Gen AI's pitfalls and why the business impact remains stagnant

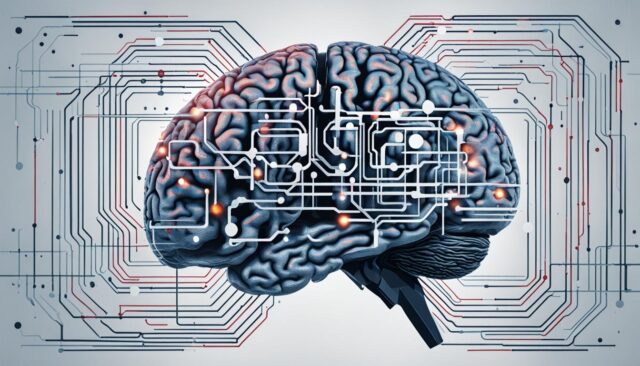
No technology, especially in its early adoption phase, is without fault. Even with the popularity generative AI has gained in both the eyes of businesses and consumers alike, its imperfections cannot be glossed over. Hallucinations and biases in training data, among other issues, are leading business owners to hesitate when considering adoption.
While some early adopters have found ways to adopt large language models (LLMs) as they exist today, many feel they are left with essentially two options. Wait until improvements come or governmental guidelines are put in place to ensure the safe use of the technology, potentially being left behind, or adopt now and without letting AI touch business-critical systems. Neither of these options is truly viable, so where can businesses go from here? Diving below the surface coverage of gen AI to understand both its pros and cons will help modern businesses to determine where they can safely implement LLMs tomorrow.
What Are Gen AI’s Pitfalls?
Each iteration of today’s most popular chatbots is built off a vastly larger data set, delivering a more capable solution with each evolution. However, given the black-box nature of generative AI, the characteristics of this technology change as the underlying dataset evolves as well. A recent update to ChatGPT addressed concerns around “lazy” and “weird” response behaviors users had started flagging, with Open.AI citing that one potential reason being ChatGPT’s reinforcement learning process.
While AI developers are still fine-tuning the auditing process, to better understand how and why generative AI programs act the way they do, hallucinations by these programs will require human monitors to act as quality assurance checks. For businesses, keeping humans in the loop may seem like a pitfall, as they’re not able to fully hand off a process AI. In reality, these checks should remain a part of the design process.
Business Leaders are Interested and Investing in AI, but Implementation Hasn’t Quite Caught Up
Providing world-class customer service, especially in the digital age, is top of mind for all business leaders. Building a standout customer journey means you must be everywhere, from mobile to social media. While headlines have started shifting customer expectations around what top-tier customer service should look like, business leaders know that even the most advanced AI available today isn’t the dream customer support agent of tomorrow. One of the biggest factors preventing enterprises from bringing AI fully into the fold is trust. Developers have noticed and are starting to react by showing transparency around their guardrails and responsible AI practices, but the implementation gap will still take some time to catch up.
The Impact of Gen AI on Businesses is Likely to Change Quickly and For a Long Time
In the short term, added attention by developers, businesses, and government regulators will shine a heavy focus on responsible AI development. By building technical guardrails and enforcing regulations that help to ensure safe and secure use of Gen AI at an industry level, enterprises looking to integrate AI can ease worries. That said, looking towards the future, there is little evidence that the innovation curve of generative AI technology has peaked or is slowing down. By continually expanding the training data on which these tools are developed, we’re only going to see the advent of more accurate, and safe, platforms for businesses. The only question remaining for businesses? How much evidence is necessary to make them feel secure that it’s time to implement AI, and where can it make the biggest difference?
Finding the Balance Between Live and Virtual Team Members
Gen AI will see a need to be overseen by human teams well into the foreseeable future, and likely forever, but that does not mean there aren’t business use cases that can benefit from it today. Using LLMs to generate content for existing virtual agents is one example, allowing businesses to get chatbots up and running in a faster timeframe without increasing risk to the business. Using LLM's is like brewing a potent potion: each ingredient adds to its power, but the wrong combination can be disastrous, make sure you have the right tools needed to mix your potion.
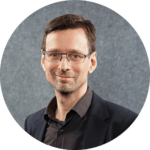
Rasmus Hauch is CTO at Boost.ai. Rasmus brings a rich background of technological leadership to boost.ai as Chief Technology Officer. Previously, he was the CTO at 2021.AI, leading teams to deliver top-notch AI/ML solutions. His advisory roles at Proprty.ai, Ryver.ai, and Capsule, along with a long tenure at IBM, reflect his broad expertise in the AI domain. At boost.ai, Rasmus is geared to further our technological front, aligning with our mission to innovate in conversational AI.