AI's rapid development is a security disaster waiting to happen

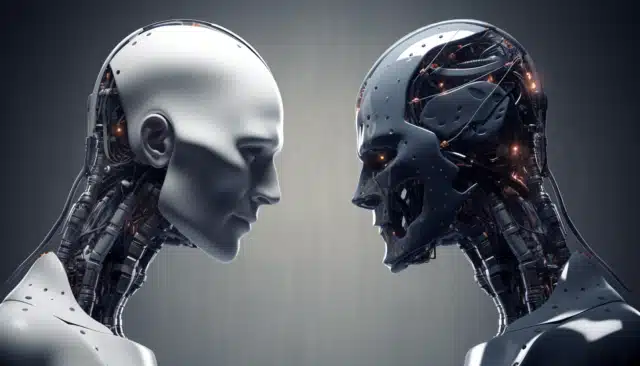
No matter how you look at it, AI is currently booming. The AI market is on track to reach $407 billion by 2027 (compared to $86.9 billion in 2024). Last year, ChatGPT became the fastest-growing consumer application in history when it reached 100 million monthly active users just two months post launch. McKinsey declared 2023 as Generative AI’s breakout year, and a follow-up 2024 survey found that the percentage of organizations using Generative AI jumped from approximately 50 percent to 72 percent from 2023 and 2024. Meanwhile, a culture shift within tech and business has accelerated AI adoption seemingly overnight.
Long before Generative AI entered the scene, tech C-suites were concerned about being left behind. AI’s disruptive potential has only exacerbated this. Companies with the bandwidth to do so are developing their own AI systems or converting existing ones over to AI. Such behavior is motivated primarily by reputation management. No major player wants to look like they were left behind as their competitors innovated to newer heights.
The problem with this reputation-minded AI mania is its flipside: hasty integration and innovation opens the AI to cybersecurity risks that can cause serious financial and reputational damage. This inconvenient fact has been dismissed in the push to get developers to build as fast as possible. The current state of AI is an all-hands-on-deck development scenario where cybersecurity has been left idling. The idea that AI can undergo a significant retooling without changes to its associated security tools and measures is untenable.
People often associate AI with Large Language Models (LLMs) such as ChatGPT. Such technologies, however, are just the latest generation of AI and machine learning (ML) that have existed for years. Powering this shift in technology and perception is the expansion of deep neural networks, from which many LLMs leverage a specific architecture known as a transformer. Transformers and other neural networks are a huge step forward, but they've introduced a new rapidly evolving attack surface that established security processes struggle to defend against.
Cyber-criminals are getting better at using the options that LLM-based AI presents them. The foundation models, such as ChatGPT and Claude, are being incorporated at pace into enterprise applications that are vulnerable to threats spanning jailbreaking, data poisoning, and model extraction. Documents uploaded to LLMs can contain hidden instructions that are executed by connected system components, posing reputational risk challenges for whomever uses the LLM.
This amounts to a major attack against AI systems waiting to happen. In fact, it’s already happening. A bug in an open-source code library used by ChatGPT led to a major data breach last year. Moreover, the established tools and strategies of vulnerability scanning, pentesting, and monitoring fail to match recent advancements within AI. How does one apply security controls to a piece of software that is intrinsically designed to be opaque and random in its behavior?
Despite cyber attacks on AI being as serious as attacks on other types of software, there’s a serious gap in understanding, tools, and skill sets required to address challenges within AI-specific security.
Only cybersecurity tools specifically built for AI security are suited to the task. From pentesting and red teaming to firewalls and data loss prevention, these AI security tools enable organizations to evolve their established cybersecurity tools and methodologies onto the newest wave of disruptive technology. Failure to build resilience and risk management into the very fabric of AI development and operation will result in financial and reputational damage that organizations cannot afford to risk.
Choosing not to integrate LLMs into products and services will place companies behind their competitors. Integrating LLMs, on the other hand, exposes companies to data loss from unmonitored use of third-party GenAI solutions -- if not deployed alongside automated and continuous security testing and real-time threat detection, that is.
34 percent of companies are already investing in AI security tools, which is a promising start. Still, it’s not enough. C-suites must realize that their deployment mania must be accompanied by rational and specific security measures. The future of their companies might just depend on it.
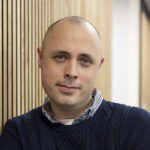
Peter Garraghan is CEO and CTO of London-based cybersecurity startup Mindgard. Professor in Computer Science at Lancaster University, and fellow of the UK Engineering Physical Sciences and Research Council (EPSRC), Peter has dedicated years of scientific and engineering expertise to create bleeding-edge technology to understand and overcome growing threats against AI. Mindgard is a deep-tech startup specialising in cybersecurity for companies working with AI, GenAI and LLMs. Mindgard was founded in 2022 at world-renowned Lancaster University and is now based in London, UK.