Building trust in telemetry data [Q&A]

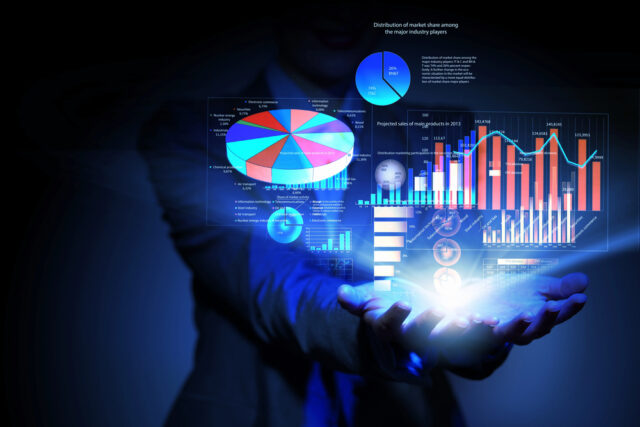
With the increasing importance of observability in digital operations, businesses need to ensure the reliability and relevance of their telemetry data in order to maintain system and application performance, debug, troubleshoot, respond to incidents and keep their systems secure.
We spoke to Tucker Callaway, CEO of Mezmo, to discuss the strategic considerations and concerns enterprises face in managing and optimizing their telemetry data.
BN: Why has telemetry data become so important?
TC: In the last five years, we have seen an accelerated shift to digital business and operations, which has led to a surge in telemetry data generated from those operations. Telemetry data includes metrics, events, logs, and traces from various systems, applications, and services. This data stream offers insights into application performance, infrastructure reliability, user experience, service interactions, and potential security threats.
As businesses move to the cloud and scale their digital infrastructures, the volume and complexity of telemetry data increase disproportionately to the value enterprises get from it. This discrepancy poses a critical problem, particularly for observability. Telemetry data is dynamic, continuously growing, and constantly changing with sporadic spikes. Enterprises struggle to confidently deliver the right data to the right systems for the right user, uncertain about data content, value, completeness, and ensuring that sensitive Personally Identifiable Information (PII) is handled properly, putting them at risk. These factors reduce trust in collected and distributed data. The organizations must change how they manage and extract value from telemetry data. Telemetry pipelines, systems designed to collect, process, and transmit telemetry data, can manage this dynamic and expansive data stream to improve operational efficiency and ensure better data utilization for increased value.
BN: How can enterprises make sure they enhance the usefulness of their telemetry data while keeping costs under control?
TC: Telemetry data is an enterprise asset and must be treated better than mere exhaust from your systems. To ensure telemetry data delivers value cost-effectively, enterprises need to adopt a well-defined system that supports cost-effectively understanding, optimizing, and responding to telemetry data. Incorporating data engineering principles into your telemetry data management helps you get there. For data understanding, enterprises profile and analyze telemetry data to identify patterns and detect anomalies to validate data quality and segregate useful data from redundant repeated data.
Once you understand the data, you can start separating the signal from the noise using various optimization techniques. Optimization focuses on reducing noisy data that incurs higher costs. Telemetry pipelines can reduce data volume by 70 percent by selectively filtering, routing, and transforming data. Moreover, you can reformat, transform, and enrich your data to confirm it is ready for downstream system consumption and analysis. These steps ensure that the data exiting the pipeline is accurate, in the right format, and relevant, helping to avoid cost overruns while maintaining data quality. Intelligent routing rules enhance efficiency by directing critical data to high-performance systems and less critical data to low-cost storage solutions. It’s also essential to determine when to rehydrate data and adjust sampling rates based on the operational context -- whether during normal operations or heightened threat environments.
The responding phase utilizes telemetry pipelines to alert about data aberrations and adapt to incidents and changing conditions. As pipelines detect any deviations in data as the data moves through them, alerts can inform users to take immediate corrective actions. Also, pipelines can switch routes or configurations between normal (reduced, sampled data volume) and incident (full-fidelity data) modes to adjust data flow based on the system’s current state. By adjusting data flow, telemetry pipelines capture and process the necessary data during critical moments.
BN: What data engineering aspects can help pipelines work more efficiently?
TC: When we say data engineering, we refer to understanding data characteristics such as data quality, data governance, policy enforcement, data lineage, and data drifts. Pipelines provide data profiling capabilities, detect data drifts, and manage governance by supporting compliance and ensuring that only the right data is routed to the right teams. Various data sources and data processing components can be preconfigured according to company policies so that usage conforms to the norms and regulatory requirements. However, the skills gap may prevent organizations from taking such an approach. Therefore, these capabilities have to be built into the foundation of the pipeline and how it operates so that there is no additional burden on DevOps, engineering, or security teams to add additional resources with data engineering skills.
BN: What can be done to support compliance and manage sensitive data in logs?
TC: Compliance requires stringent data management practices within telemetry data streams. Organizations must ensure that PII is not inadvertently included in their logs and makes its way to systems and users who should not have access to the sensitive data. To protect sensitive data, they can implement redaction, masking, encryption, and decryption techniques to transform data as it moves through the pipeline. Telemetry pipelines use in-stream alerts to identify and notify teams of any data changes that allow PII to sneak into the pipeline. Automated compliance checks and data governance frameworks can further help organizations maintain adherence to these regulations, ensuring that sensitive data is consistently protected.
BN: Can good telemetry data help to achieve effective business decision making?
TC: Absolutely, good telemetry data provides insights into various aspects of operations, from performance metrics and customer experience to user behavior. For example, an e-commerce company can use telemetry pipelines to extract business insights from metrics such as product orders, cart checkouts, cart abandonments, transaction performance, and compliance risk incidents, providing valuable information for effective decision-making. A telemetry pipeline can help extract metrics from events and logs or convert certain events to metrics for easier analysis and visualization. The data is aggregated, enriched, and delivered in easily consumable formats using visualization tools like Grafana, ensuring that organizations can confidently analyze and visualize their reports. By leveraging these insights, organizations can make informed decisions that enhance operational efficiency, improve user experience, and drive business growth.
Treating telemetry data as an enterprise asset ensures that all teams have access to the data they need in the right format while meeting compliance requirements. This approach maximizes the value observability investments, leading to better business outcomes and a sustained competitive edge.
Image credit: SergeyNivens/depositphotos.com