AI degradation -- what is it and how do we address it? [Q&A]

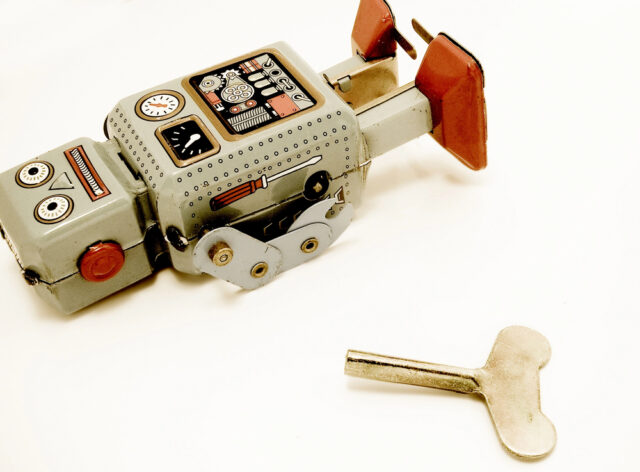
Many in the industry believe that AI is degrading because it's being starved of human-generated data. This leads to models being trained on the output of older models which increases the risk of hallucinations and errors.
But how big an issue is this and what can we do to fix it? We spoke to Persona CEO and co-founder, Rick Song to find out.
BN: Could you elaborate on how the shift to synthetic data is impacting AI models and the potential dangers it poses?
RS: AI models thrive on human-generated data, which provides the rich context, nuance, and diversity necessary for effective learning. However, as organizations increasingly turn to synthetic data to feed these models, we're crossing into dangerous territory.
When AI models are trained on outputs generated by previous iterations, they tend to propagate errors and introduce noise, leading to a decline in output quality. This recursive process, known as 'Model Collapse' or 'Model Autophagy Disorder' (MAD), causes AI to drift further from human-like understanding, which not only undermines performance but also raises critical concerns about the long-term viability of relying on self-generated data for continued AI development.
And the consequences are far more dangerous than just sub-par results -- AI's degradation could lead to serious risks, such as medical misdiagnosis, financial losses, and even life-threatening accidents.
BN: What exactly is 'Model Collapse' and why is it a significant concern for the future of AI?
RS: Model collapse is the degenerative process in which AI systems progressively lose their grasp on the true underlying data distribution they're meant to model.
The more AI-generated content spreads online, the faster it will infiltrate datasets and, subsequently, the models themselves. And it's happening at an accelerated rate, making it increasingly difficult for developers to filter out anything that is not pure, human-created training data. The fact is, using synthetic content in training can trigger Model Collapse or MAD.
Model collapse is the degenerative process in which AI systems progressively lose their grasp on the true underlying data distribution they're meant to model. This often occurs when AI is trained recursively on content it generated, leading to a number of issues:
- Loss of Nuance: Models begin to forget outlier data or less-represented information, crucial for a comprehensive understanding of any dataset.
- Reduced Diversity: There is a noticeable decrease in the diversity and quality of the outputs produced by the models.
- Amplification of Biases: Existing biases, particularly against marginalized groups, may be exacerbated as the model overlooks the nuanced data that could mitigate these biases.
- Generation of Nonsensical Outputs: Over time, models may start producing outputs that are completely unrelated or nonsensical.
A case in point: a study published in Nature highlighted the rapid degeneration of language models trained recursively on AI-generated text. By the ninth iteration, these models were found to be producing entirely irrelevant and nonsensical content, demonstrating the rapid decline in data quality and model utility.
BN: You've identified the risks associated with AI degradation, particularly regarding the use of synthetic data. Who should be held responsible for addressing these issues, and what steps should be taken?
RS: The responsibility for addressing AI degradation lies significantly with online giants, the gatekeepers of vast digital spaces where content is created and spread. These platforms have allowed low-quality, AI-generated data to flood their ecosystems, prioritizing engagement and growth over the authenticity of content. As a result, they have fueled the very issues that now threaten the integrity of AI.
To combat this, there will be a massive push for verification from social media companies and other digital platforms. But that alone won't filter out all inauthentic content. What platforms can do is pick up on patterns of people that frequently use AI to post. And if they do, the platforms can place restrictions or ban these users.
BN: Beyond the responsibilities of online giants, what role can individuals play in combating the degradation of AI?
RS: Individuals also have a crucial role to play in combating AI degradation. We need to demand transparency from digital platforms, hold companies accountable for the content they host, and push for stronger verification processes. It's not just about relying on these companies to fix the problem; we, as users and consumers, must take a stand.
By supporting ethical AI practices, advocating for responsible regulation, and practicing digital literacy, we can help steer AI development in a direction that benefits society. Ensuring that the content we engage with is authentic, that our data is protected, and that AI is developed in a way that serves humanity is a collective responsibility.
BN: What are the broader implications if we fail to address the issue of AI degradation, and how can we mitigate these risks?
RS: If we fail to address AI degradation, the consequences could be both far-reaching and severe. As AI models continue to degrade, we risk a breakdown in reality, identity, and data authenticity. Another major implication is that AI development could completely stall, leaving AI systems unable to ingest new data and essentially becoming 'stuck in time.' This stagnation would not only hinder progress but also trap AI in a cycle of diminishing returns, with potentially catastrophic effects on technology and society.
The ripple effects could undermine trust in technology and create significant challenges in fields that rely heavily on precise data and AI-driven insights. To mitigate these risks, we must reduce the reliance on synthetic data, ensure more human-generated input, and push for robust verification processes to maintain the integrity of AI. By doing so, we can preserve the benefits of AI while safeguarding against the dangers of its potential degradation.
Image credit: davincidig/depositphotos.com