Why strong data foundations are essential to implementing AI [Q&A]

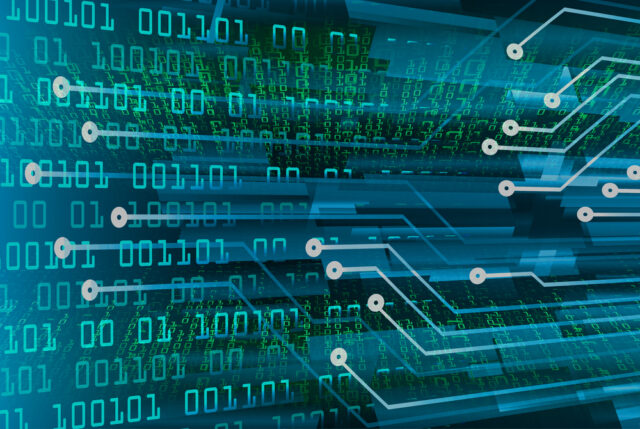
Lots of organizations are rushing to embrace AI and hoping to deliver business value. But AI is only as good as the data that underpins it.
We spoke to Julian LaNeve, chief technology officer of Astronomer, to discuss why it's important to fix the foundations before implementing AI solutions.
BN: Why are so many companies rushing to implement AI without focusing on building strong data foundations first?
JLN: There are a number of reasons for this. The main one is there's competitive pressure; organizations want to gain an edge quickly, which is leading to shortcuts in building the necessary infrastructure.
This fervor is also in part being driven by venture capital interest, as investors globally are chomping at the bit to find the next big AI opportunity.
To be clear, the motivations are all well and good. But it’s leading to misplaced priority -- companies are focusing on AI implementation without addressing the critical foundation of data quality and management. In other words, they are putting the cart before the horse.
What this also clearly emphasizes is the decades long underinvestment in data engineering, which is now coming to the forefront as companies look to scale AI projects.
Ultimately, this short-term thinking is leading companies to prioritize quick wins over sustainable solutions. And the consequences are starting to come to light. A study by RAND Corporation revealed that 80 percent of AI projects fail due to poor data infrastructure and insufficient data for training.
We're about to hear much more along these lines in the coming few years.
BN: Can you explain the key challenges organizations face when managing their data for successful AI implementation?
JLN: One of the biggest challenges is making sure data is accurate and relevant -- it's the backbone of training and augmenting reliable AI models. But even when companies get their data right, integrating it from different sources and departments can be a real headache, especially with systems that don't talk to each other easily.
Then there's the issue of data governance. It's crucial, but tricky, because organizations have to create policies that handle data access, privacy, and compliance, all while keeping everything secure.
When it comes to real-time processing, many companies simply aren't equipped with the right tools or infrastructure. This leaves them struggling with fragmented systems and a lack of good data for training AI models effectively.
The bottom line is that, as AI projects grow, the need for scalable infrastructure will become more urgent in order to manage the increasing data load.
BN: How did investing in developers and adopting DevOps transform software development, and what lessons can we take from that for data engineering?
JLN: In 2011, Andreessen Horowitz's ‘software is eating the world' helped ignite a decade-long arms race for organizations to invest heavily in software development. This led to DevOps, which boosted productivity and innovation by optimizing workflows and automating processes, creating immense ROI on the vast investments made in software engineering and infrastructure. DevOps improved development speed and product quality, establishing engineers as 'kingmakers' and software as the foundation for competitive differentiation.
But while software is obviously still necessary, it's not enough for maintaining a competitive edge. The differentiator lies in the date. In other words, we're now in a time where 'data is feeding the world.'
To illustrate my point, Companies like Netflix and Spotify disrupted industries with software, but they sustain their dominance by using data to provide unmatched personalization, insights, and user engagement. Software enables scale, but data creates stickiness and differentiation -- it's how companies with unique data assets can leverage those insights better than anyone else. As a result, companies are now redirecting attention and budgets to data engineering and infrastructure, paralleling the investment wave software experienced a decade ago.
Like software and DevOps, data teams now command large budgets, encompassing data engineering, machine learning engineering (MLE), and AI infrastructure. A similar focus on maximizing ROI has emerged, with tools and frameworks designed to optimize productivity and support innovation across data functions. In fact, recent studies indicate that even a small productivity gain can yield substantial returns: McKinsey reports a potential 30 percent productivity improvement through advanced data infrastructure and analytics automation.
The bottom line is, to stay competitive, companies must treat their data functions as they did software: by investing in tools, automation, and processes that elevate productivity and unlock the full value of data.
BN: What are the main reasons behind the current under-investment in data engineering, and how is this impacting organizations?
JLN: Data engineering has long been overlooked because many companies used to see data as just a support function -- something for internal reports, rather than a true asset for driving strategy. This outdated view led to underinvestment in data, with most of the focus going toward software development instead.
But today, data is often a company's secret sauce. Think of Netflix with its unique viewing data, Ramp with its insights on vendor spending, or OpenAI with its vast textual data -- all of which fuel products and experiences that set them apart. Tapping into these kinds of unique data advantages requires strong data engineering to turn raw information into insights that actually make a difference. Without those investments, companies risk missing out on better product experiences, smarter recommendations, and even industry leadership.
The problem is made worse by a shortage of skilled data engineers and fewer established tools, which makes building solid data teams challenging. While software engineers have often taken the spotlight, data engineers are now crucial to keeping a competitive edge, especially as AI increasingly relies on high-quality, well-managed data.
BN: How can DataOps become the new DevOps for data teams, and what steps should organizations take to build a DataOps culture?
JLN: To make DataOps as valuable for data teams as DevOps has been for software, companies need to focus on making life easier for their data teams in practical ways. First, reduce the time spent maintaining existing data products -- ideally, make the maintenance almost effortless so teams can focus on creating new value rather than just keeping the lights on.
Second, streamline the process for building new data solutions to make it as fast and easy as possible. When data teams don’t have to struggle with tedious steps or endless fixes, they’re free to innovate and get valuable insights out quickly.
Building this kind of DataOps culture means investing in flexible, scalable infrastructure that supports teamwork across data engineers, scientists, and business teams. Automation should handle the routine, like running data pipelines and checking for quality, so people can focus on higher-impact work. Data governance policies help everyone feel confident about security and compliance, and agile methods let teams adapt fast to changing needs.
Empowering data teams to grow their skills and recognizing their key role in driving AI success is essential, too. When data engineers feel valued and equipped to lead, the entire organization benefits. Ultimately, if companies create a culture where data teams can work collaboratively and productively, DataOps can become as crucial to growth and innovation as DevOps has been for software development.
Image credit: [email protected]/depositphotos.com