comes to backups: they protect against cyberattacks, help to automate routine tasks, improve the efficiency of systems, and ultimately reduce a company's technical debt, for example through improved maintenance or timely and efficient updates.
It's time to recognize that AI can support your whole organization, and not just in the ways that seem the most obvious. While backups might be one element of IT that we tend to set up and then forget about, it’s well worth considering the benefits that this technology could bring to this vital part of the data lifecycle.
Photo Credit: Photon photo/Shutterstock
Ian Wood is Senior SE Director at Commvault.