How to unlock the power of real-time analytics [Q&A]

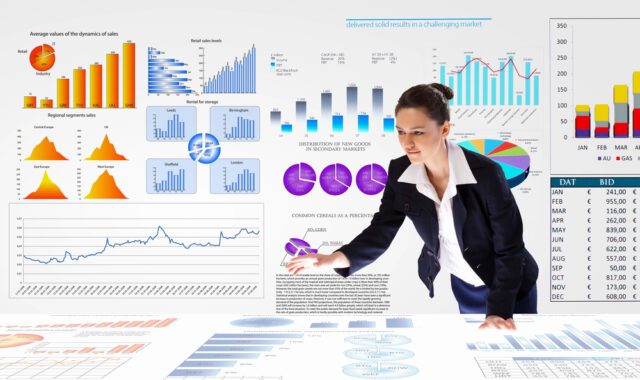
The increased need for real-time analytics is driven by the rise of the on-demand economy, where consumer expectations for immediate access to products, services, and information are transforming how businesses operate and compete.
We spoke to Kishore Gopalakrishna, co-founder and CEO of StarTree, to discuss the need for real-time data capabilities, the strategic utilization of real-time data to enhance operational efficiency and competitiveness, and the essential technology and operational considerations for building a robust analytics infrastructure.
BN: What are some of the primary business dynamics driving the increased need for real-time analytics in today's market, particularly in the on-demand economy?
KG: Modern consumers demand immediate access to products and services, compelling businesses to adapt and provide real-time responsiveness for a competitive edge. This urgency for speed is reshaping whole industries, requiring companies to continuously monitor and analyze data to meet customer needs promptly.
The ability to make data-driven decisions in real time enables companies to optimize their operations, personalize customer interactions, and swiftly address market changes. This agility is crucial for maintaining customer satisfaction and loyalty in an era where delays result in lost business.
A consumer waiting for a ride wants to know exactly, in real-time, where their ride is and how long they need to wait. The rideshare business itself wants to understand how their drivers are meeting customer demand. Plus all parties involved need to be protected from payments fraud.
The integration of advanced technologies such as AI and machine learning into analytics platforms allows for more precise and proactive decision-making. These dynamics collectively underscore the strategic importance of real-time analytics as businesses strive to remain relevant and competitive in the fast-evolving market landscape.
BN: In what ways can companies utilize real-time data as a strategic asset to streamline operations, enhance efficiency, and stay competitive in their respective industries?
KG: Batch-oriented systems are always operating minutes, hours, if not a day or more lagged behind reality. In recent years this simply became untenable, and the shift to real-time accelerated. While data warehouses and lakehouses still serve their traditional roles, real-time analytics systems are where the freshest data is being processed to gain insights in seconds.
Companies can utilize real-time data to monitor supply chains continuously, identifying and addressing bottlenecks or disruptions proactively, minimizing downtime. It also enhances customer experiences through personalized interactions and offers, increasing sales and satisfaction. For example, eCommerce platforms can recommend products based on a customer's current browsing behavior, leading to increased sales and customer satisfaction.
Real-time data enables quick, data-driven decisions, allowing businesses to respond swiftly to market trends and demands. This agility is crucial in dynamic industries. Additionally, real-time analytics support predictive maintenance in manufacturing, reducing equipment failures.
Real-time data fosters innovation by revealing new opportunities, helping businesses pivot and capitalize on emerging trends and markets. In essence, real-time data transforms operations, drives efficiency, and maintains competitiveness.
BN: What technology and operational considerations should businesses prioritize when establishing a robust real-time analytics infrastructure, and what role can tools like Apache Pinot play?
KG: When establishing a robust real-time analytics infrastructure, businesses should prioritize scalability, low-latency data processing, seamless data integration, security, and data quality.
Scalability ensures the infrastructure can handle large data volumes and grow with the business, making cloud solutions and distributed computing frameworks essential. Low-latency processing capabilities are critical for delivering timely insights. Apache Pinot, a real-time distributed OLAP datastore, plays a vital role here, offering sub-second query response times for real-time analytics.
Data integration is another key consideration, requiring systems that can ingest data from various sources efficiently. Apache Pinot integrates smoothly with data streaming systems like Apache Kafka, enabling real-time data ingestion and analysis. It also integrates with stream processing tools like Apache Flink, which can transform and enrich data for deeper insights.
Operationally, businesses should build a skilled team to manage and optimize their analytics infrastructure. Fostering a culture of continuous improvement and innovation ensures the infrastructure evolves with technological advancements and changing business needs. By addressing these considerations and leveraging Apache Pinot, businesses can create a resilient and efficient real-time analytics infrastructure.
BN: How does observability play a role in enhancing real-time analytics, and what are the key benefits of adopting a disaggregated observability stack over a unified platform?
KG: There’re two parts here. The first is the observability of your real-time analytics stack itself, making sure it is operating at peak efficiency. Apache Pinot provides such metrics right out of the box to monitor every aspect of performance and robustness of your production clusters -- gauges, meters and timers.
The other is using real-time analytics in a disaggregated observability stack to provide real-time insights into your overall operations and infrastructure.
That's what Cisco did, integrating Pinot for analytics with Grafana for visualization. Adopting a disaggregated observability stack, as opposed to a unified platform, offers several key benefits. It allows for greater flexibility, enabling businesses to choose the best-in-class tools for specific observability tasks such as logging, metrics, and tracing. This tailored approach ensures optimal performance and adaptability to different use cases. A disaggregated stack also enhances scalability, allowing components to be scaled independently based on demand. Additionally, it provides better fault isolation, where issues in one component do not affect the entire system, leading to increased resilience and uptime.
Overall, observability and a disaggregated stack together ensure robust, efficient, and reliable real-time analytics.
BN: What role does anomaly detection play, and how can businesses leverage this capability to identify and address potential issues proactively?
KG: Anomaly detection is pivotal in real-time analytics as it identifies deviations from normal patterns in data, signaling potential issues that require immediate attention. By leveraging this capability, businesses can proactively detect irregularities such as fraud, system failures, or unusual customer behavior, allowing for swift intervention before these anomalies escalate into significant problems.
Real-time anomaly detection enables continuous monitoring and instant alerts, ensuring that businesses can respond to issues promptly, minimizing downtime and reducing operational risks. Additionally, it aids in maintaining data integrity and accuracy, as anomalies often indicate data quality issues.
By integrating anomaly detection into their real-time analytics infrastructure, businesses enhance their ability to maintain smooth operations, improve decision-making, and sustain a high level of service quality.
Image credit: SergeyNivens/depositphotos.com