How collaborative learning and conversational intelligence are changing AIOps [Q&A]

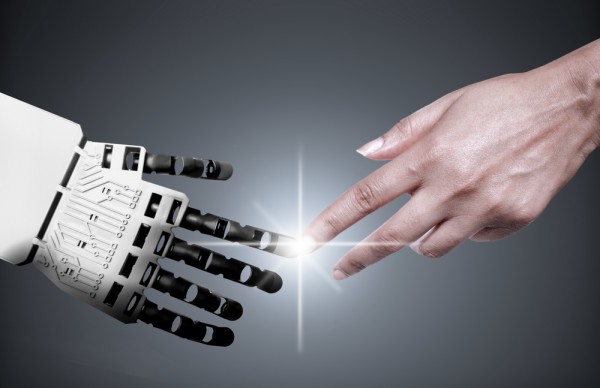
Artificial intelligence is changing the way that we work with computers and in particular collaborative learning (CL) and conversational intelligence (CI) are set to reshape AI-powered operations.
We talked to Dr. Maitreya Natu, chief data scientist at Digitate, to discover more about what this means both for businesses and for the role of operations professionals.
BN: What is Collaborative Learning (CL)?
MN: AI solutions seek human expertise from time to time to improve its effectiveness and trustworthiness. Collaborative learning is an interaction paradigm where the AI solutions bring human-in-the-loop (HITL) to, for example, verify the data-driven insights, get guidance on exceptions, and augment its knowledge with human intuition and insight.
BN: What are some the common use cases where CL is found to be very useful?
MN: There are a number use cases where CL provides value. Examples include the following:
- An AI solution mines observations and insights. CL can bring human-in-the-loop to help translate them into actionable recommendations.
- An AI solution performs auto-triage and resolution. CL can bring human-in-the-loop to handle exceptions and unknown conditions.
- An AI solution can automate repetitive tasks. CL can bring human-in-the-loop to 'derisk' them by expert validation and corrections. • An AI solution generates insights. CL can bring human-in-the-loop to review/validate/approve insights for proactive planning.
Let's take an example of CL's auto-triage and resolution: An AI solution mines data for context, finds the cause of a problem and recommends a fix. CL enables human experts to review and approve the fix or reject and recommend an alternative fix. CL also enables a human expert to become the handler of exceptions. Through CL, the AI solution learns from this interaction and forms its own point of view as it sees more and more cases. This increases the overall coverage, effectiveness, 'explainability' and trustworthiness of auto-triage and resolution.
BN: What are the benefits of CL?
MN: CL helps the AI solutions to become smarter as these solutions gain a better understanding of the system, the data, and the domain. CL can also compensate in situations where data is incomplete and inconsistent and thus expands the reach of the AI solutions. CL makes the AI engines trustworthy by bringing in training and validation by a human expert.
BN: Which design principles should be adhered to while implementing CL?
MN: While offering a wealth of benefits, CL can present challenges, for example, inundating the human expert with too many questions, and the potential over-reliance on human expertise. Hence, it is important to adhere to two key principles while implementing CL:
- Ask the right questions at the right time. Make as much inference as possible from the available data, the enterprise context, and by extrapolating from similar conversations in the past, and engage humans only for the missing pieces of information.
- Assess when to trust human intuition and when to trust data-driven insights, by assessing the insights on the basis of factors such as support, confidence, recency, and consistency.
BN: What is Conversational Intelligence (CI)?
MN: Conversational Intelligence is an interaction paradigm where humans can engage in intelligent conversations with machines. Historically, when we think CI, we perhaps think of chat-bots that enable interactions such as Q&As. Increasingly sophisticated solutions started bringing the flavor of sentiment analysis into conversations. Recent advances have made CI much more a way to engage with humans in intelligent conversations, which goes beyond just answering questions. For example, CI should:
- Ask just the right questions.
- Learn 'between the lines' and interpolate.
- Form a point of view.
- Should lead the conversation.
- Should learn and adapt to user responses.
BN: What are some the common use cases where CI is found to be very useful?
MN: CI can very effectively address 'insight fatigue,' as users are able to easily find areas that need attention and insights of interest through simple conversations. CI an also be a very effective tool in bringing 'explainability' and trustworthiness to the AI-driven insights.
Let's take an example: a head of a line-of-business interacts with a CI engine and asks "What are the areas that need my attention?" The CI engine understands the user, their estate, their past preferences, and business criticality, then forms a point of view and provides insights into the areas that require attention. The CI engine then can lead the conversation and help prioritize these areas, provide additional details, and recommend corrective actions. The CI engine should also adapt to the conversation, for instance, if the user is assessing an application’s poor performance, then the CI engine can proactively provide the root-cause analysis, or find similar issues faced in other applications, and so on. The CI engine continuously expands its understanding with each user conversation and how the users are responding to the responses.
BN: What are the benefits of CI?
MN: CI empowers the user of an AI solution to make the most of AI-driven insights and makes those insights explainable and easy to access. Consequently, CI enables an increase in the adoption of an AI solution by the business teams.
BN: Which design principles should be adhered to while implementing CI?
MN: There are two main principles to factor in when implementing CI:
1. Engage in intelligent conversations with humans. This can be done by capturing the conversation context, forming a point of view, leading the conversation, and adapting the conversation with user responses.
2. Bring 'explainability' into the AI insights using the concepts of explainable intelligence*. Bring textual and visual evidence of the reasoning process of the AI engine.
*Explainable AI encompasses a range of methods, principles, and procedures aimed at aiding both creators and users of artificial intelligence models in comprehending the decision-making process of these models.
BN: How the convergence of CL and CI will bring about transformative changes in AIOps for informed decision-making.
MN: The convergence of CL and CI help create new metaphors of augmented intelligence.
CL helps make AIOps solutions become smarter. AIOps solutions understand the system, the data, and the factual/situational/operational knowledge. Through CL, AIOps solutions are able to 'connect the dots,' fill in the missing pieces, be trained, and get validated. CI helps humans make the most of AI-powered insights, making them explainable and easy to consume, which leads to an increase in the adoption of AIOps. Together, CI and CL help head towards conversational, explainable, trustworthy, and operational intelligence.
Image credit: Pixelbliss/Shutterstock