Uncovering GenAI's unsung heroes [Q&A]

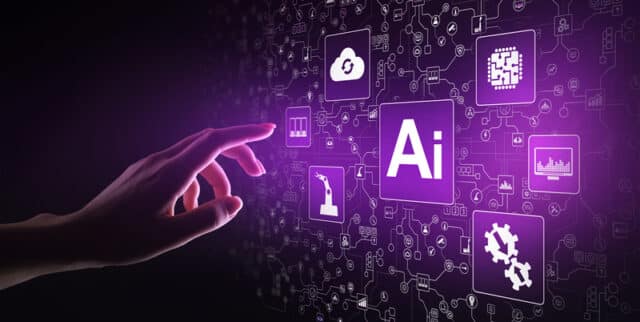
There's no doubt that AI is seen as flavor of the month across many sectors at the moment. But how much of this is hype and how much genuine value?
We spoke to Martin Hawksey, collaboration engineer at Qodea, to discuss GenAI and the areas where GenAI is making a real difference, some of which you may not be aware of.
BN: There's been a lot of hype around AI, but what are the more practical ways that companies are using GenAI that is making a difference today?
MH: From the development of new lifesaving drugs, to making manufacturing processes more efficient, GenAI is already helping to make a real difference for ordinary people. Often overlooked, however, are some of the low-key, yet vital, impacts that AI is having across many different sectors of the economy. GenAI's capabilities lend themselves to a huge variety of everyday use cases that can take away the pain of traditionally laborious and costly tasks. In the coming months and years, it will help us to do things that were previously impossible.
BN: Can you give us any examples?
MH: Chatbot testing is a good example. When we think of testing in a normal application environment, there is a clear set of functions to test to make sure the application is working as it should. With a chatbot, this isn't the case. There could be thousands of questions that someone might ask it -- how do you test for all those? The reality is many businesses can't do this manually, so just a fraction of questions and service tickets end up tested -- which is perhaps one of the reasons we are seeing so many 'rogue' chatbots around. And with 35 percent of customers feeling that chatbots are bad at customer service, businesses should be doing all they can to improve this experience.
By using GenAI to simulate a wide range of user interactions, creating diverse personas, each with unique conversational styles and queries then businesses can start to close those test coverage gaps. They can bombard the chatbot with queries and tickets, in which an LLM checks responses, forming a feedback loop for qualitative and quantitative analysis.
We have found that the accuracy is generally very high -- helping businesses to increase test coverage at high speed and low cost. Businesses can also add a firewall to the application to create a boundary for what it can and can't talk about. This ensures it won't answer questions outside of its domain -- helping to reduce risk even further.
BN: You mention AI generated synthetic data and personas; can you give us some examples of where this can be useful (beyond testing)?
MH: AI generated synthetic data and personas can be used to tap into new data potential for businesses. Often, businesses will build models but find they can't make use of the data. In fact, 73 percent of businesses are facing challenges in data use, hindering the advance of IT projects. COVID had a big part to play here -- creating anomalies in data, especially in industries like travel and leisure. But LLM's can synthesise existing data, identify gaps and predict how best to fill them. By doing so, LLMs help businesses unlock previously undiscovered insights essential for informed decision-making.
Meanwhile, in the medical field, the scarcity of data on rare conditions, such as specific types of brain tumours or early stages of certain diseases, poses a significant challenge. LLMs can synthesise existing data, spot where gaps exist and aid anomaly prediction, which can be used to train other LLMs. This innovative application of GenAI has the potential to revolutionise medical diagnostics and treatment planning.
AI created synthetic data can also be used for market intelligence and research -- useful for checking a pulse on an issue. Synthetic data can be used to model different market scenarios, helping businesses forecast potential outcomes and make data-driven decisions. For example, a company looking to launch a new product can use GenAI to simulate consumer reactions, refine their marketing strategies, and anticipate potential challenges. This predictive capability allows for more informed decision-making, reducing the risks associated with new ventures.
BN: What are the potential draw backs of using AI generated synthetic data?
MH: While creating LLMs to devise questions and create personas is a very helpful use case -- particularly in testing -- if you are looking for true market intelligence then this model is fraught with issues. Ultimately, businesses need to train the model and create the personas, so the prompts they use will be inherently biased. It's a bit like filling a focus group with a group of actors – you'd be leading the witness, so how much genuine insight you could glean is questionable.
What's more, the LLM will be trained on information on the internet -- which is usually very polarised. If you're conducting market research on a new development in a town, are you really going to get a true picture of how the community feels by looking at what the online sentiment is? Or will you hear a groundswell of people who are dissatisfied? People on the internet only tend to comment on things if they feel strongly one way or another, which makes for an environment with little nuance.
The danger is that you will just create echo chambers. And if the research then gets published this effect will only be amplified, as the LLM uses the research to create further personas, reinforcing the messages and amplifying the echo effect. The question then becomes: how do you test it? How do you assess the validity of the outputs? Without careful validation and triangulation with real-world data and human judgment, relying solely on LLM-generated insights can lead to misleading conclusions and reinforce existing biases.
BN: What advice would you give to companies looking to use AI generated synthetic data?
MH: My main piece of advice would be to make sure you have safeguards in place. Clearly outline what you aim to achieve with synthetic data and recognise that -- whilst useful -- synthetic data will not perfectly replicate all the nuances of real-world data. Whilst LLMs can make assumptions and informed predictions, they are not fact. This is why it’s important to validate the performance models with real-world data, to ensure they remain accurate and reliable. This way, companies can leverage AI-generated synthetic data to enhance their models and systems, while maintaining high standards of quality and accuracy.
Image Credit: Wrightstudio/Dreamstime.com