The impact of generative AI cybersecurity [Q&A]

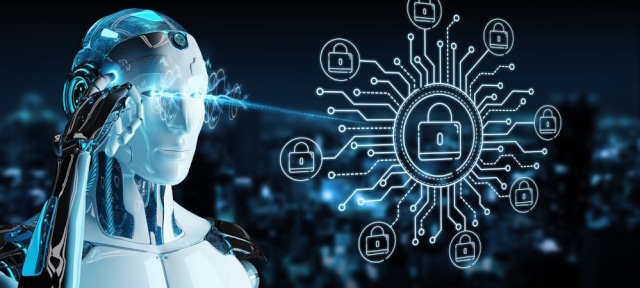
Since ChatGPT's launch in 2022, there's been an explosion of speculative use cases for generative AI in the workforce -- and concern from the cybersecurity community over an unproven, unvetted, and potentially powerful new tool.
How have those concerns played out in the real world? We sat down with Nick Hyatt, director of threat intelligence at Blackpoint Cyber, to hear about the reality of generative AI's risk to the modern workplace.
BN: How has generative AI affected the cybersecurity field?
NH: Generative AI (GenAI) has definitely impacted cybersecurity, but its greatest impacts won't be felt for a while.
Consider the difference between using GenAI for information retrieval versus possible threat detection.
When a SOC analyst triages a potential security incident, they could ask a GenAI chatbot what certain activity log tags mean. The GenAI will return a straightforward answer to their question, though the analyst should double-check the response's accuracy before acting.
Now, the analyst can spend more time on making critical connections between activity and threats, rather than rote information gathering.
On the other hand, if the analyst asks that same GenAI to interpret the log activity itself -- that is, for the GenAI to determine if the input activity was benign or malicious -- the resulting output would not be nearly as useful or reliable.
BN: Why can't generative AI do the same evaluation as a human analyst?
NH: It comes down to a lack of context.
GenAI chatbots and LLMs receive inputs ('prompts' and questions from our example user-analyst) that are completely divorced from the wider managed environment, making responses equally divorced from the real situation.
Environmental contextualization -- that human analysts process and use to analyze potential threat activity -- is simply not present in the average GenAI prompt-query. Current GenAI models also aren't trained on the sort of language or context that would help their chatbots ask users for (or otherwise consider) that missing context.
However, this limitation won't always be the case. The next generation of GenAI cybersecurity tools is already in development, using industry-specific data and vocabulary to tailor outputs based on specific security use cases.
Researchers are also designing powerful LLM knowledge base architectures known as 'transformer neural networks' to empower autonomous decisions to resolve analyzed environmental threats -- without an external analyst’s input or prompting.
BN: What are some new threats powered by generative AI?
NH: GenAI isn't doing anything that hasn't been done before by experienced threat actors. But, they are providing cheaper capabilities to less-skilled adversaries -- and speeding up experienced foes' attack cycles.
For example, GenAI enables threat actors to generate, test, and distribute large-scale misinformation operations to manipulate public opinion and threaten democratic processes.
Due to these and similar threats, government agencies and leaders must balance regulatory measures on GenAI tools and capabilities with the preservation of fundamental rights like freedom of speech -- combined with realistic assessments of what’s already out there, regardless of future 'white hat' regulation.
While regions like the EU are advancing their initial regulatory responses, such as the AI Act, other regions lag. We need to come together as a global community to address GenAI-powered threats, particularly misinformation campaigns.
Threat actors still have the same goals, however, and behave the same in compromised environments. Defense-in-depth security strategies with heuristics-based alerting and remediation remain critically effective -- even against GenAI-boosted threats.
BN: What about generative AI's impact on identity theft, in particular?
NH: GenAI has increased identity theft risks -- especially fraud potential in corporate environments.
Imagine that someone in your finance office receives a call 'from' the CEO. The caller ID is right, and the person sounds exactly like your CEO.
Except it's not the CEO calling with new payment instructions; it's a spearphishing attack, augmented by GenAI’s synthesis of the CEO’s voice based on surprisingly few audio samples.
There's just so many open-source tools and widely available public data for threat actors to quickly spin up new versions of old initial intrusion campaigns like this fake CEO spearphishing attempt.
BN: How can security teams secure their users using generative AI tools?
NH: If we look at the current GenAI landscape, it's difficult to anticipate all potential new or expanded attack surfaces, but we can pull out specific areas of concern:
- Before your organization builds an on-premises and proprietary LLM -- or just tries to customize a cloud-based GenAI app! -- it must first establish and enforce data hygiene. Organizations can't just dump an entire server into an LLM and expect things to go swimmingly, especially if they're handling other organizations', clients', or users' data.
- Supply-chain defense just got more complicated, too. What happens if your customers or your vendors are using (or creating) LLMs? What data of yours is being incorporated into their knowledge bases?
- Output validation remains key to effective GenAI workflow integration. Who’s checking your GenAI's outputs?
It's ironic, coming from a threat intelligence guy like me, but I believe that technology won't ultimately answer these questions. Rather, it's the right experienced people and processes that will fix GenAI's problems in the long run.
It means growing pains, for sure, but the outcome will be worth it.
Image credit: sdecoret/depositphotos.com